Chronic Obstructive Pulmonary Disease in the United States: A Comparison of Multiple Linear Regression and Machine Learning Models (Preprint)
crossref(2024)
摘要
BACKGROUND Lung disease is a severe problem in the United States. Despite the decreasing rates of cigarette smoking, COPD continues to be health burden in the United States. In this paper, we focus on Chronic Obstructive Pulmonary Disease in the United States from 2016 to 2019. OBJECTIVE We gather a diverse set of data sources to better understand and predict COPD rates at the level of Core-Based Statistical Area in the United States. The objective is to compare linear models with machine learning models to obtain the most accurate and interpretable model of COPD. METHODS We integrate data from multiple Centers for Disease Control sources and use them to analyze Chronic Obstructive Pulmonary Disease by using different types of methods. We include cigarette smoking, a well-known contributing factor, and race / ethnicity variables because health disparities among different races and ethnicities in the United States are also well-known. The models also include air quality index, education, employment, and economic variables. We fit models with both multiple linear regression and machine learning methods. RESULTS The most accurate multiple linear regression model has variance explained = 81.1% and Root Mean Squared Error = 0.73. The most accurate machine learning model has variance explained = 87.1% and Root Mean Squared Error = 0.53. Overall, cigarette smoking and household income are the strongest predictor variables. Hispanic percentage of CBSA, Education, and American Indian / Alaska Native percentage of CBSA are moderately strong predictors. CONCLUSIONS This research highlights the importance of using diverse data sources as well as multiple methods to understand and predict COPD. The most accurate model is a Support Vector Machine, which captured non-linearities in a model whose accuracy is superior to the best multiple linear regression. Our interpretable models suggest ways that individual predictor variables can be used in interventions aimed at decreasing COPD rates. Gaps in understanding the health impacts of air pollution, particularly in relation to climate change, suggest a need for further research to design interventions and improve public health.
更多查看译文
AI 理解论文
溯源树
样例
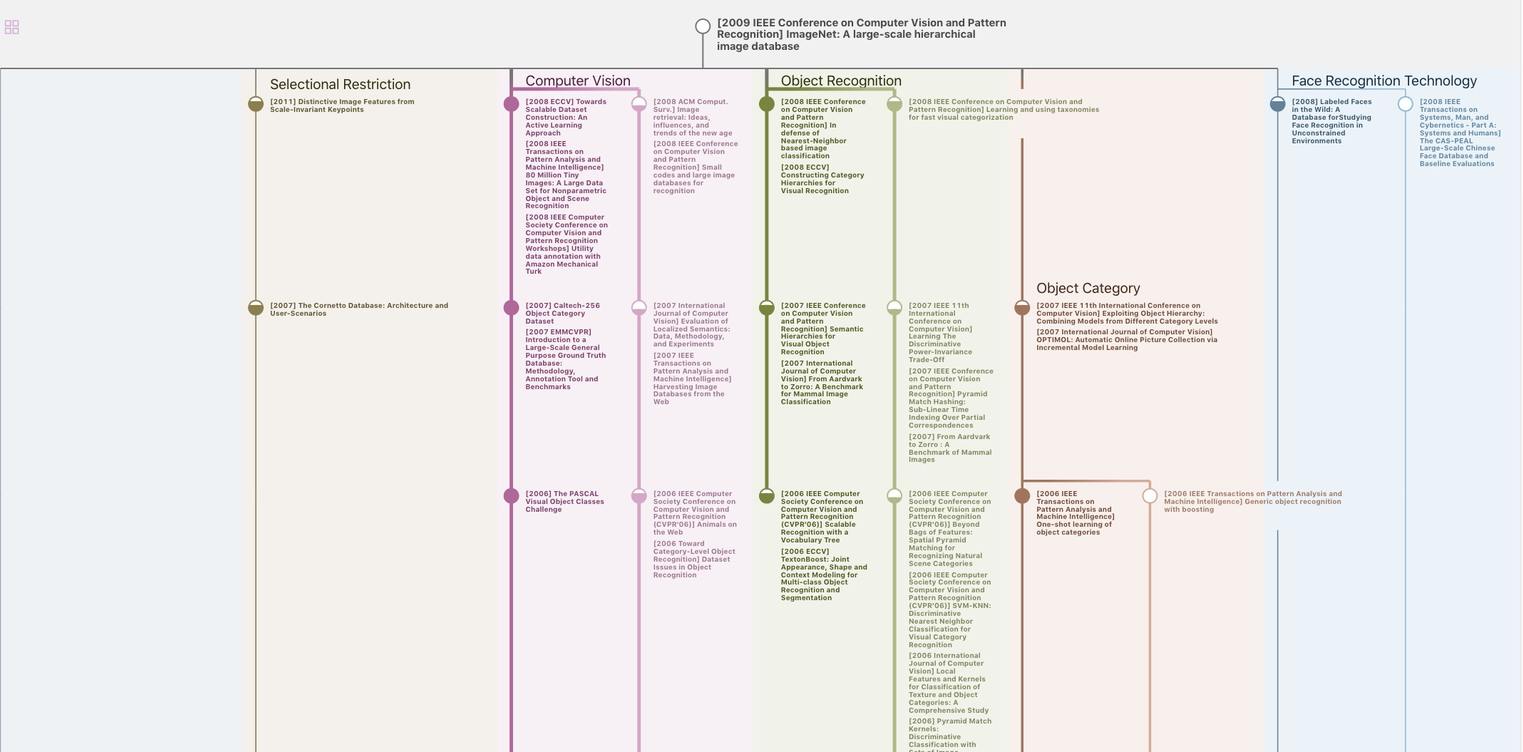
生成溯源树,研究论文发展脉络
Chat Paper
正在生成论文摘要