Learning Discriminative Features via Multi-Hierarchical Mutual Information for Unsupervised Point Cloud Registration
IEEE Transactions on Circuits and Systems for Video Technology(2024)
摘要
Extracting discriminative representations is the key step for correspondence-free point cloud registration. The extracted representations require to be discriminative to transformation, which demands representations to reduce the influence of redundant information irrelevant to transformation. However, recently proposed methods ignore this crucial property, resulting in limited ability to represent point cloud. In addition, researching correspondence-free point cloud registration has stagnated in recent years. In this paper, we try to relieve features redundancy issue for correspondence-free point cloud registration from a new perspective. Specifically, our method comprises two stages: feature extraction stage and rigid body transformation stage. In feature extraction stage, we aim to maximize multi-hierarchical mutual information between different hierarchical features, which can provide discriminative and less redundancy representations to regress transformation parameters for next stage. In rigid body transformation stage, we utilize dual quaternion to estimate transformation parameters, which combines rotation and translation simultaneously within a unified framework and obtains a compact representations for rigid transformation. The proposed model is trained in an unsupervised manner on the ModelNet40 dataset. The experimental results illustrate that our method achieves higher accuracy and robustness compared with existing correspondence-free methods.
更多查看译文
关键词
Point cloud registration,multi-hierarchical mutual information,discriminative representations,dual quaternion
AI 理解论文
溯源树
样例
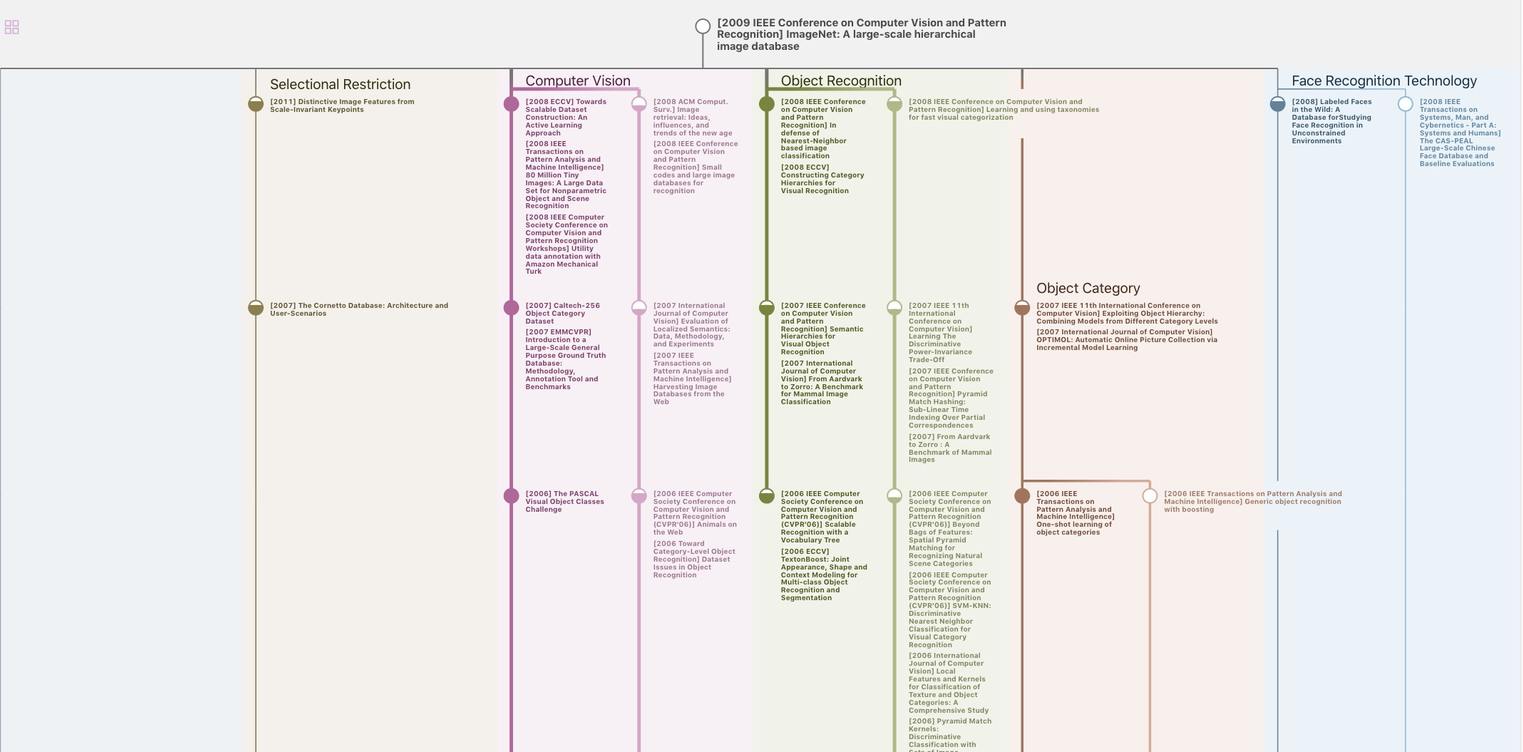
生成溯源树,研究论文发展脉络
Chat Paper
正在生成论文摘要