Referring Image Editing: Object-level Image Editing Via Referring Expressions
CVPR 2024(2024)
摘要
Significant advancements have been made in image editing with the recent advance of the Diffusion model. How-ever, most of the current methods primarily focus on global or subject-level modifications, and often face limitations when it comes to editing specific objects when there are other objects coexisting in the scene, given solely textual prompts. In response to this challenge, we introduce an object-level generative task called Referring Image Editing (RIE), which enables the identification and editing of specific source objects in an image using text prompts. To tackle this task effectively, we propose a tailoredframework called ReferDiffusion. It aims to disentangle input prompts into multiple embeddings and employs a mixed-supervised multi-stage training strategy. To facilitate further research in this domain, we introduce the Ref COCO-Edit dataset, comprising images, editing prompts, source object segmen-tation masks, and reference edited images for training and evaluation. Our extensive experiments demonstrate the ef-fectiveness of our approach in identifying and editing tar-get objects, while conventional general image editing and region-based image editing methods have difficulties in this challenging task.
更多查看译文
关键词
Reference Image,Image Editing,Specific Objectives,Image Object,Training Strategy,Diffusion Model,Target Object,Region-based Methods,Transformer,Image Quality,Generalization Ability,Ability Of The Model,Diffusion Process,Recurrent Neural Network,Super-resolution,Bounding Box,Latent Space,Image Generation,Source Images,Output Image,Image Inpainting,Forward Process,Objects In The Scene,Backward Process,Variational Autoencoder,Correct Object,Ground Truth Reference,Reference Output,Fully Convolutional Network
AI 理解论文
溯源树
样例
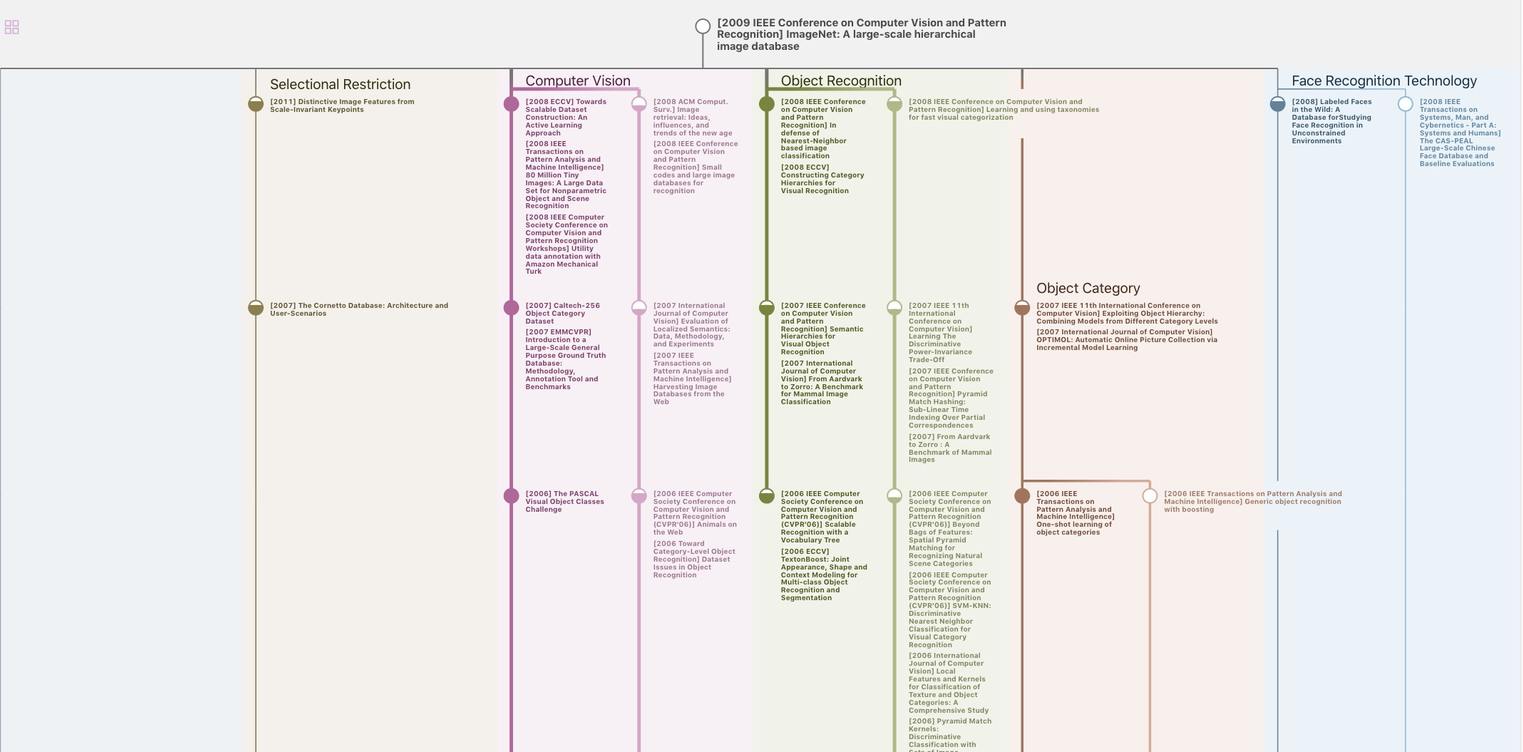
生成溯源树,研究论文发展脉络
Chat Paper
正在生成论文摘要