Scalable Scalar-on-Image Cortical Surface Regression with a Relaxed-Thresholded Gaussian Process Prior
arxiv(2024)
摘要
In addressing the challenge of analysing the large-scale Adolescent Brain
Cognition Development (ABCD) fMRI dataset, involving over 5,000 subjects and
extensive neuroimaging data, we propose a scalable Bayesian scalar-on-image
regression model for computational feasibility and efficiency. Our model
employs a relaxed-thresholded Gaussian process (RTGP), integrating
piecewise-smooth, sparse, and continuous functions capable of both hard- and
soft-thresholding. This approach introduces additional flexibility in feature
selection in scalar-on-image regression and leads to scalable posterior
computation by adopting a variational approximation and utilising the
Karhunen-Loève expansion for Gaussian processes. This advancement
substantially reduces the computational costs in vertex-wise analysis of
cortical surface data in large-scale Bayesian spatial models. The model's
parameter estimation and prediction accuracy and feature selection performance
are validated through extensive simulation studies and an application to the
ABCD study. Here, we perform regression analysis correlating intelligence
scores with task-based functional MRI data, taking into account confounding
factors including age, sex, and parental education level. This validation
highlights our model's capability to handle large-scale neuroimaging data while
maintaining computational feasibility and accuracy.
更多查看译文
AI 理解论文
溯源树
样例
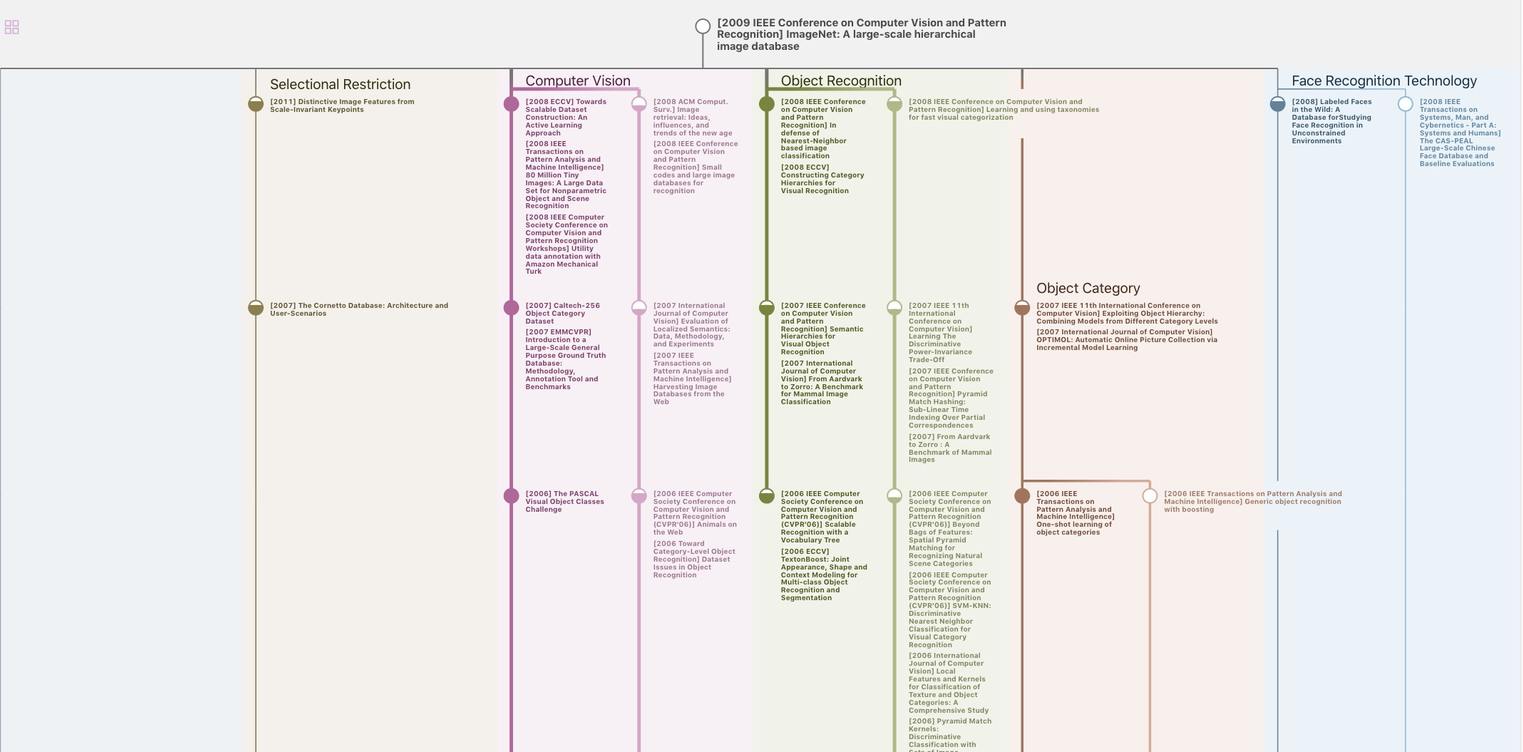
生成溯源树,研究论文发展脉络
Chat Paper
正在生成论文摘要