Toxicity prediction and classification of Gunqile-7 with small sample based on transfer learning method
Computers in Biology and Medicine(2024)
摘要
Drug-induced diseases are the most important component of iatrogenic disease. It is the duty of doctors to provide a reasonable and safe dose of medication. Gunqile-7 is a Mongolian medicine with analgesic and anti-inflammatory effects. As a foreign substance in the body, even with reasonable medication, it may produce varying degrees of adverse reactions or toxic side effects. Since the cost of collecting Gunqile-7 for pharmacological animal trials is high and the data sample is small, this paper employs transfer learning and data augmentation methods to study the toxicity of Gunqile-7. More specifically, to reduce the necessary number of training samples, the data augmentation approach is employed to extend the data set. Then, the transfer learning method and one-dimensional convolutional neural network are utilized to train the network. In addition, we use the support vector machine-recursive feature elimination method for feature selection to reduce features that have adverse effects on model predictions. Furthermore, due to the important role of the pre-trained model of transfer learning, we select a quantitative toxicity prediction model as the pre-trained model, which is consistent with the purpose of this paper. Lastly, the experimental results demonstrate the efficiency of the proposed method. Our method can improve accuracy by up to 9 percentage points compared to the method without transfer learning on a small sample set.
更多查看译文
关键词
Mongolian medicine,Gunqile-7,Feature selection,Transfer learning,Data augmentation,Toxicity classification
AI 理解论文
溯源树
样例
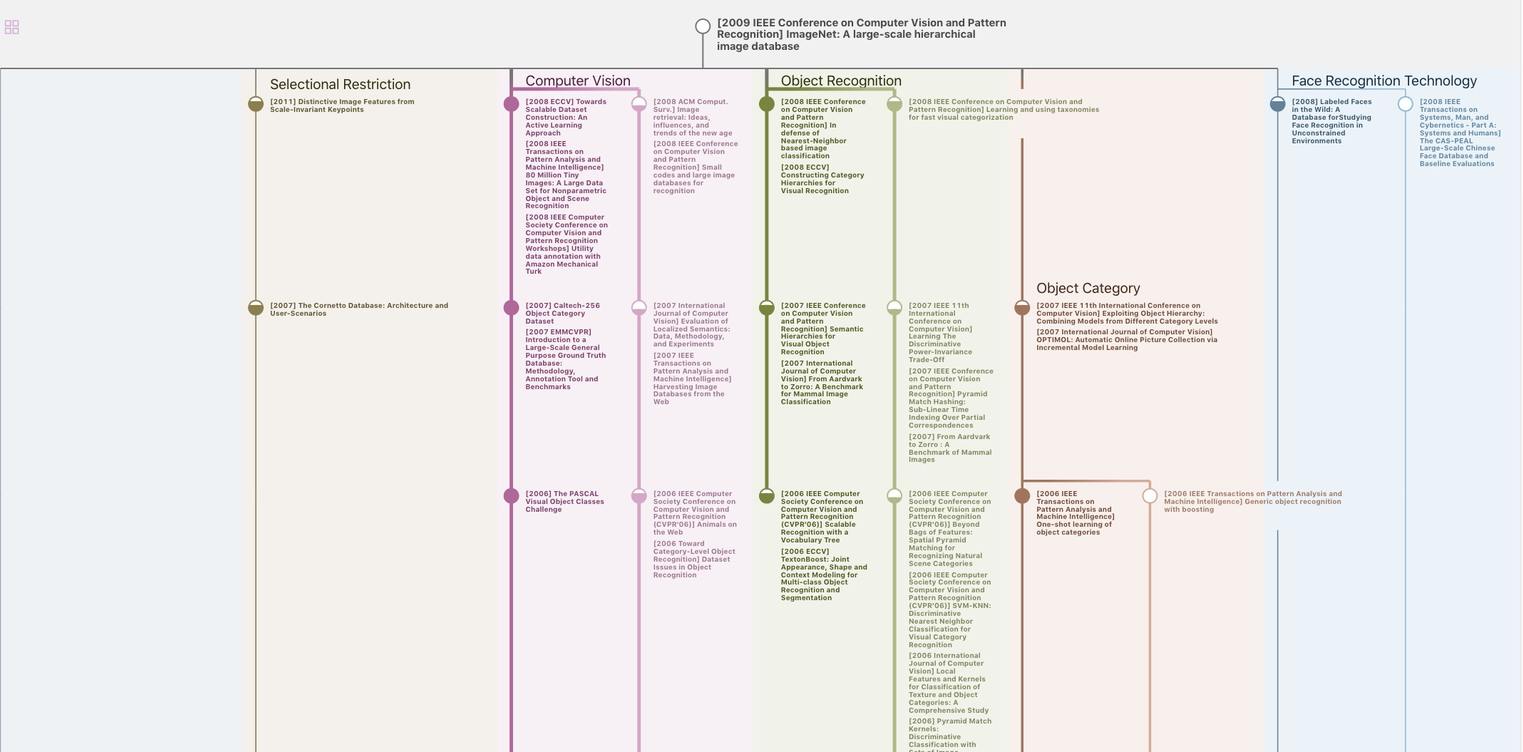
生成溯源树,研究论文发展脉络
Chat Paper
正在生成论文摘要