Pre-trained Vision and Language Transformers Are Few-Shot Incremental Learners.
2024 IEEE/CVF Conference on Computer Vision and Pattern Recognition (CVPR)(2024)
Abstract
Few-Shot Class Incremental Learning (FSCIL) is a task that requires a modelto learn new classes incrementally without forgetting when only a few samplesfor each class are given. FSCIL encounters two significant challenges:catastrophic forgetting and overfitting, and these challenges have driven priorstudies to primarily rely on shallow models, such as ResNet-18. Even thoughtheir limited capacity can mitigate both forgetting and overfitting issues, itleads to inadequate knowledge transfer during few-shot incremental sessions. Inthis paper, we argue that large models such as vision and language transformerspre-trained on large datasets can be excellent few-shot incremental learners.To this end, we propose a novel FSCIL framework called PriViLege, Pre-trainedVision and Language transformers with prompting functions and knowledgedistillation. Our framework effectively addresses the challenges ofcatastrophic forgetting and overfitting in large models through new pre-trainedknowledge tuning (PKT) and two losses: entropy-based divergence loss andsemantic knowledge distillation loss. Experimental results show that theproposed PriViLege significantly outperforms the existing state-of-the-artmethods with a large margin, e.g., +9.38+13.36https://github.com/KHU-AGI/PriViLege.
MoreTranslated text
Key words
Incremental learning,Few-shot learning,Parameter Efficient Tuning
AI Read Science
Must-Reading Tree
Example
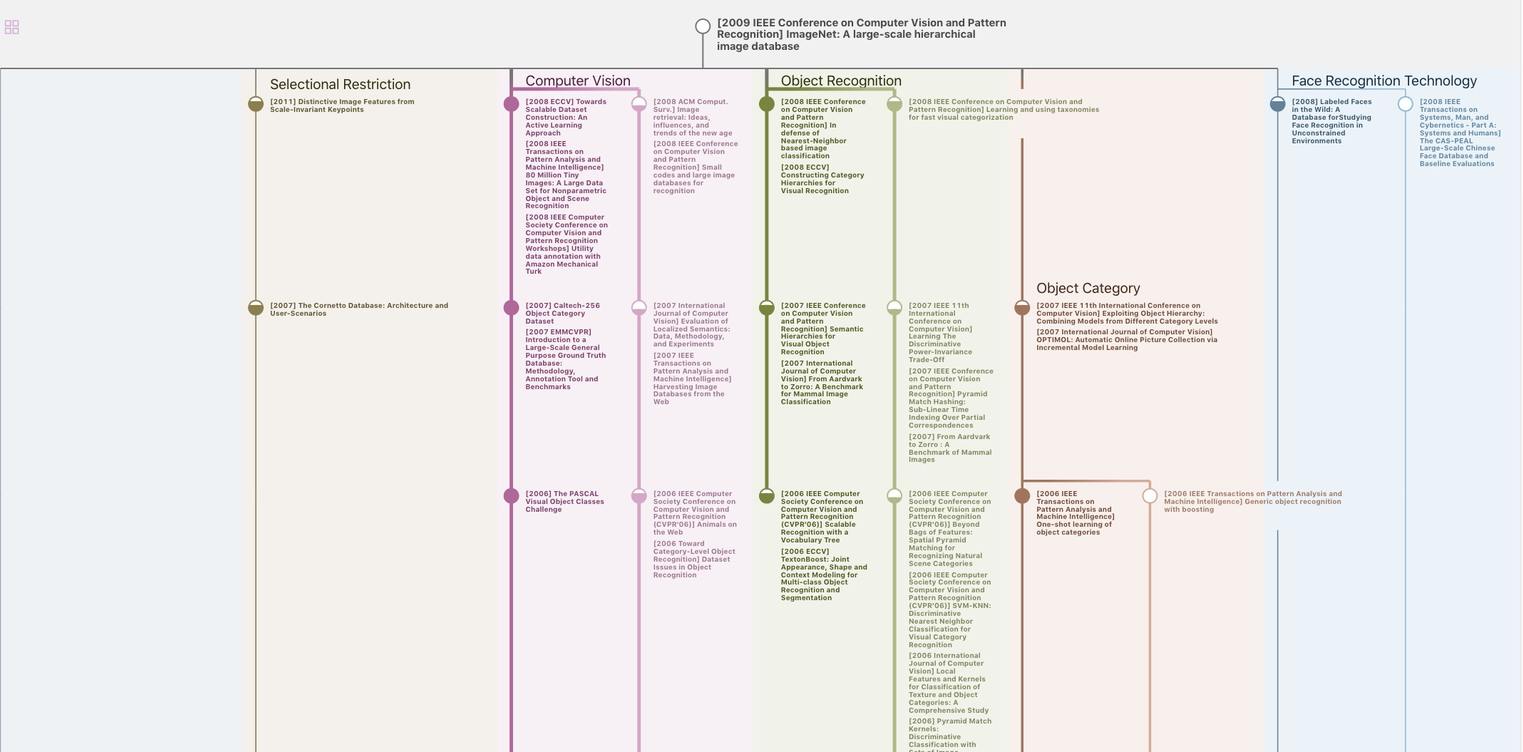
Generate MRT to find the research sequence of this paper
Chat Paper
Summary is being generated by the instructions you defined