Insights and reviews on battery lifetime prediction from research to practice
Journal of Energy Chemistry(2024)
摘要
The rising demand for energy storage solutions, especially in the electric vehicle and renewable energy sectors, highlights the importance of accurately predicting battery health to enhance their longevity and reliability. This article comprehensively examines various methods used to forecast battery health, including physics-based models, empirical models, and equivalent circuit models, among others. It delves into the promise of data-driven prognostics, utilizing both conventional machine learning and cutting-edge deep neural network techniques. The advantages and limitations of hybrid models are thoroughly analyzed, with a focus on the benefits of integrating diverse data sources to improve prognostic precision. Through practical case studies, the article showcases the effectiveness and flexibility of these approaches. It also critically addresses the challenges encountered in applying battery health prognostics in real-world scenarios, such as issues of scalability, complexity, and data anomalies. Despite these challenges, the article underscores the emerging opportunities brought about by recent technological, academic, and research advancements. These include the development of digital twin models for batteries, the use of data-centric AI and standardized benchmarking, the potential integration of blockchain technology for enhanced data security and transparency, and the synergy between edge and cloud computing to boost data analysis and processing. The primary goal of this article is to enrich the understanding of current battery health prognostic techniques and to inspire further research aimed at overcoming existing hurdles and tapping into new opportunities. It concludes with a visionary perspective on future research directions and potential developments in this evolving field, encouraging both researchers and practitioners to explore innovative solutions.
更多查看译文
关键词
Batteries,Lifetime,Health,Prediction,Data-driven,Machine learning,Deep learning,Field,Real world
AI 理解论文
溯源树
样例
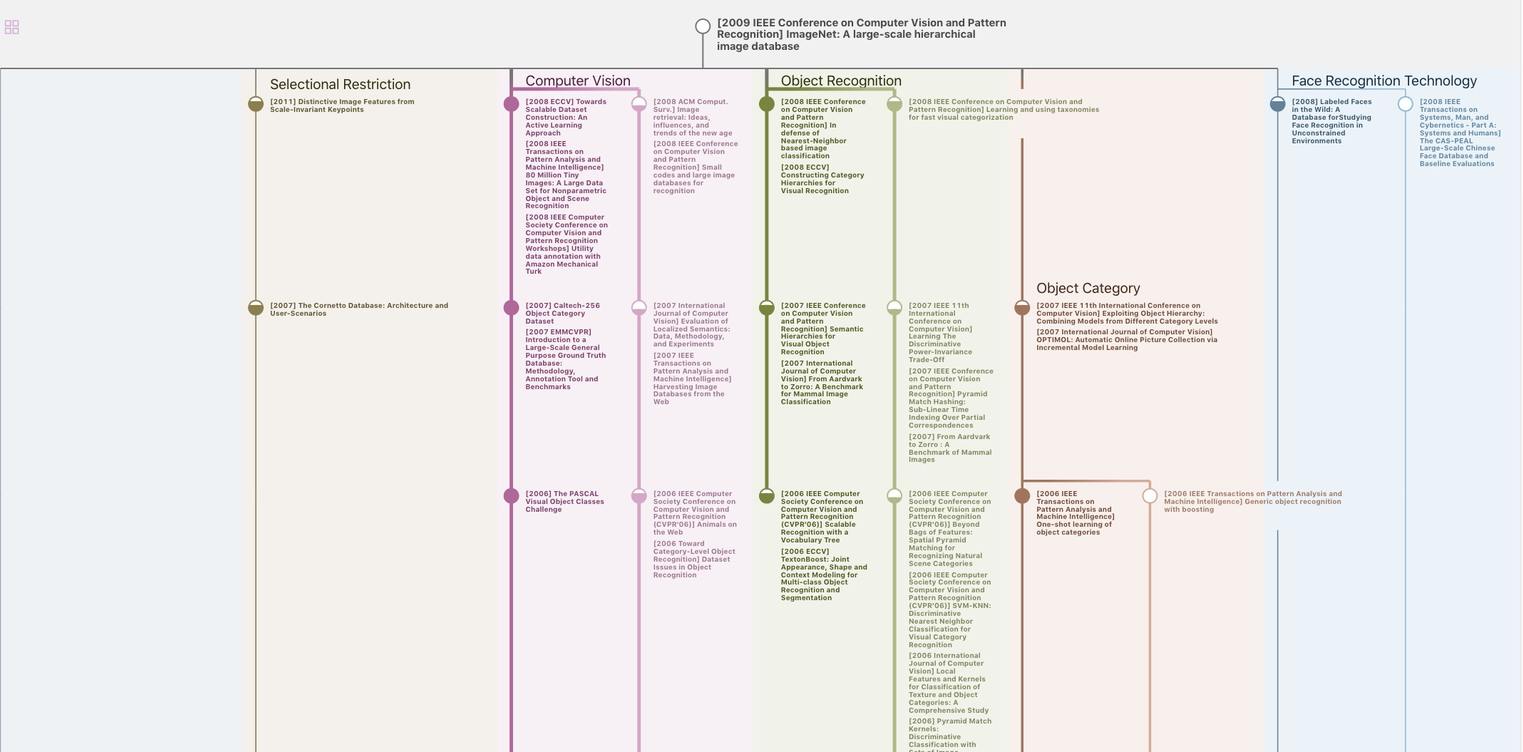
生成溯源树,研究论文发展脉络
Chat Paper
正在生成论文摘要