Shape generation via learning an adaptive multimodal prior
The Visual Computer(2024)
摘要
Significant interest and progress have been drawn to the recent advancements in image creation using deep generative model, but the field of automatic three-dimensional shape creation is largely under-developed and inspires a great deal of research activity across a wide variety of disciplines. We add a new kind of previously named variational mixture of posteriors into the adversarial network using geometric data described as volumetric grids. Our main contribution is the introduction of a new type of prior called variational mixture of posteriors prior into the adversarial network, dubbed VampPrior-3DGAN, in a mathematic principled way. Specifically, we leverage an encoder as a regularizer to penalize missing modes, while introduce a variational mixture of posterior prior as the latent variable distribution of GAN to dynamically and adaptively update its prior distribution. The key intuition behind this architecture is that the latent variables should retain information about the data to minimize the undue impact of the prior assumptions. This seemingly simple modification to the GAN framework is surprisingly effective and results in models which enable diversity in generated samples, although trained with limited data. Realistic 3D objects can be easily generated by sampling the VampPrior-3DGAN’s latent probabilistic manifold. For validation, we apply our method on tasks from the fields of three-dimensional volumetric generation, reconstruction from a single RGB image and partial shape completion from a single perspective view, and show that it is on par with or outperforms the state-of-the-art approaches, both quantitatively and qualitatively.
更多查看译文
关键词
Shape generation,Variational mixture of posteriors prior,GAN,Diversity
AI 理解论文
溯源树
样例
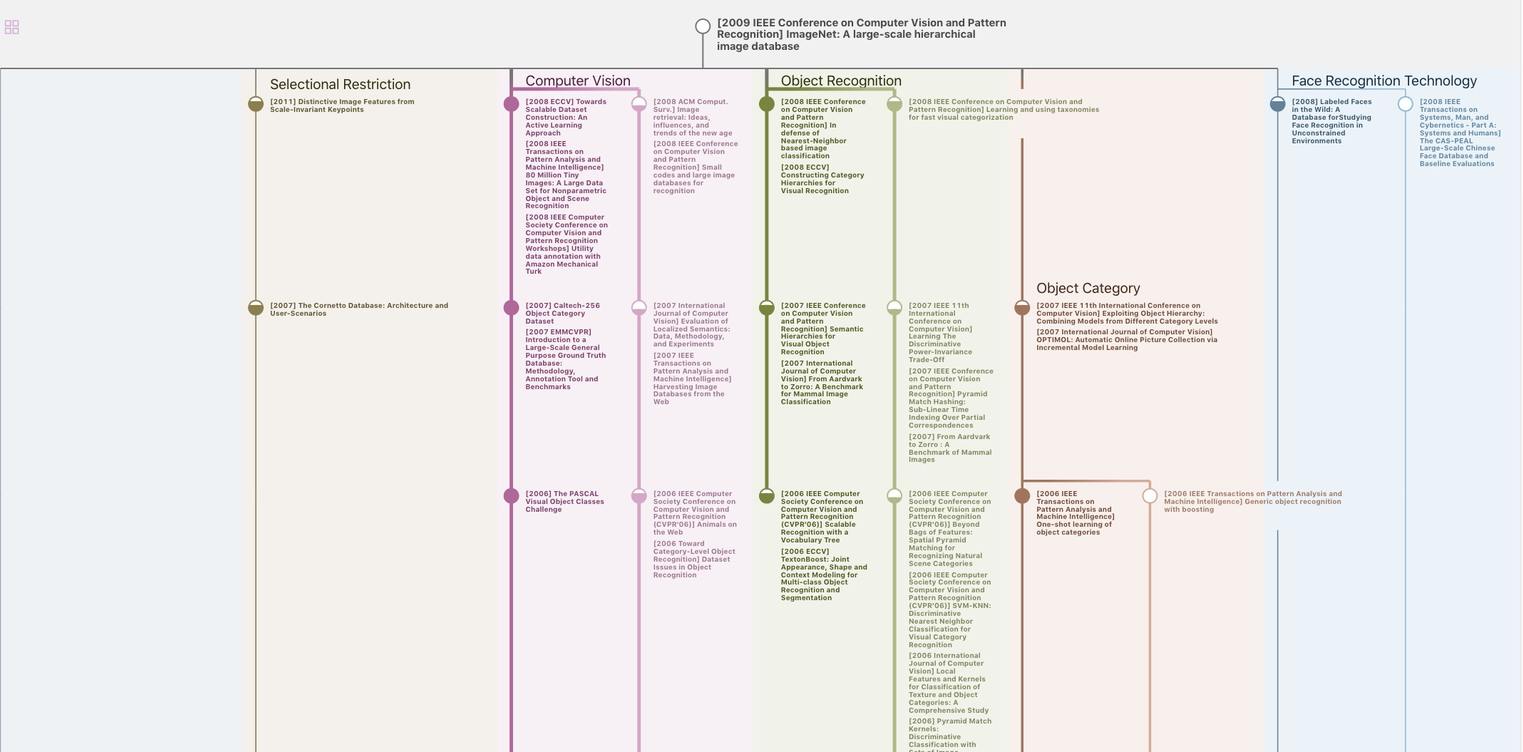
生成溯源树,研究论文发展脉络
Chat Paper
正在生成论文摘要