Transferable Adversarial Attacks on Transformer and CNN
2023 China Automation Congress (CAC)(2023)
摘要
Vision Transformers (ViTs) are attention-based encoder-decoder architectures widely used in computer vision (CV) and have shown excellent performance. However, ViTs are also vulnerable to adversarial attacks. In actuality, the adversarial samples produced by various models exhibit some degree of overfitting. The primary challenge with anti-sample crossmodel transfer is based on this. In this research, a novel approach to enhance the transferability of adversarial samples between various ViTs and between ViTs and CNNs is proposed. The main steps of this method include: dividing the original image (224*224) into patches (16*16), extracting high-frequency information and calculating interaction values, and then applying momentum attacks to reduce the interaction between patches. (The negative correlation between game interaction among adversarial perturbation units and adversarial transferability has been verified in the work of Xin Wang et al. [1].) According to experimental findings, the suggested technique may significantly enhance the transferability of ViTs and between ViTs and CNNs.
更多查看译文
AI 理解论文
溯源树
样例
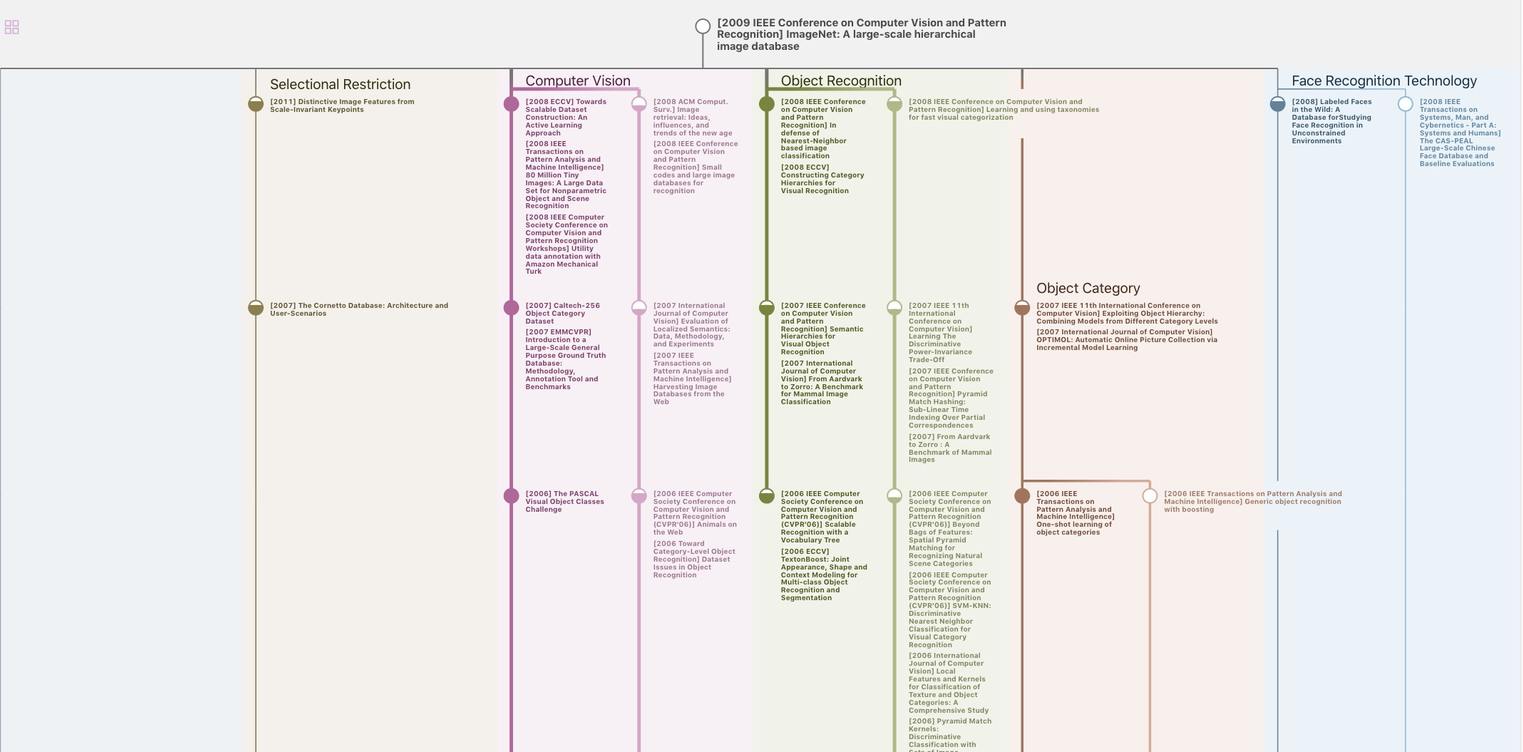
生成溯源树,研究论文发展脉络
Chat Paper
正在生成论文摘要