A Canary in the AI Coal Mine: American Jews May Be Disproportionately Harmed by Intellectual Property Dispossession in Large Language Model Training
Computing Research Repository (CoRR)(2024)
Faculty of Computing & Data Sciences | Northwestern University | School of Computing Science
Abstract
Systemic property dispossession from minority groups has often been carriedout in the name of technological progress. In this paper, we identify evidencethat the current paradigm of large language models (LLMs) likely continues thislong history. Examining common LLM training datasets, we find that adisproportionate amount of content authored by Jewish Americans is used fortraining without their consent. The degree of over-representation ranges fromaround 2x to around 6.5x. Given that LLMs may substitute for the paid labor ofthose who produced their training data, they have the potential to cause evenmore substantial and disproportionate economic harm to Jewish Americans in thecoming years. This paper focuses on Jewish Americans as a case study, but it isprobable that other minority communities (e.g., Asian Americans, HinduAmericans) may be similarly affected and, most importantly, the results shouldlikely be interpreted as a "canary in the coal mine" that highlights deepstructural concerns about the current LLM paradigm whose harms could soonaffect nearly everyone. We discuss the implications of these results for thepolicymakers thinking about how to regulate LLMs as well as for those in the AIfield who are working to advance LLMs. Our findings stress the importance ofworking together towards alternative LLM paradigms that avoid both disparateimpacts and widespread societal harms.
MoreTranslated text
Key words
large language models,economic impacts,dataset documentation
PDF
View via Publisher
AI Read Science
AI Summary
AI Summary is the key point extracted automatically understanding the full text of the paper, including the background, methods, results, conclusions, icons and other key content, so that you can get the outline of the paper at a glance.
Example
Background
Key content
Introduction
Methods
Results
Related work
Fund
Key content
- Pretraining has recently greatly promoted the development of natural language processing (NLP)
- We show that M6 outperforms the baselines in multimodal downstream tasks, and the large M6 with 10 parameters can reach a better performance
- We propose a method called M6 that is able to process information of multiple modalities and perform both single-modal and cross-modal understanding and generation
- The model is scaled to large model with 10 billion parameters with sophisticated deployment, and the 10 -parameter M6-large is the largest pretrained model in Chinese
- Experimental results show that our proposed M6 outperforms the baseline in a number of downstream tasks concerning both single modality and multiple modalities We will continue the pretraining of extremely large models by increasing data to explore the limit of its performance
Try using models to generate summary,it takes about 60s
Must-Reading Tree
Example
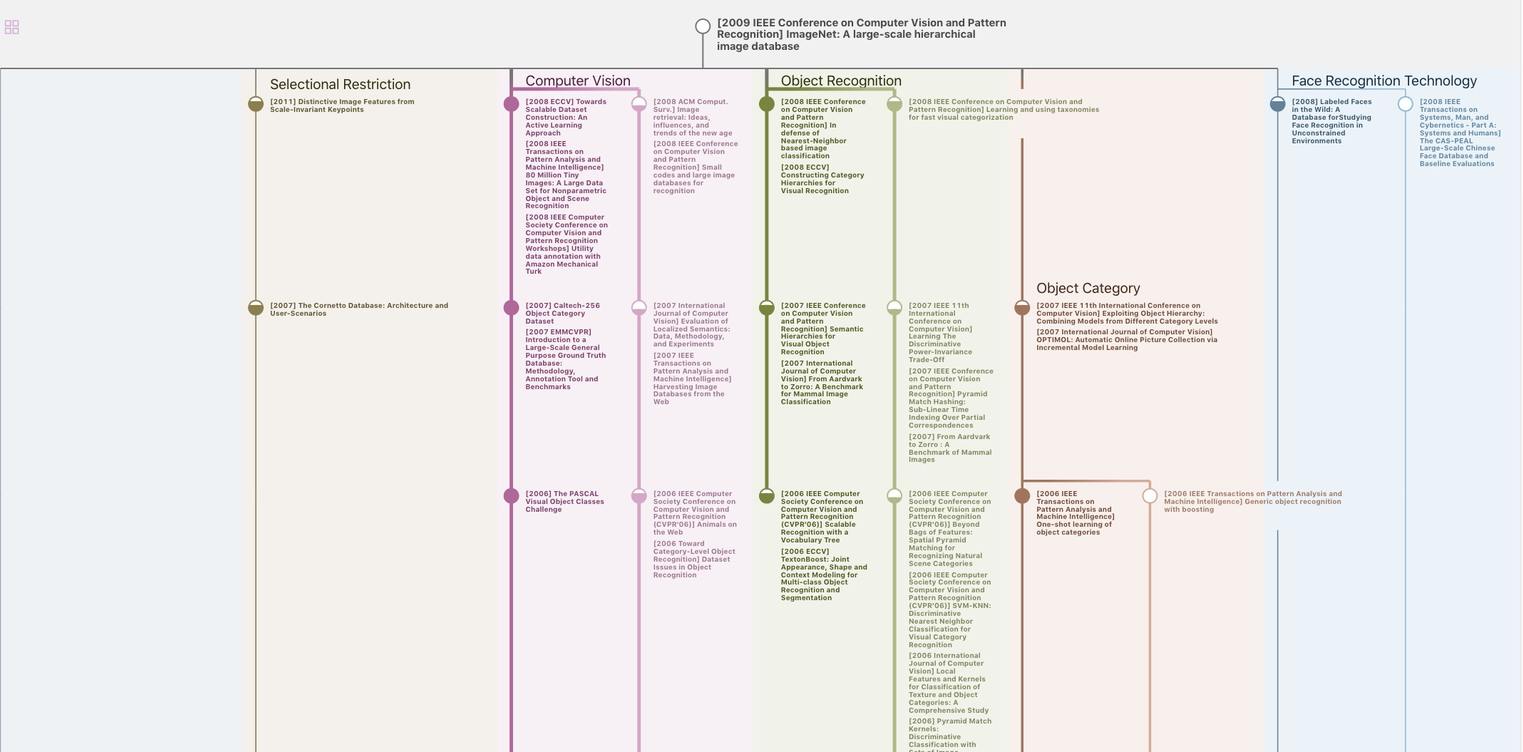
Generate MRT to find the research sequence of this paper
Related Papers
1998
被引用57 | 浏览
2019
被引用45 | 浏览
2021
被引用117 | 浏览
Trend Towards Virtual and Hybrid Conferences May Be an Effective Climate Change Mitigation Strategy.
2021
被引用70 | 浏览
2022
被引用12 | 浏览
2023
被引用865 | 浏览
2023
被引用31 | 浏览
Data Disclaimer
The page data are from open Internet sources, cooperative publishers and automatic analysis results through AI technology. We do not make any commitments and guarantees for the validity, accuracy, correctness, reliability, completeness and timeliness of the page data. If you have any questions, please contact us by email: report@aminer.cn
Chat Paper
GPU is busy, summary generation fails
Rerequest