Trustworthiness of Pretrained Transformers for Lung Cancer Segmentation
CoRR(2024)
摘要
We assessed the trustworthiness of two self-supervision pretrained
transformer models, Swin UNETR and SMIT, for fine-tuned lung (LC) tumor
segmentation using 670 CT and MRI scans. We measured segmentation accuracy on
two public 3D-CT datasets, robustness on CT scans of patients with COVID-19, CT
scans of patients with ovarian cancer and T2-weighted MRI of men with prostate
cancer, and zero-shot generalization of LC for T2-weighted MRIs. Both models
demonstrated high accuracy on in-distribution data (Dice 0.80 for SMIT and 0.78
for Swin UNETR). SMIT showed similar near-out-of-distribution performance on CT
scans (AUROC 89.85
far-out-of-distribution accuracy on CT (AUROC 97.2
vs. 73.8
0.78 vs. 0.69). We expect these findings to guide the safe development and
deployment of current and future pretrained models in routine clinical use.
更多查看译文
AI 理解论文
溯源树
样例
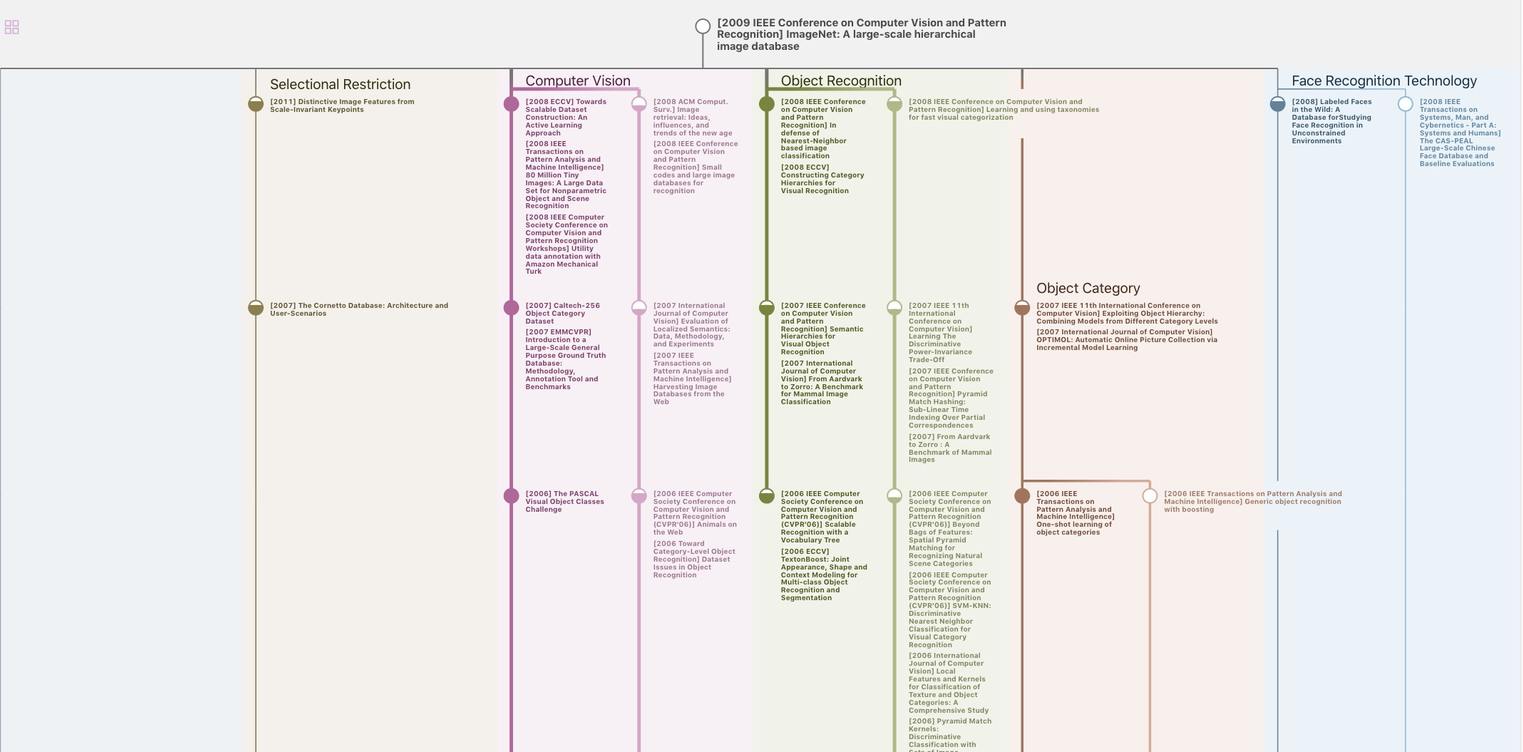
生成溯源树,研究论文发展脉络
Chat Paper
正在生成论文摘要