Reflectance Multispectral Imaging for Fungal Growth Level Estimation
2024 IEEE 3rd International Conference on Control, Instrumentation, Energy & Communication (CIEC)(2024)
摘要
Fungal contamination in food is a serious concern in food safety due to the harmful toxins it produces. Methods available in the current state of the art for fungal detection tend to be expensive and time consuming. To overcome this, Multispectral Imaging provides a practical and cost effective solution to estimate fungal growth levels. In this study, multispectral images are obtained via a simple, easy to use, in house built imaging system which facilitates onsite testing. It utilizes 13 spectral bands with peak wavelengths ranging from 365 nm to 940 nm. A meticulous procedure is followed when synthesizing fungal contaminated food samples. Fungal contaminated bread samples are imaged in several stages such as Day 0, Day 3, Day 5, and Day 6. A total of 1344 individual images are utilized for training and testing of the developed models with an 80% - 20% test-train split. Several preprocessing techniques are followed including dark current reduction. Principle Component Analysis (PCA) is employed as the dimensional reduction technique for the analysis. Classification models and a regression model as a functional map are developed for a better interpretation of the results. The classification model is validated with an accuracy of 95% for the KNN and SVM classifiers while the developed functional map modeled with a first order polynomial is proved to be accurate with a $R^{2}$ value of 0.989.
更多查看译文
关键词
Multispectral imaging,Food quality,Aspergillus,Fungus detection,Machine learning
AI 理解论文
溯源树
样例
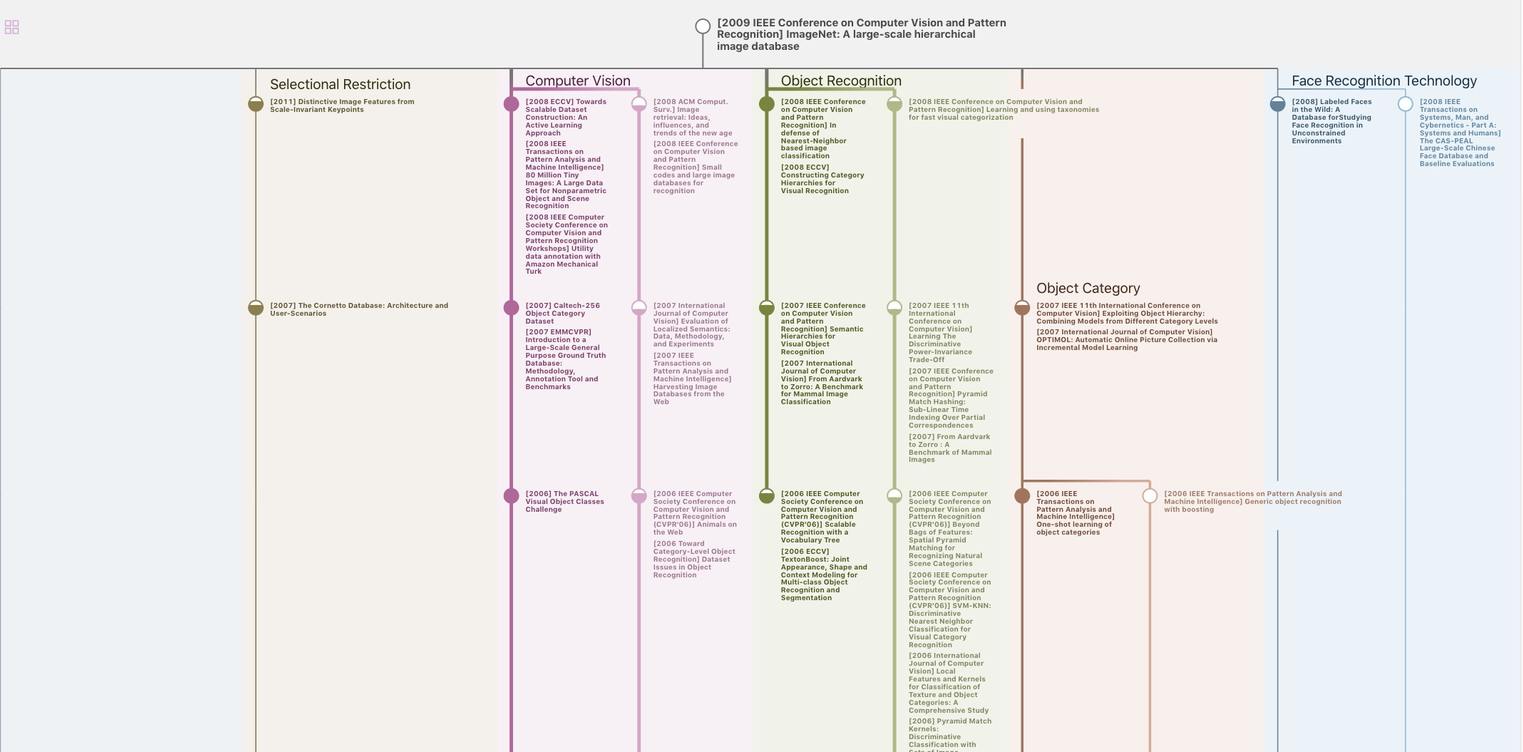
生成溯源树,研究论文发展脉络
Chat Paper
正在生成论文摘要