EEG Signal Processing Based on Incremental Sparse Nonnegative Matrix
2023 China Automation Congress (CAC)(2023)
摘要
This paper proposes a novel algorithm for the classification identification of epileptic electroencephalogram (EEG) signals. The method utilizes an incremental sparse nonnegative matrix and feature selection to improve the accuracy of classification between seizure and nonseizure signals. The proposed algorithm, based on a sparse incremental non-negative matrix (INMFSC), models the sparse characteristics of EEG signals and enhances online learning efficiency. Simulation experiments demonstrate that INMFSC achieves faster processing speed while improving classification recognition accuracy for epileptic EEG signals. Furthermore, INMFSC exhibits superior performance in distinguishing seizure and nonseizure phases of epileptic patients. This method provides a benchmark for further investigation development of algorithms for the analysis and classification of epileptic EEG signals.
更多查看译文
关键词
Nonnegative matrix,sparse constraint,incremental learning,classification identification,epilepsy EEG
AI 理解论文
溯源树
样例
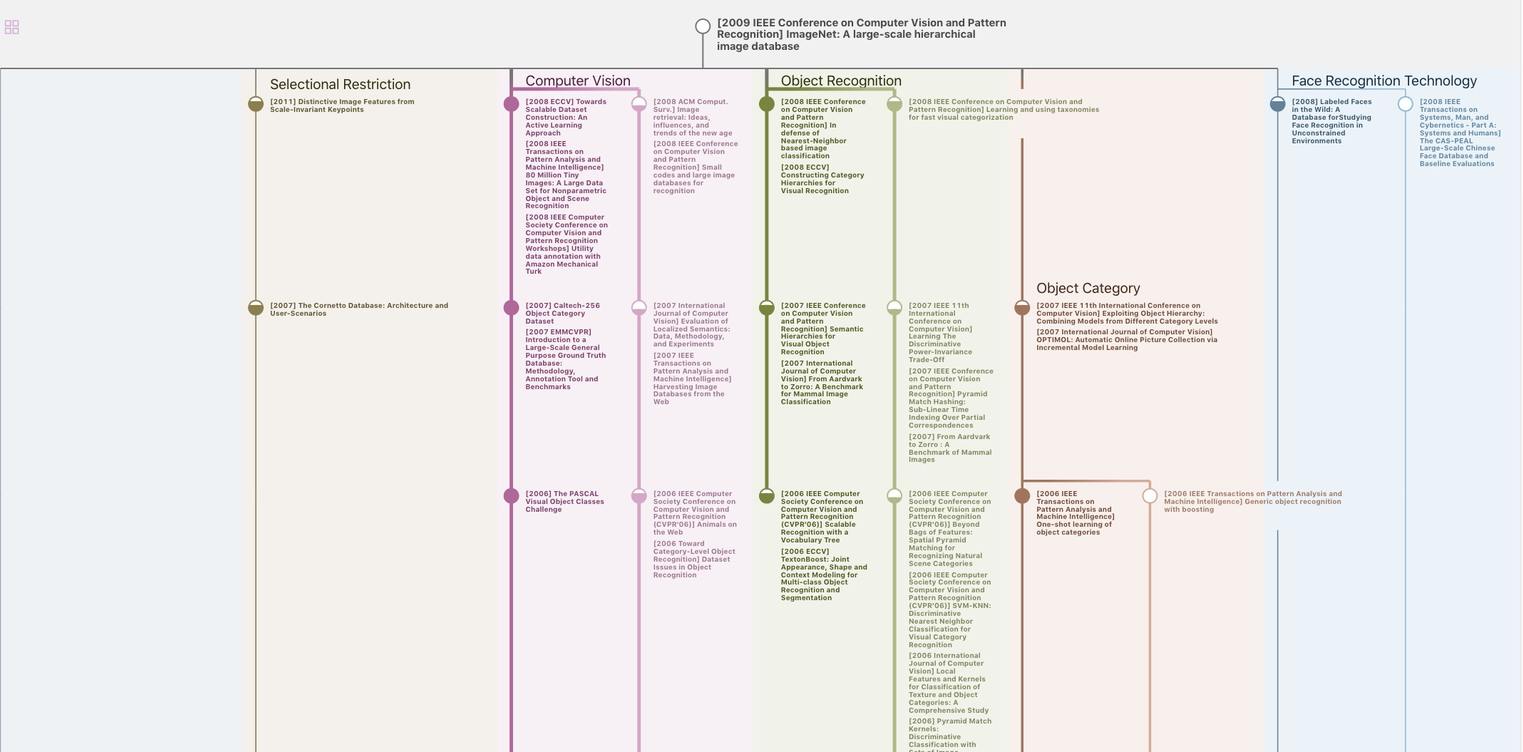
生成溯源树,研究论文发展脉络
Chat Paper
正在生成论文摘要