Drone Identification Based on Normalized Cyclic Prefix Correlation Spectrum
IEEE Transactions on Cognitive Communications and Networking(2024)
摘要
Utilizing deep learning (DL) to identify drones through radio signals has been proven to be a promising approach. However, two significant challenges remain to be solved. The first is how to identify drones effectively at the low signal-to-noise ratio (SNR) regime, and the second is how to identify drones stably among numerous unknown interferences. In theory, sufficient data can alleviate the above problems, but the costs of signal acquisition and labeling are usually unacceptable. In this work, we aim to improve the robustness of feature representation by introducing stable prior knowledge of drone signals to alleviate the two problems. Since drones commonly adopt orthogonal frequency division multiplexing (OFDM) modulation with particular cyclic prefix (CP) structures for video transmission, we propose a drone identification algorithm using a convolutional neural network (CNN) and normalized CP correlation spectrum (NCPCS). The NCPCS is strongly correlated with two invariant parameters, i.e., OFDM symbol duration and CP duration, and is mutually exclusive with other signals. Thus, NCPCS natively improves the robustness of the drone identification system to unknown interference signals. Besides, to keep the characteristic of NCPCS clear at the low SNR regime, we calculate the improved NCPCS by accumulating multiple consecutive OFDM symbols. The increase in correlation length effectively sharpens the peaks at low SNRs. Finally, a suitable CNN and a data augmentation (DA) method for NCPCS are proposed to precisely and generically extract these characteristics from NCPCS to identify drones. In this work, a universal software radio peripheral (USRP) X310 is utilized to collect the radio signals of five drones to construct the experimental dataset. We test the proposed algorithm under two different conditions: Gaussian noise condition and co-frequency interference condition. Experimental results show that the proposed algorithm outperforms waveform-based, spectrum-based and spectrogram-based algorithms under the two conditons. Besides, the proposed algorithm remains good robustness to unknown interference signals.
更多查看译文
关键词
Drone identification,radio signal,OFDM,cyclic prefix correlation spectrum
AI 理解论文
溯源树
样例
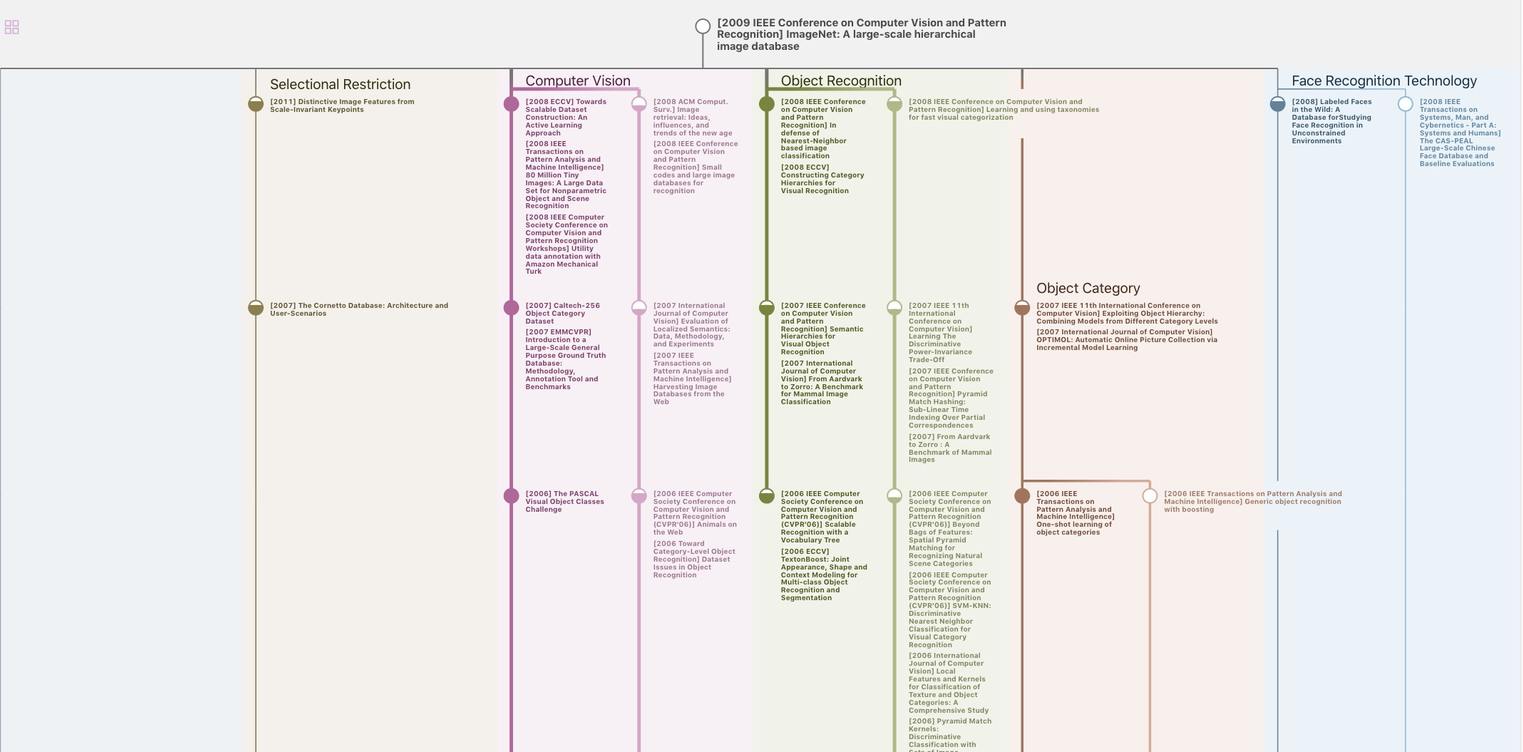
生成溯源树,研究论文发展脉络
Chat Paper
正在生成论文摘要