Synergizing CNN and Random Forest for Enhanced Damping Off Disease Classification in Tomatoes
2024 International Conference on Intelligent and Innovative Technologies in Computing, Electrical and Electronics (IITCEE)(2024)
摘要
The abstract reveals a thorough analysis of numerous evaluation indicators for a classification model's efficiency across ten illness effect levels. The precision variable, which measures how well the model predicts true positives, varies between 6.67% to 93.05%. Recall, which measures how many real positives the model correctly predicted, has a range between 6.25% to 93.26%. The F1-Score, an equal index of recall and precision, ranges from 6.45% to 93.02%. For each disease effect level in the dataset, the ' support' column includes real occurrences, highlighting the significance and representation of every one of them. Also known as "Support proportion," this term denotes the proportion of examples for every single level in a data set. The accuracy statistic demonstrates the model's overall accuracy in classifying the various illness impact levels, with a total accuracy percentage of 88.617%. Macro and micro averages also feature in the evaluation to give a broader picture of the model's performance. The macro average determines the assessment metrics' unweighted mean, whereas the micro average takes a weighted mean into account and corrects for class imbalances. The evaluation thoroughly evaluates the classification model's effectiveness in determining illness impact levels, offering insightful information about its effectiveness and adaptability for the intended task. To accomplish the results, the model architecture is comprised of 4 layers for convolution, 4 maximally paired layers, and 1 interconnected layer.
更多查看译文
关键词
Diseases,Precision,Forest,Class
AI 理解论文
溯源树
样例
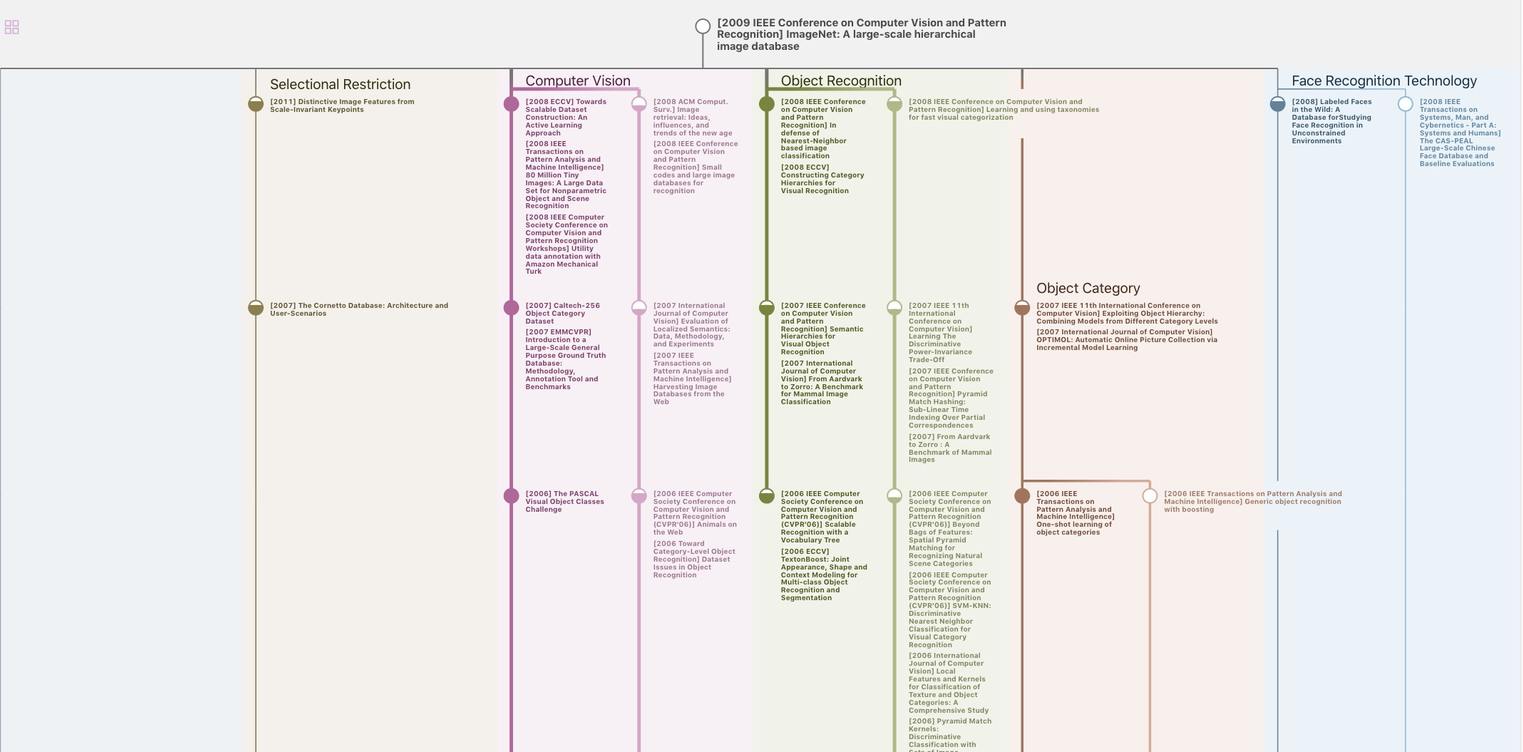
生成溯源树,研究论文发展脉络
Chat Paper
正在生成论文摘要