Distributed Learning based on 1-Bit Gradient Coding in the Presence of Stragglers
IEEE Transactions on Communications(2024)
摘要
This paper considers the problem of distributed learning (DL) in the presence
of stragglers. For this problem, DL methods based on gradient coding have been
widely investigated, which redundantly distribute the training data to the
workers to guarantee convergence when some workers are stragglers. However,
these methods require the workers to transmit real-valued vectors during the
process of learning, which induces very high communication burden. To overcome
this drawback, we propose a novel DL method based on 1-bit gradient coding
(1-bit GCDL), where 1-bit data encoded from the locally computed gradients are
transmitted by the workers to reduce the communication overhead. We
theoretically provide the convergence guarantees of the proposed method for
both the convex loss functions and nonconvex loss functions. It is shown
empirically that 1-bit GC-DL outperforms the baseline methods, which attains
better learning performance under the same communication overhead.
更多查看译文
关键词
Distributed learning,1-bit quantization,stragglers,communication overhead,convergence analysis
AI 理解论文
溯源树
样例
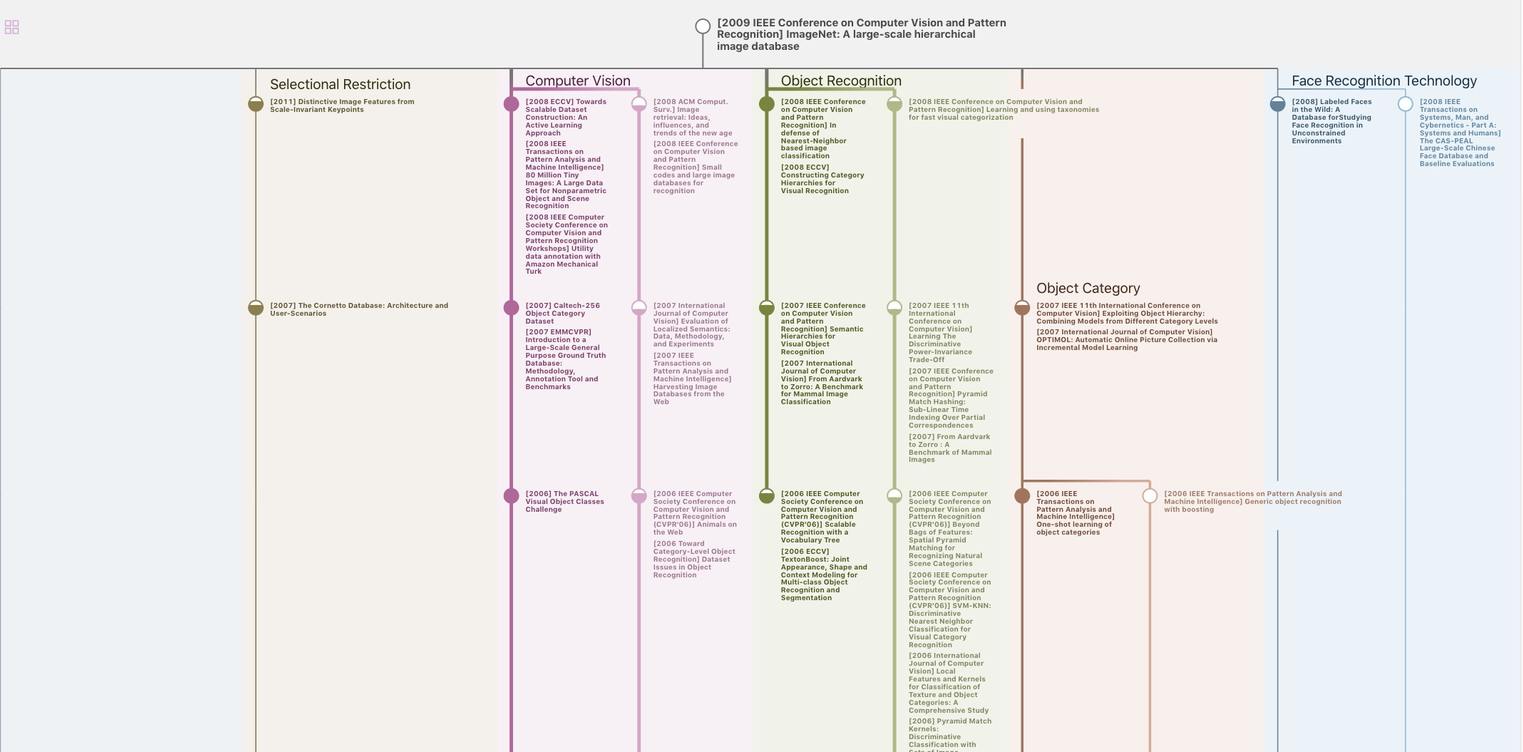
生成溯源树,研究论文发展脉络
Chat Paper
正在生成论文摘要