Modality-Collaborative Test-Time Adaptation for Action Recognition
CVPR 2024(2024)
摘要
Video-based Unsupervised Domain Adaptation (VUDA) method improves the generalization of the video model, en-abling it to be applied to action recognition tasks in different environments. However, these methods require contin-uous access to source data during the adaptation process, which are impractical in real scenarios where the source videos are not available with concerns in transmission efficiency or privacy issues. To address this problem, in this paper, we focus on the Multimodal Video Test- Time Adaptation (MVTTA) task. Existing image-based TTA methods cannot be directly applied to this task because videos have domain shifts in multimodal and temporal, which brings difficulties to adaptation. To address the above challenges, we propose a Modality-Collaborative Test-Time Adaptation (MC-TTA) Network. MC-TTA contains maintain teacher and student memory banks respectively for generating pseudo-prototypes and target-prototypes. In the teacher model, we propose Self-assembled Source-friendly Feature Reconstruction (SSFR) to encourage the teacher memory bank to store features that are more likely to be consistent with the source distribution. Through multimodal prototype alignment and cross-modal relative consistency, our method can effectively alleviate domain shift in videos. We evaluate the proposed model on four public video datasets. The results show that our model outperforms existing state-of-the-art methods.
更多查看译文
关键词
Multi-modal learning
AI 理解论文
溯源树
样例
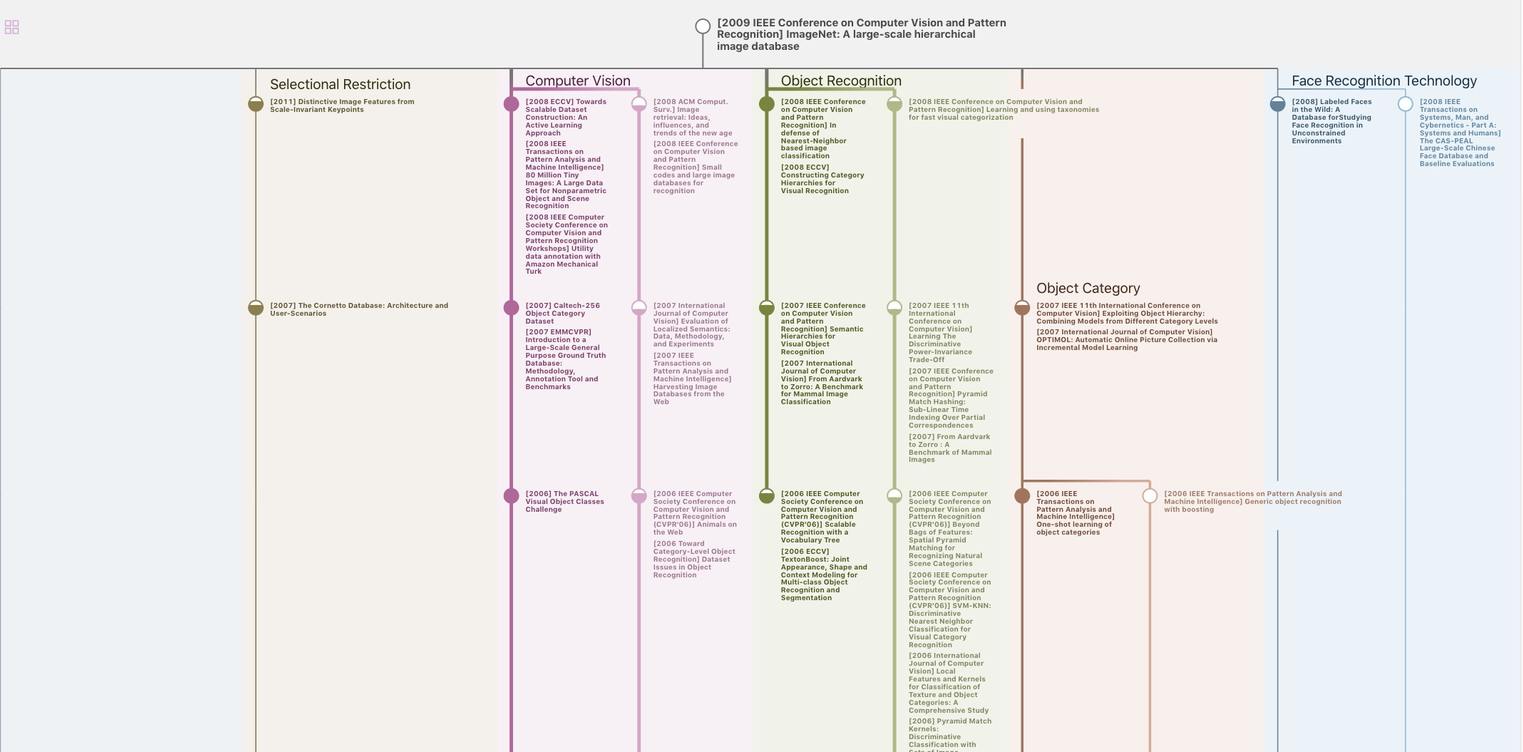
生成溯源树,研究论文发展脉络
Chat Paper
正在生成论文摘要