Road Segmentation for Remote Sensing Images Based on Global Feature Attention ResUnet
2023 China Automation Congress (CAC)(2023)
摘要
In current road segmentation methods for remote sensing images, high-level semantic features (such as roads and shadows) are often lost in the decoding process, resulting in a decrease in the accuracy of road segmentation. To solve this problem, we strengthened the correlation between shallow and deep features in a typical U-Net framework using two global attention mechanisms (channel and spatial) as follows. We first extract the features of the image in ResUnet's decoder and calculate channel attention on these features. we then map all features to shallow features and define spatial attention, which enhances the representation of deep spatial semantic information on the shallow feature. The feature maps processed by the global channel attention and global spatial attention are then combined with the ResUnet decoder for feature fusion and up-sampling, finally producing the road segmentation result. Experimental results show that our model effectively resolves the discontinuity problem in road segmentation and significantly improves the accuracy of road extraction from remote sensing images.
更多查看译文
AI 理解论文
溯源树
样例
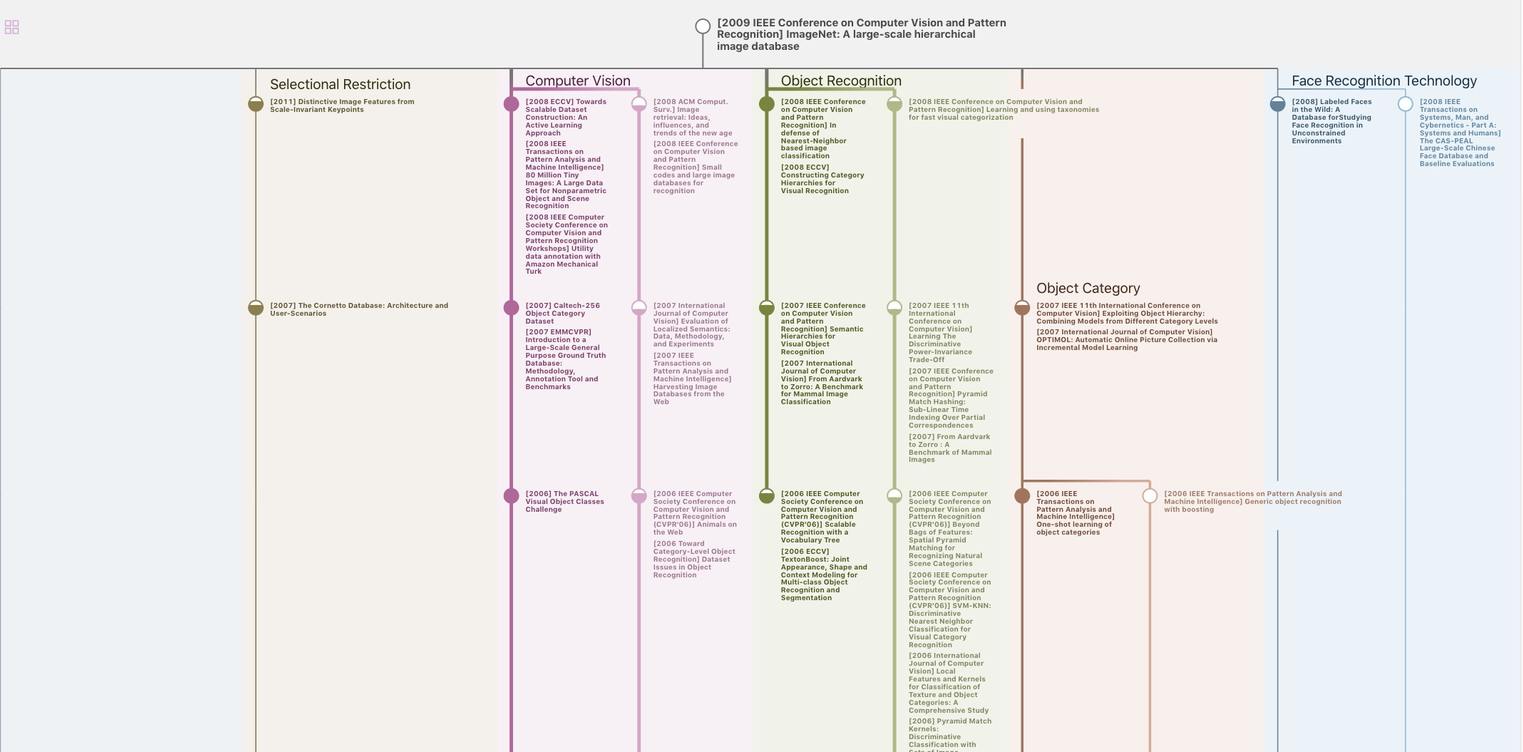
生成溯源树,研究论文发展脉络
Chat Paper
正在生成论文摘要