Data integration of non-probability and probability samples with predictive mean matching
arxiv(2024)
摘要
In this paper we study predictive mean matching mass imputation estimators to
integrate data from probability and non-probability samples. We consider two
approaches: matching predicted to observed (ŷ-y matching) or predicted
to predicted (ŷ-ŷ matching) values. We prove the consistency of
two semi-parametric mass imputation estimators based on these approaches and
derive their variance and estimators of variance. Our approach can be employed
with non-parametric regression techniques, such as kernel regression, and the
analytical expression for variance can also be applied in nearest neighbour
matching for non-probability samples. We conduct extensive simulation studies
in order to compare the properties of this estimator with existing approaches,
discuss the selection of k-nearest neighbours, and study the effects of model
mis-specification. The paper finishes with empirical study in integration of
job vacancy survey and vacancies submitted to public employment offices (admin
and online data). Open source software is available for the proposed
approaches.
更多查看译文
AI 理解论文
溯源树
样例
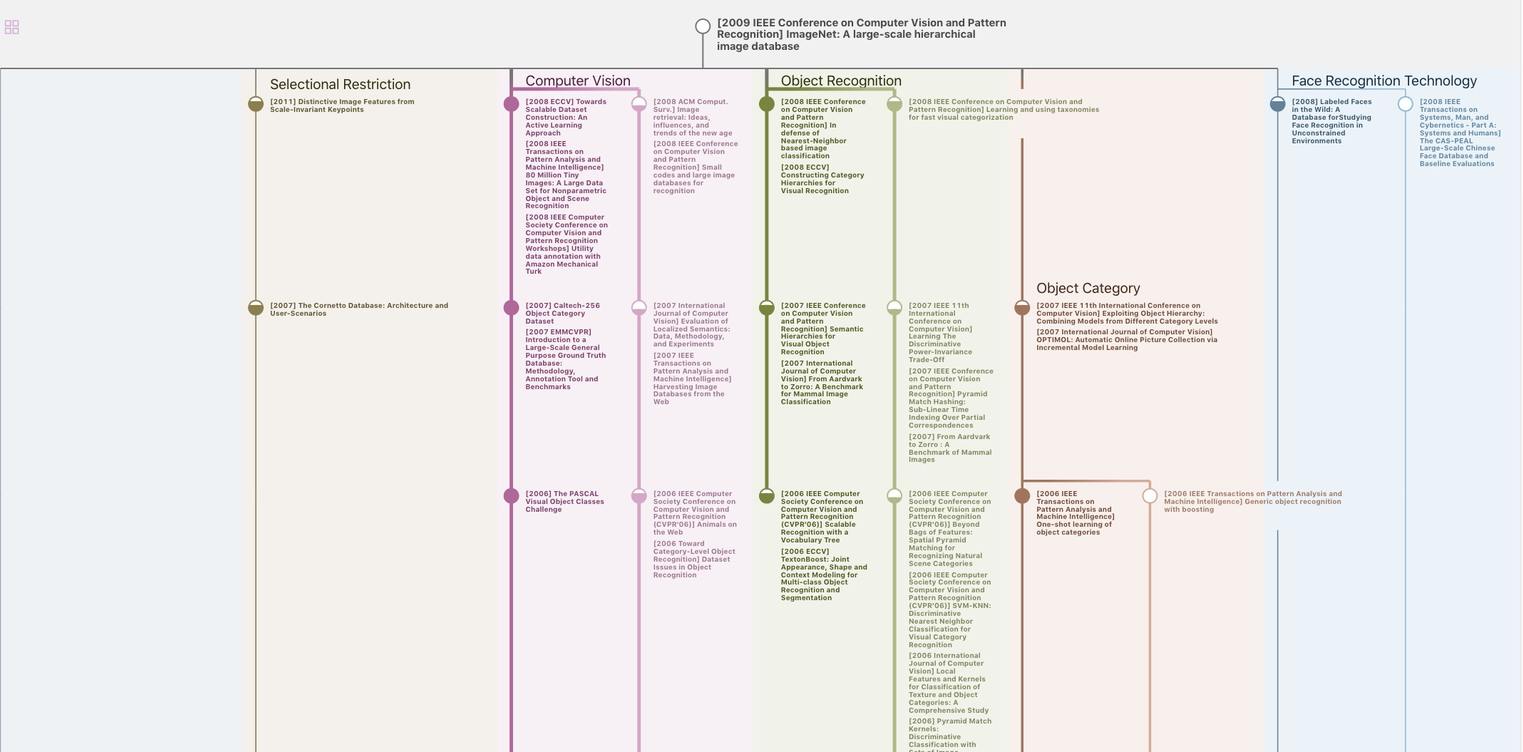
生成溯源树,研究论文发展脉络
Chat Paper
正在生成论文摘要