Flood-ResNet50: Optimized Deep Learning Model for Efficient Flood Detection on Edge Device.
International Conference on Machine Learning and Applications(2023)
摘要
Floods are highly destructive natural disasters that result in significant economic losses and endanger human and wildlife lives. Efficiently monitoring Flooded areas through the utilization of deep learning models can contribute to mitigating these risks. This study focuses on the deployment of deep learning models specifically designed for classifying flooded and non-flooded in UAV images. In consideration of computational costs, we propose modified version of ResNet50 called Flood-ResNet50. By incorporating additional layers and leveraging transfer learning techniques, Flood-ResNet50 achieves comparable performance to larger models like VGG16/19, AlexNet, DenseNet161, EfficientNetB7, Swin(small), and vision transformer. Experimental results demonstrate that the proposed modification of ResNet50, incorporating additional layers, achieves a classification accuracy of 96.43%, F1 score of 86.36%, Recall of 81.11%, Precision of 92.41 %, model size 98MB and FLOPs 4.3 billions for the FloodNet dataset. When deployed on edge devices such as the Jetson Nano, our model demonstrates faster inference speed (820 ms), higher throughput (39.02 fps), and lower average power consumption (6.9 W) compared to larger ResNet101 and ResNet152 models.
更多查看译文
关键词
ResNet50,Edge device,Vision transformer,VGG,AlexNet,Jetson nano
AI 理解论文
溯源树
样例
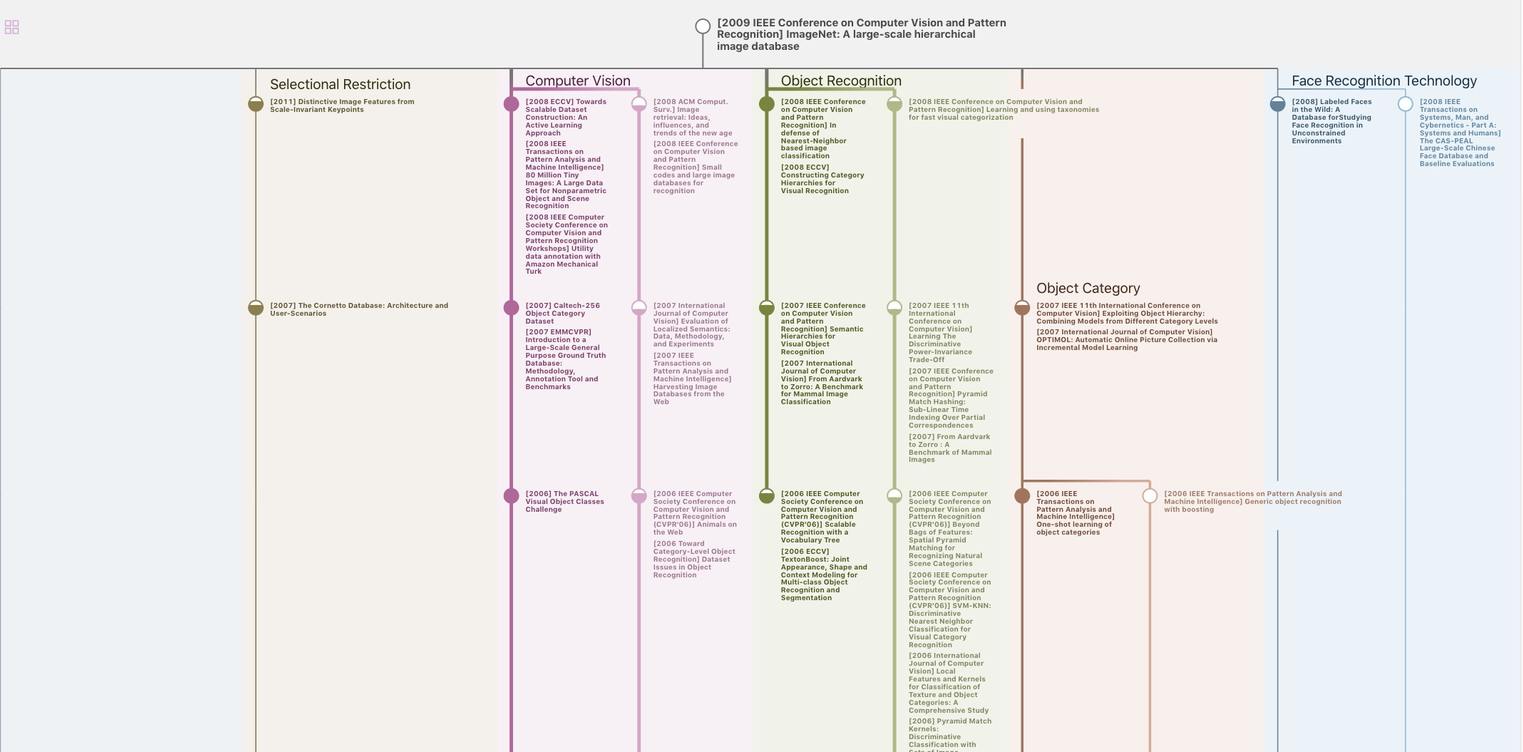
生成溯源树,研究论文发展脉络
Chat Paper
正在生成论文摘要