Few-shot Oriented Object Detection with Memorable Contrastive Learning in Remote Sensing Images
CoRR(2024)
摘要
Few-shot object detection (FSOD) has garnered significant research attention
in the field of remote sensing due to its ability to reduce the dependency on
large amounts of annotated data. However, two challenges persist in this area:
(1) axis-aligned proposals, which can result in misalignment for arbitrarily
oriented objects, and (2) the scarcity of annotated data still limits the
performance for unseen object categories. To address these issues, we propose a
novel FSOD method for remote sensing images called Few-shot Oriented object
detection with Memorable Contrastive learning (FOMC). Specifically, we employ
oriented bounding boxes instead of traditional horizontal bounding boxes to
learn a better feature representation for arbitrary-oriented aerial objects,
leading to enhanced detection performance. To the best of our knowledge, we are
the first to address oriented object detection in the few-shot setting for
remote sensing images. To address the challenging issue of object
misclassification, we introduce a supervised contrastive learning module with a
dynamically updated memory bank. This module enables the use of large batches
of negative samples and enhances the model's capability to learn discriminative
features for unseen classes. We conduct comprehensive experiments on the DOTA
and HRSC2016 datasets, and our model achieves state-of-the-art performance on
the few-shot oriented object detection task. Code and pretrained models will be
released.
更多查看译文
AI 理解论文
溯源树
样例
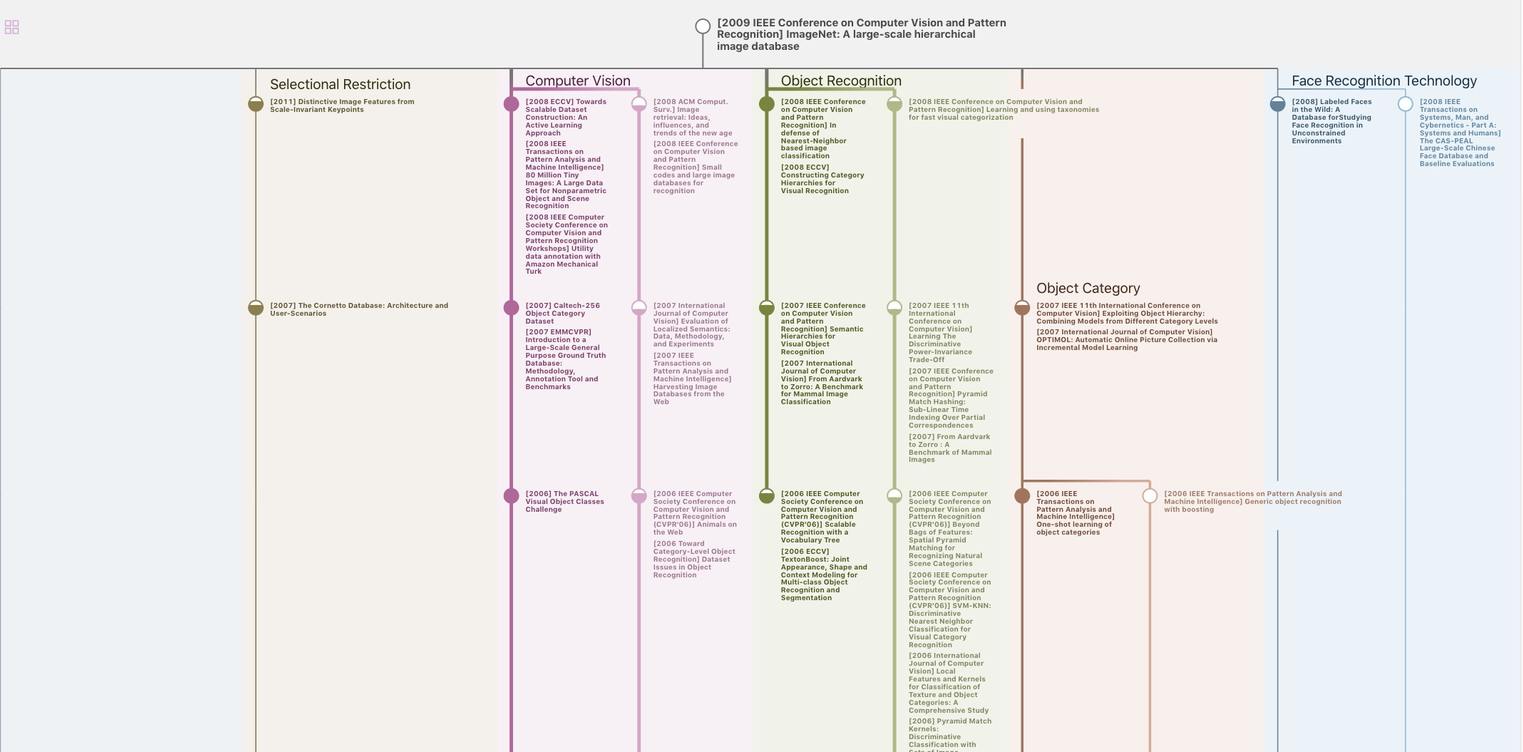
生成溯源树,研究论文发展脉络
Chat Paper
正在生成论文摘要