Encode Once and Decode in Parallel: Efficient Transformer Decoding
arxiv(2024)
摘要
Transformer-based NLP models are powerful but have high computational costs
that limit deployment scenarios. Finetuned encoder-decoder models are popular
in specialized domains and can outperform larger more generalized decoder-only
models, such as GPT-4. We introduce a new configuration for encoder-decoder
models that improves efficiency on structured output and question-answering
tasks where multiple outputs are required of a single input. Our method,
prompt-in-decoder (PiD), encodes the input once and decodes output in parallel,
boosting both training and inference efficiency by avoiding duplicate input
encoding, thereby reducing the decoder's memory footprint. We achieve
computation reduction that roughly scales with the number of subtasks, gaining
up to 4.6x speed-up over state-of-the-art models for dialogue state tracking,
summarization, and question-answering tasks with comparable or better
performance. We release our training/inference code and checkpoints.
更多查看译文
AI 理解论文
溯源树
样例
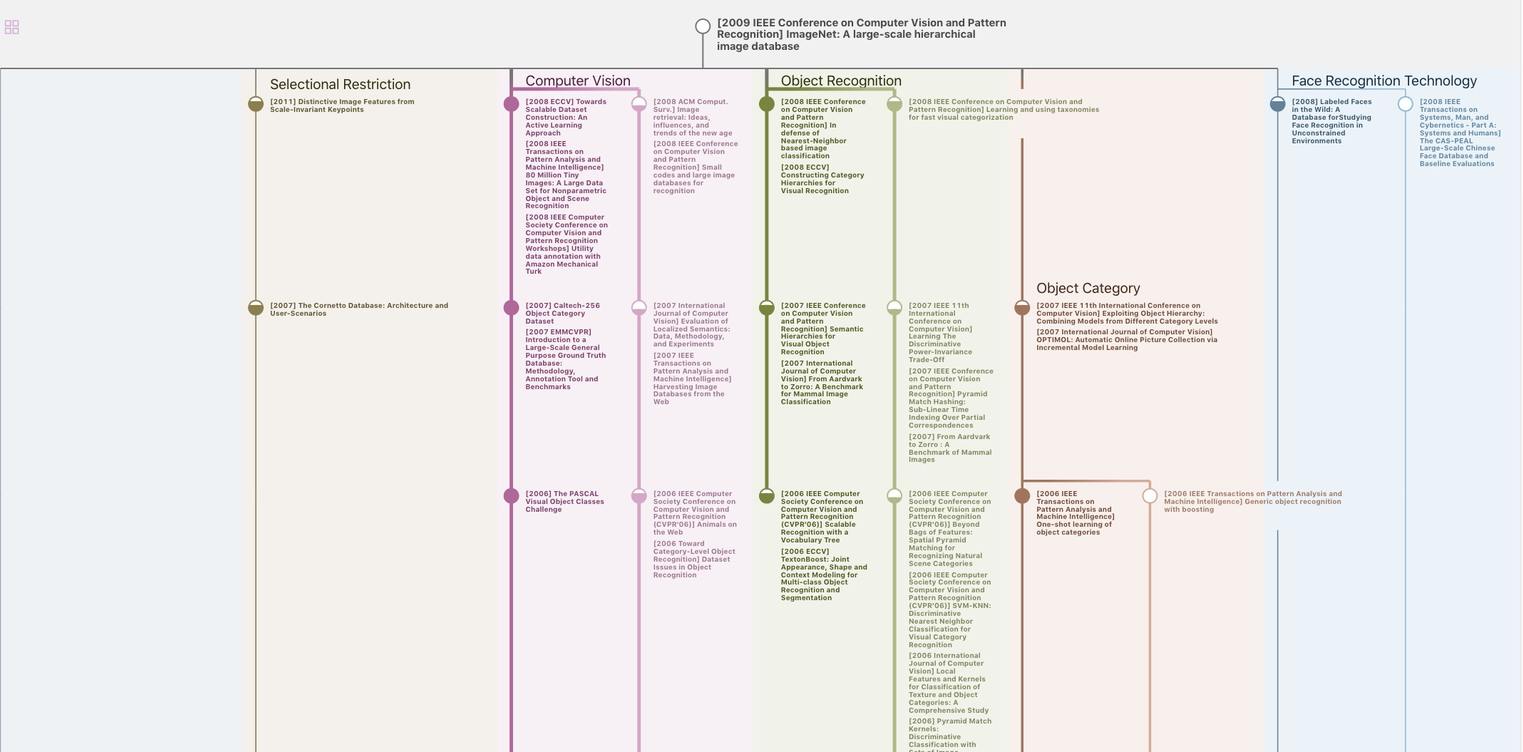
生成溯源树,研究论文发展脉络
Chat Paper
正在生成论文摘要