Transfer learning and pretraining enhanced physics-informed machine learning for closed-circuit reverse osmosis modeling
Desalination(2024)
摘要
Closed-circuit reverse osmosis (CCRO) is a widely concerned batch-type desalination process that exhibits dynamic, multi-mode, and cyclic behavior. This study provides a novel physics-informed machine learning method that integrate pretraining and transfer learning (PT-TL) to construct spatiotemporal model of the CCRO process. In this model, two types of networks are specifically tailored to approximate the latent solutions of the closed-circuit and flushing modes within each running cycle. To facilitate long-time integration of partial differential equations in the closed-circuit mode, time-adaptive decomposition is utilized in parameter transfer learning to identify appropriate sequence partitioning and accelerate the learning process. During the pretraining step, a coarse-grained model is constructed by adjusting the linear initial conditions of the flushing mode to capture time-varying characteristics. The integration of PT-TL with physics-informed machine learning not only reduces training time by over 50 % but also demonstrates comparable predictive ability to traditional numerical methods.
更多查看译文
关键词
Closed-circuit reverse osmosis,Dynamic,Transfer learning,Pretraining,Physics-informed machine learning,Coarse-grained model
AI 理解论文
溯源树
样例
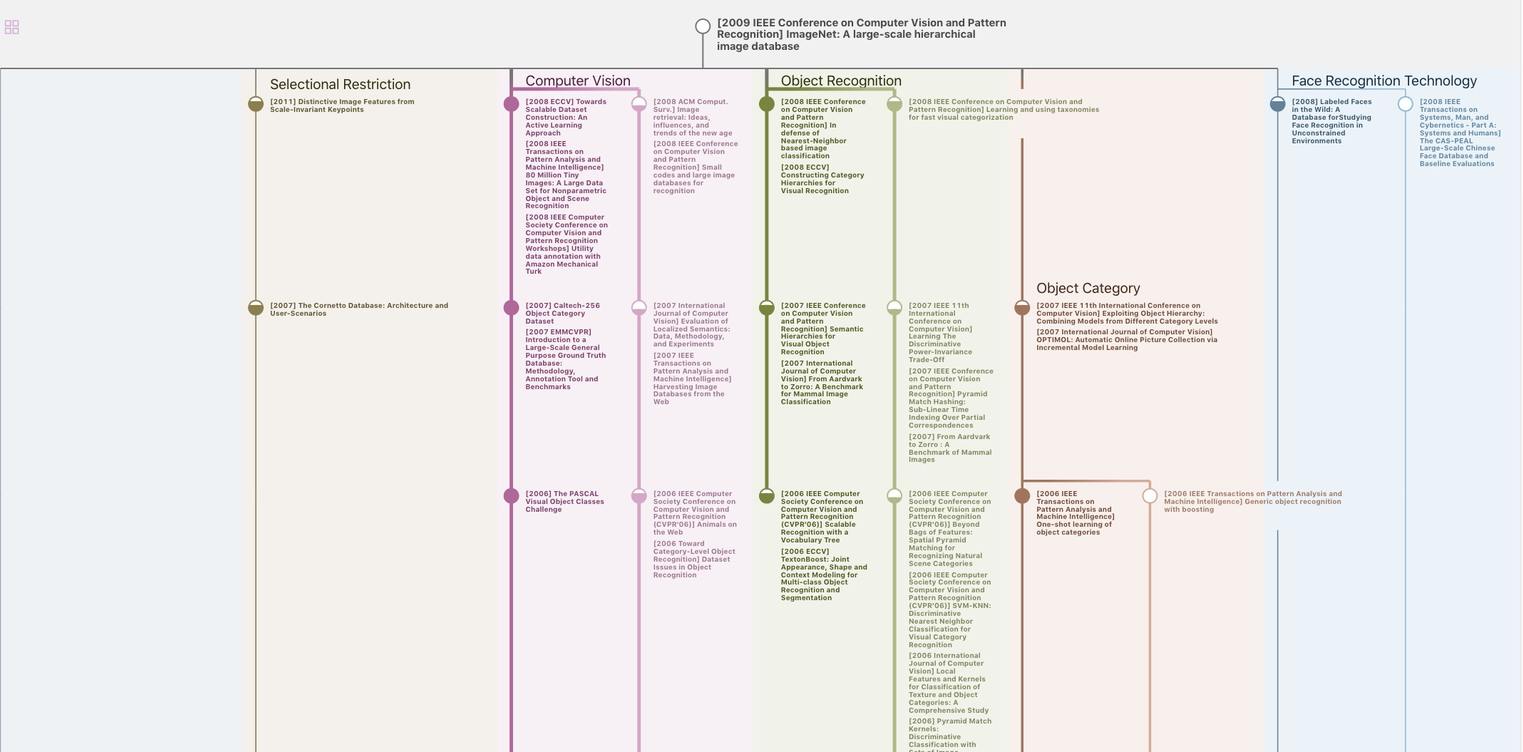
生成溯源树,研究论文发展脉络
Chat Paper
正在生成论文摘要