Neural-Network-Based Free-Boundary Equilibrium Solver to Enable Fast Scenario Simulations
IEEE TRANSACTIONS ON PLASMA SCIENCE(2024)
摘要
A numerical free-boundary equilibrium (FBE) solver, based on finite-difference and Picard-iteration methods, has been recently developed on a rectangular grid to compute the poloidal-flux distribution in tokamaks. An accelerated version of this computationally intensive numerical solver, named FBE-Net, has been developed in this work by leveraging the physics-informed neural network (PINN) method. Within this framework, the neural-network (NN) component employs a fully connected multilayer perceptron (MLP) architecture. Critically, the underlying physical constraints are defined by the Grad-Shafranov (G-S) equation, ensuring the NN-based solver adheres to essential governing principles. FBE-net is trained on a dataset generated by the numerical solver, which serves as a source of ground truth. The inputs for FBE-Net are the plasma current, the normalized beta, and the coil currents, while the outputs are the poloidal-flux map and a set of flux-averaged equilibrium parameters. When compared to the numerical solver, the NN-based solver displays a significant increase in computational efficiency without notably sacrificing accuracy.
更多查看译文
关键词
Plasmas,Mathematical models,Artificial neural networks,Training,Coils,Tokamaks,Toroidal magnetic fields,Free-boundary equilibrium (FBE) solver,Grad-Shafranov (G-S) equation,physics-informed neural network (PINN)
AI 理解论文
溯源树
样例
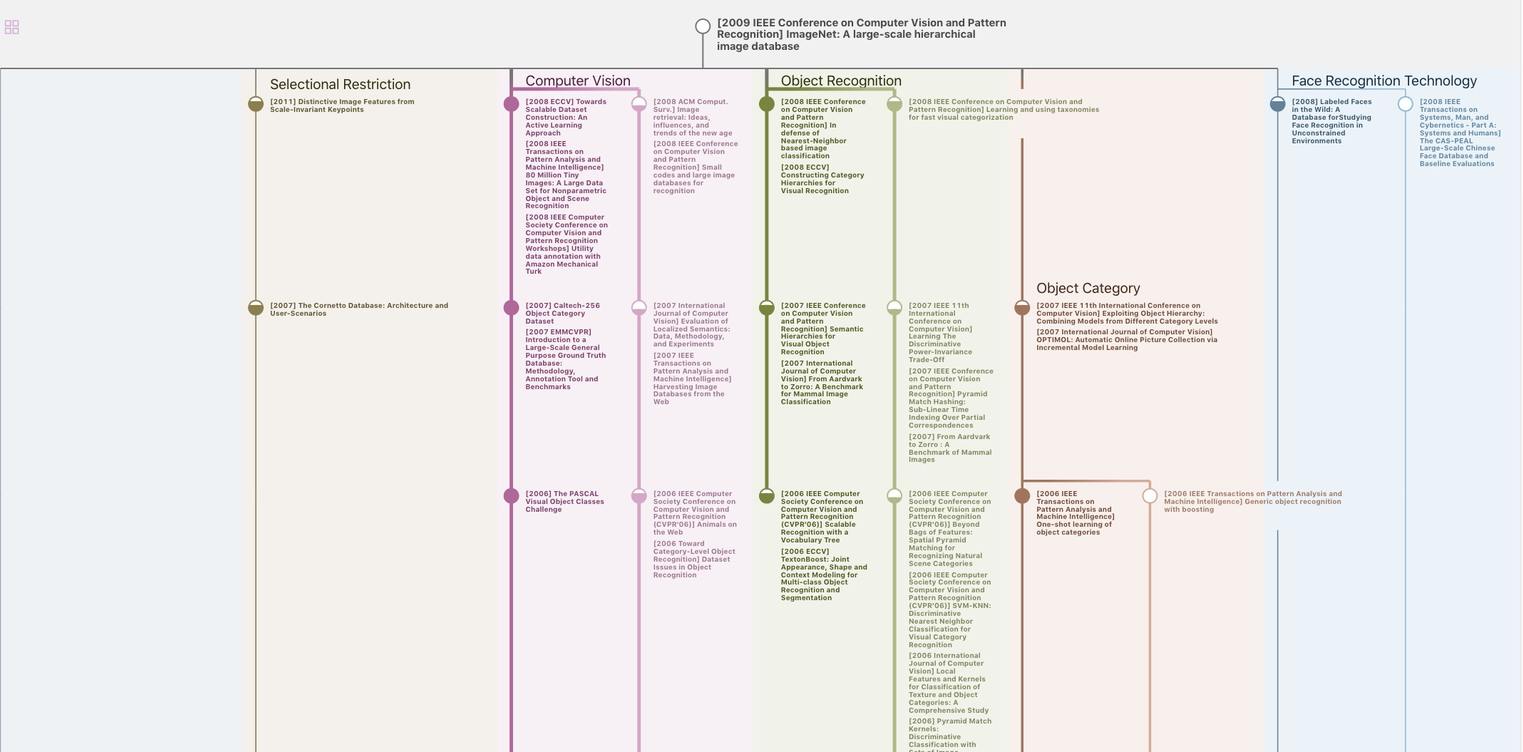
生成溯源树,研究论文发展脉络
Chat Paper
正在生成论文摘要