Clean and accurate soil quality monitoring in mining areas under environmental rehabilitation in the Eastern Brazilian Amazon
Environmental Monitoring and Assessment(2024)
摘要
Soil quality monitoring in mining rehabilitation areas is a crucial step to validate the effectiveness of the adopted recovery strategy, especially in critical areas for environmental conservation, such as the Brazilian Amazon. The use of portable X-ray fluorescence (pXRF) spectrometry allows a rapid quantification of several soil chemical elements, with low cost and without residue generation, being an alternative for clean and accurate environmental monitoring. Thus, this work aimed to assess soil quality in mining areas with different stages of environmental rehabilitation based on predictions of soil fertility properties through pXRF along with four machine learning algorithms (projection pursuit regression, PPR; support vector machine, SVM; cubist regression, CR; and random forest, RF) in the Eastern Brazilian Amazon. Sandstone and iron mines in different chronological stages of rehabilitation (initial, intermediate, and advanced) were evaluated, in addition to non-rehabilitated and native forest areas. A total of 81 soil samples (26 from sandstone mine and 55 from iron mine) were analyzed by both traditional wet-chemistry methods and pXRF. The available/exchangeable contents of K, Ca, B, Fe, and Al, in addition to H+Al, cation exchange capacity at pH = 7, Al saturation, soil organic matter, pH, sum of bases, base saturation, clay, and sand were accurately predicted (R2 > 0.70) using pXRF data, with emphasis on the prediction of Fe (R2 = 0.93), clay content (R2 = 0.81), H+Al (R2 = 0.81), and K+ (R2 = 0.85). The best predictive models were developed by RF and CR (86
更多查看译文
关键词
Proximal sensing,Machine learning,Environmental monitoring,Soil health
AI 理解论文
溯源树
样例
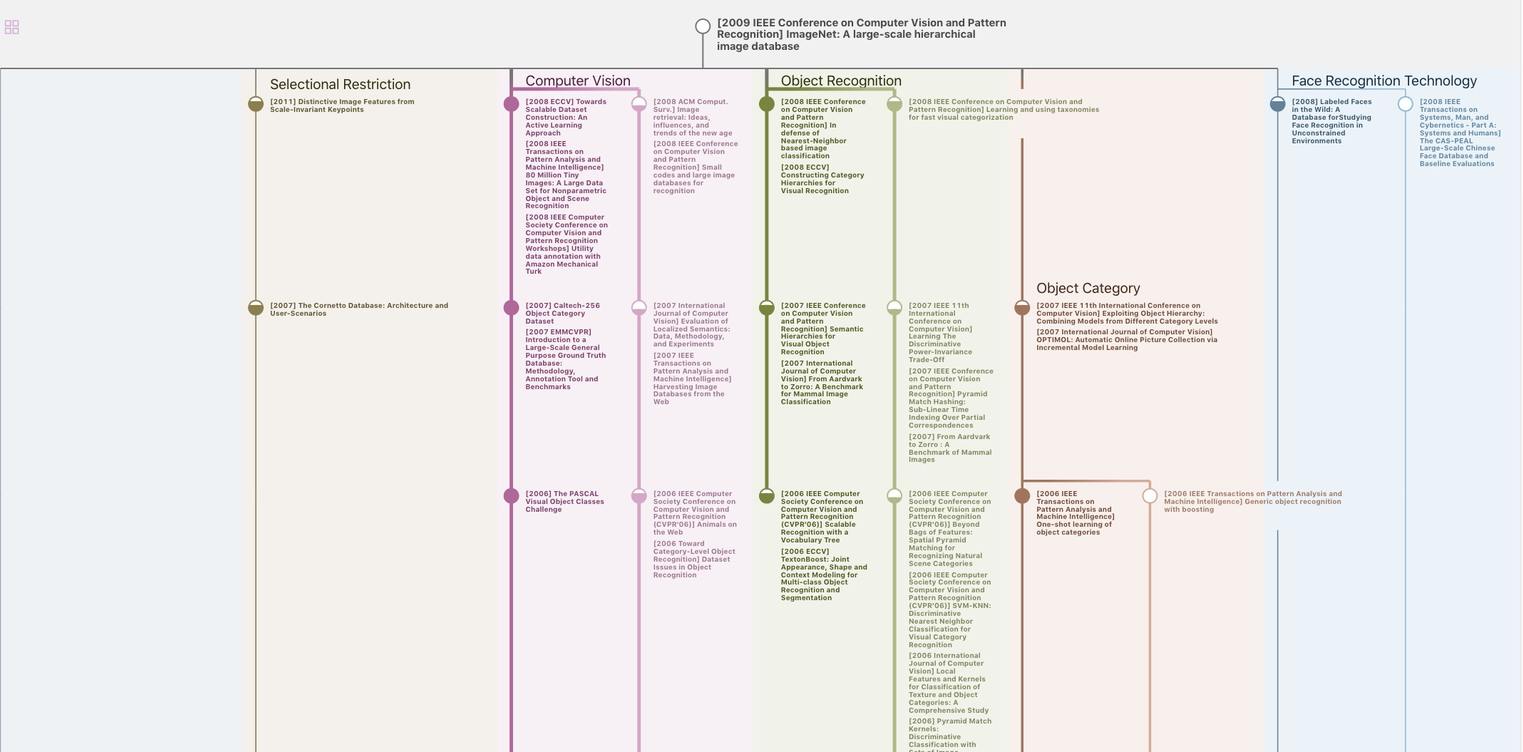
生成溯源树,研究论文发展脉络
Chat Paper
正在生成论文摘要