Domain-Generalized Robotic Picking via Contrastive Learning-Based 6-D Pose Estimation
IEEE TRANSACTIONS ON INDUSTRIAL INFORMATICS(2024)
摘要
Vision-guided robotic picking in 3-D space is a key technology for industrial automation and intelligent manufacturing. However, existing methods rely on labeled real-world data for learning, significantly limiting their ability to generalize to novel objects and robustness to challenging scenes containing occlusions and clutter. To address these problems, we propose a domain-generalized robotic picking method (DGPF6D) that builds on contrastive learning-based 6-D pose estimation. DGPF6D generalizes to real-world scenes by training only on synthetic data and without using shape priors. Specifically, we first perform continuous data augmentations on the synthetic RGB and point cloud images such that they can better simulate real-world scenes with occlusions and clutter. We then feed the augmented images in parallel to a two-stage (i.e., 3-D shape reconstruction and 6-D pose estimation) contrastive learning framework, thereby enhancing the domain-generalization ability and robustness of DGPF6D. Moreover, we propose a point cloud cross attention-guided intracategory unknown object 3-D shape reconstruction network, which can effectively fuse the observed and the unit random point clouds and explicitly highlight their differences, thus avoiding the dependence of DGPF6D on shape priors. Finally, we build a robotic picking system employing DGPF6D to realize domain-generalized robotic picking in 3-D space. Extensive experiments on two benchmarks and real-world scenes show that DGPF6D achieves state-of-the-art performance, and can be effectively applied for domain-generalized robotic picking.
更多查看译文
关键词
Shape,Robots,Pose estimation,Point cloud compression,Robot kinematics,Image reconstruction,Self-supervised learning,6D pose estimation,contrastive learning,domain generalization,robotic picking
AI 理解论文
溯源树
样例
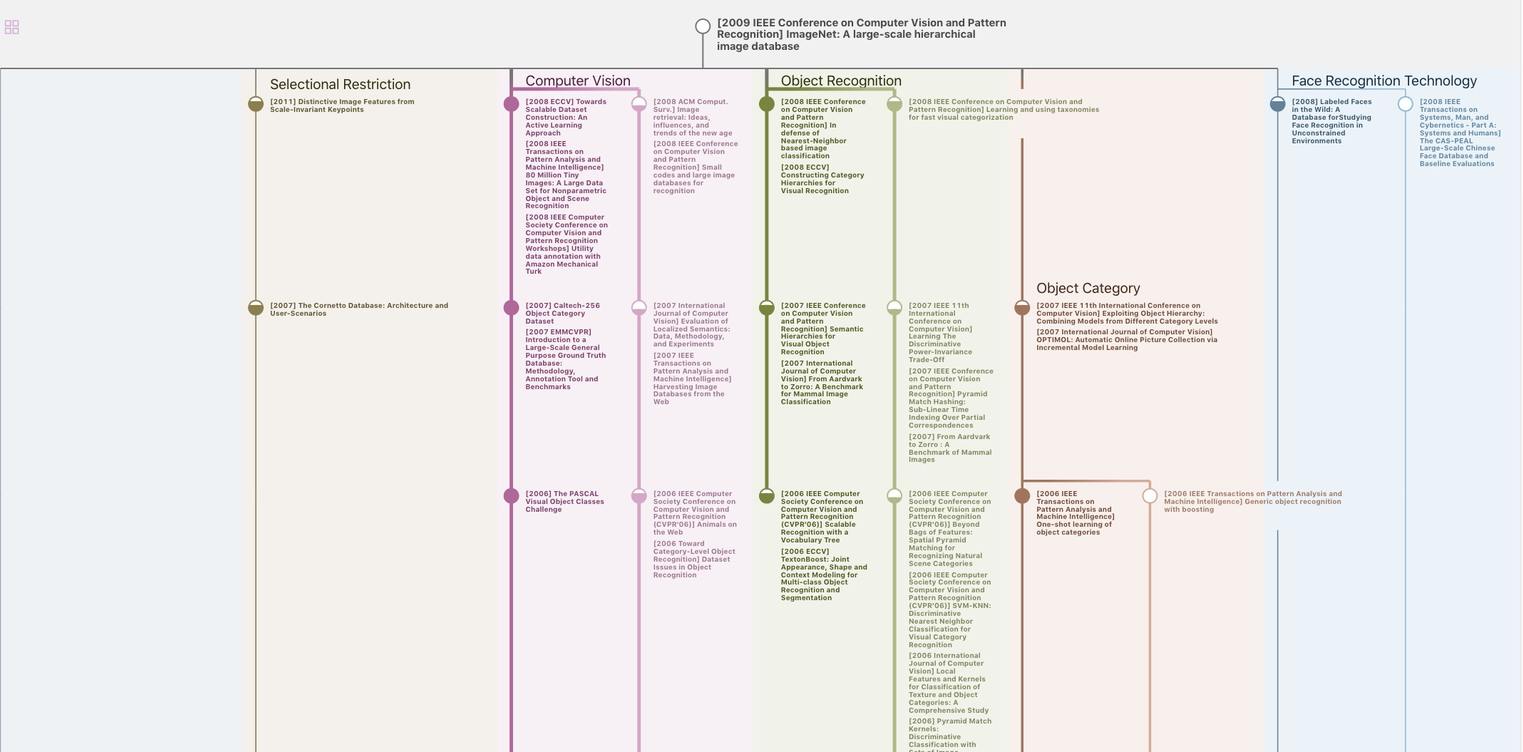
生成溯源树,研究论文发展脉络
Chat Paper
正在生成论文摘要