Path Planning Based on Deep Reinforcement Learning and Perturbed Fluids
2023 China Automation Congress (CAC)(2023)
摘要
Path planning is one of the fundamental components in autonomous navigation. Most existing works assume static and fixed environments. However, path planning is widely applied in random and dynamic environments, such as search and rescue, surveillance, and other scenarios. In this paper, we propose a method that combines deep reinforcement learning with dynamic disturbance fluid systems, enabling unmanned aerial vehicles (UAVs) to perform navigation tasks in environments with randomness and dynamics. The method is based on the disturbance fluid algorithm and the twin-delayed deep deterministic policy gradient (TD3) algorithm. Simulation results demonstrate that this method enables UAVs to safely accomplish autonomous navigation tasks in multi-obstacle environments, showcasing its effectiveness. Moreover, compared to Deep Deterministic Policy Gradient (DDPG), this method exhibits superior generalization capabilities.
更多查看译文
AI 理解论文
溯源树
样例
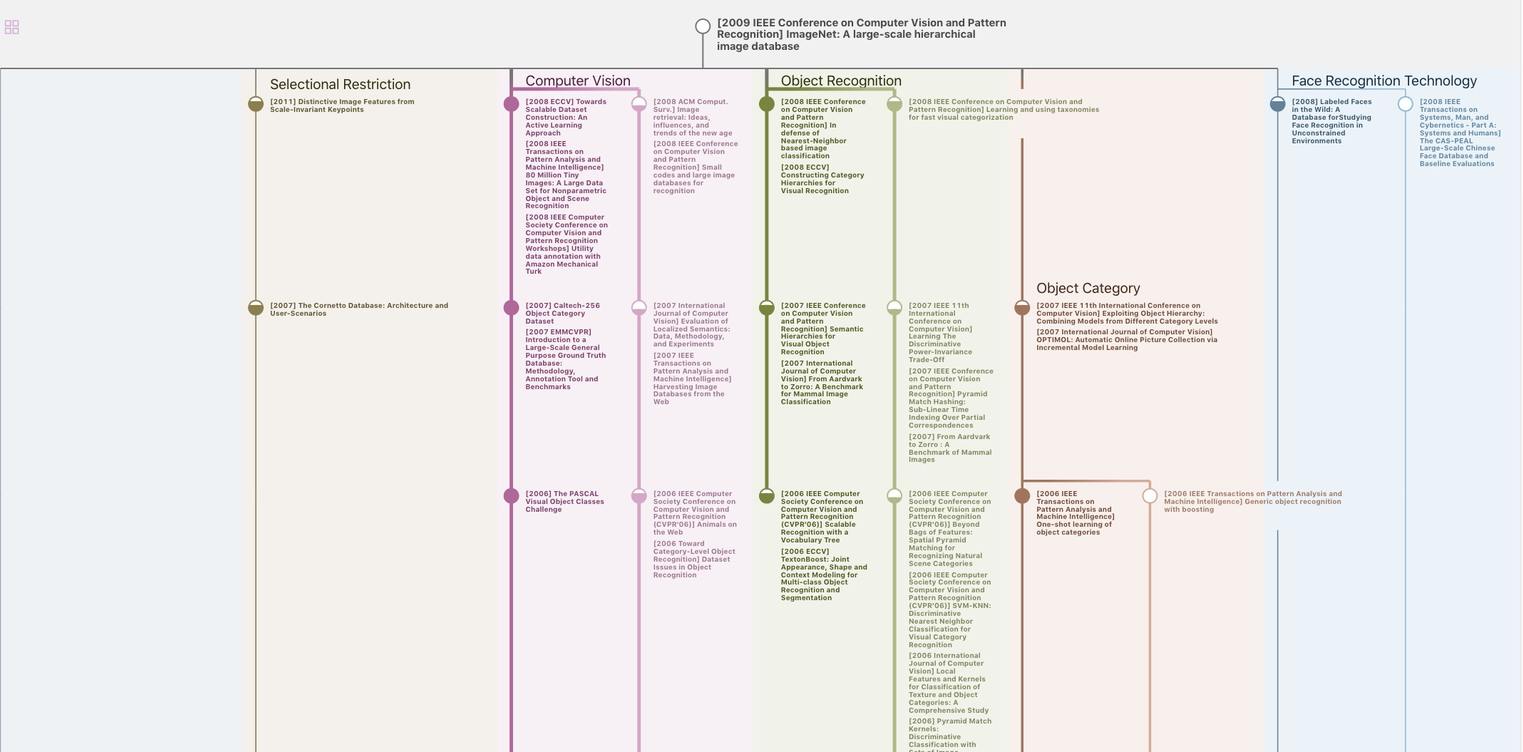
生成溯源树,研究论文发展脉络
Chat Paper
正在生成论文摘要