Unsupervised Domain Adaptation via Adversarial Attack Consistency
2023 China Automation Congress (CAC)(2023)
摘要
Many unsupervised domain adaptation methods leverage pseudo-labels to facilitate model training in the target domain. However, due to the domain gap, these pseudo-labels often exhibit a certain level of noise, leading to a dispersion of intra-class features within the target domain. Unlike previous self-training methods that use random perturbations for consistency regularization, we propose an adversarial attack consistency framework for unsupervised domain adaptation, which treats the adversarial example as a different version of the original sample in the target domain and aligns the predictions between them. The algorithm effectively facilitates the network's ability to learn class-wise semantic information and a more compact feature space in the target domain. The experimental results show that the proposed algorithm has achieved significant performance over state-of-the-art methods.
更多查看译文
AI 理解论文
溯源树
样例
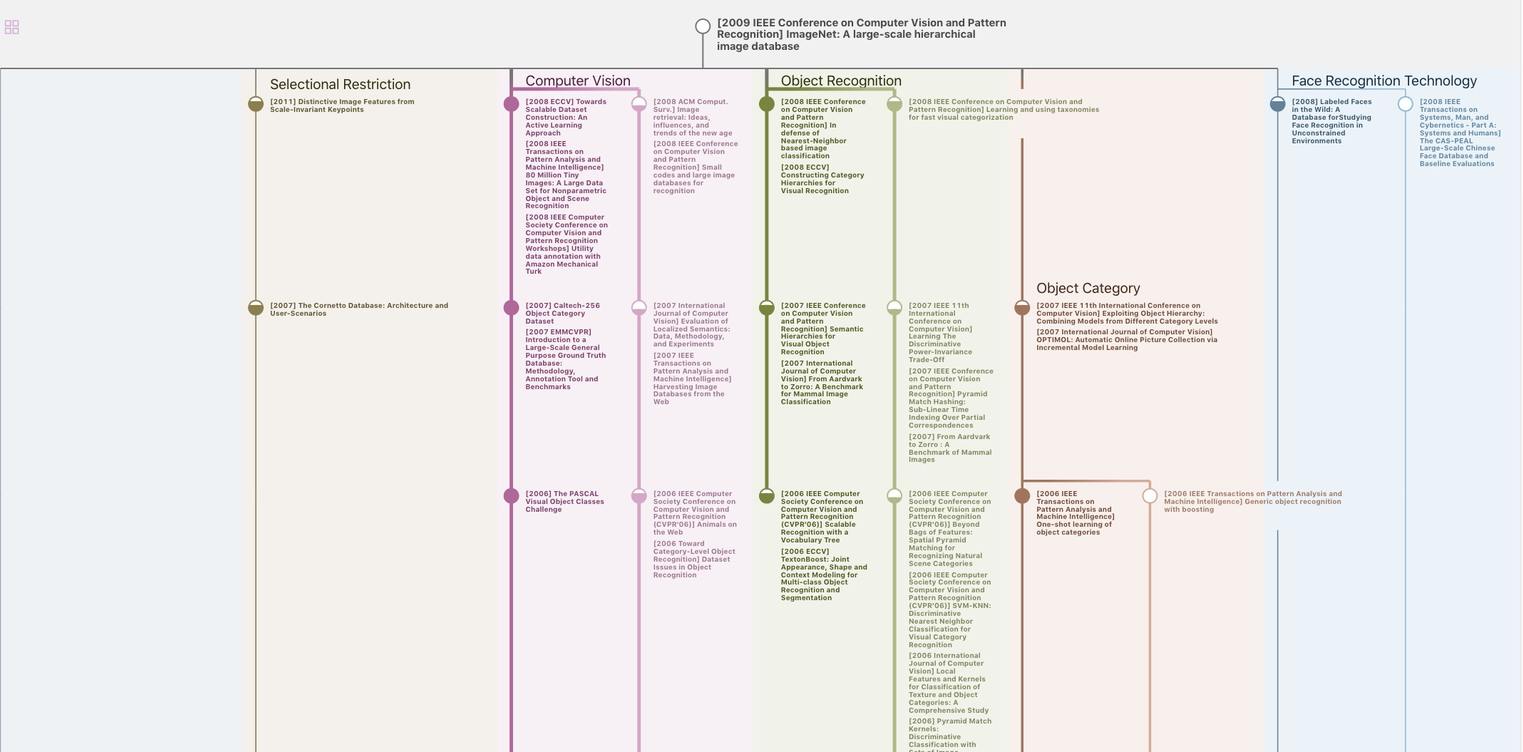
生成溯源树,研究论文发展脉络
Chat Paper
正在生成论文摘要