Multimodal Collaboration Networks for Geospatial Vehicle Detection in Dense, Occluded, and Large-Scale Events
IEEE TRANSACTIONS ON GEOSCIENCE AND REMOTE SENSING(2024)
摘要
In large-scale disaster events, the planning of optimal rescue routes depends on the object detection ability at the disaster scene, with one of the main challenges being the presence of dense and occluded objects. Existing methods, which are typically based on the RGB modality, struggle to distinguish targets with similar colors and textures in crowded environments and are unable to identify obscured objects. To this end, we first construct two multimodal dense and occlusion vehicle detection datasets for large-scale events, utilizing RGB and height map modalities. Based on these datasets, we propose a multimodal collaboration network (MuDet) for dense and occluded vehicle detection, MuDet for short. MuDet hierarchically enhances the completeness of discriminable information within and across modalities and differentiates between simple and complex samples. MuDet includes three main modules: Unimodal Feature Hierarchical Enhancement (Uni-Enh), Multimodal Cross Learning (Mul-Lea), and Hard-easy Discriminative (He-Dis) Pattern. Uni-Enh and Mul-Lea enhance the features within each modality and facilitate the cross-integration of features from two heterogeneous modalities. He-Dis effectively separates densely occluded vehicle targets with significant intra-class differences and minimal inter-class differences by defining and thresholding confidence values, thereby suppressing the complex background. Experimental results on two re-labeled multimodal benchmark datasets, the 4K Stereo Aerial Imagery of a Large Camping Site (4K-SAI-LCS) dataset, and the ISPRS Potsdam dataset, demonstrate the robustness and generalization of the MuDet.
更多查看译文
关键词
Vehicle detection,Feature extraction,Object detection,Disasters,Streaming media,Remote sensing,Convolutional neural networks,Convolutional neural networks (CNNs),dense and occluded,hard-easy balanced attention,large-scale disaster events,multimodal vehicle detection (MVD),remote Sensing (RS)
AI 理解论文
溯源树
样例
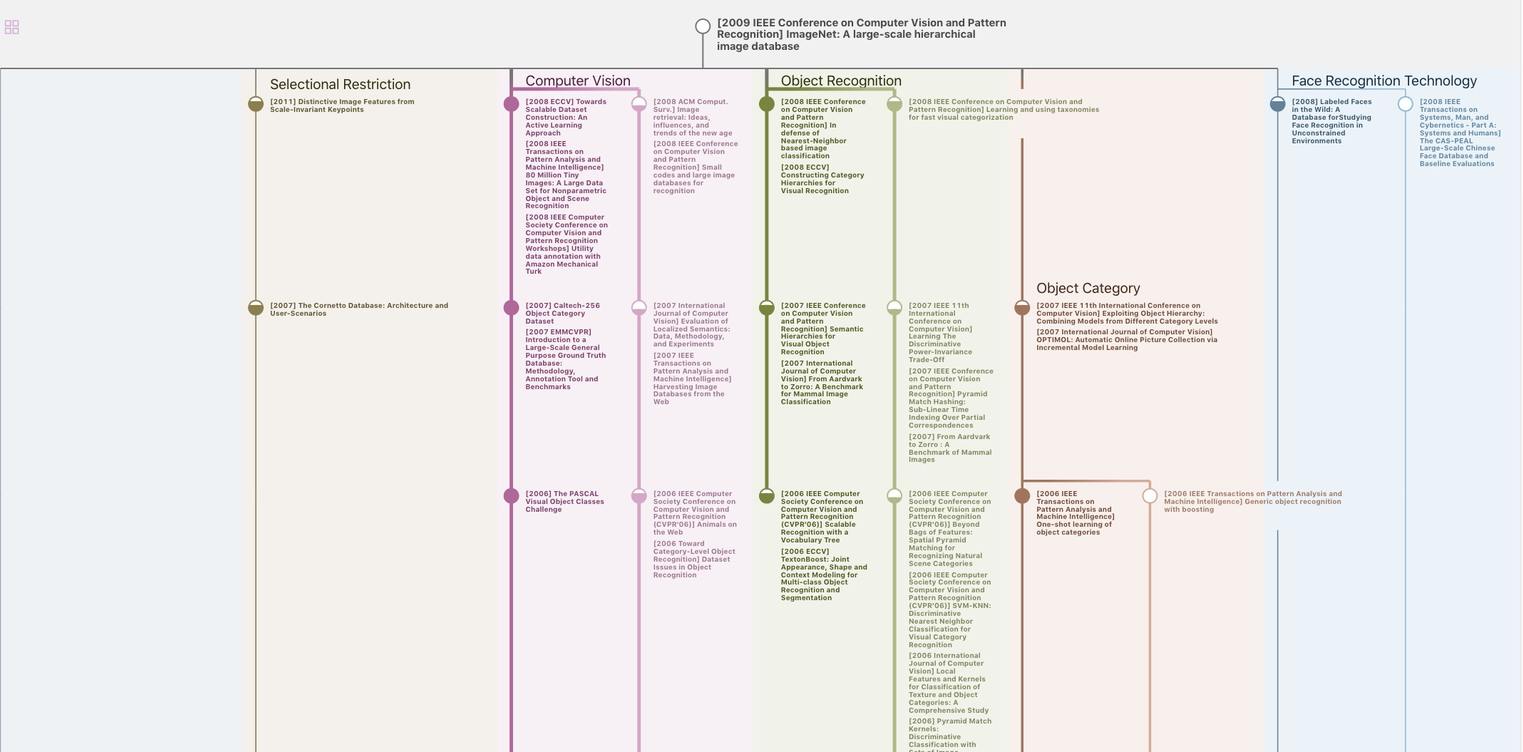
生成溯源树,研究论文发展脉络
Chat Paper
正在生成论文摘要