Unified Entropy Optimization for Open-Set Test-Time Adaptation
2024 IEEE/CVF Conference on Computer Vision and Pattern Recognition (CVPR)(2024)
摘要
Test-time adaptation (TTA) aims at adapting a model pre-trained on thelabeled source domain to the unlabeled target domain. Existing methods usuallyfocus on improving TTA performance under covariate shifts, while neglectingsemantic shifts. In this paper, we delve into a realistic open-set TTA settingwhere the target domain may contain samples from unknown classes. Manystate-of-the-art closed-set TTA methods perform poorly when applied to open-setscenarios, which can be attributed to the inaccurate estimation of datadistribution and model confidence. To address these issues, we propose a simplebut effective framework called unified entropy optimization (UniEnt), which iscapable of simultaneously adapting to covariate-shifted in-distribution (csID)data and detecting covariate-shifted out-of-distribution (csOOD) data.Specifically, UniEnt first mines pseudo-csID and pseudo-csOOD samples from testdata, followed by entropy minimization on the pseudo-csID data and entropymaximization on the pseudo-csOOD data. Furthermore, we introduce UniEnt+ toalleviate the noise caused by hard data partition leveraging sample-levelconfidence. Extensive experiments on CIFAR benchmarks and Tiny-ImageNet-C showthe superiority of our framework. The code is available athttps://github.com/gaozhengqing/UniEnt
更多查看译文
关键词
Test-Time Adaptation
AI 理解论文
溯源树
样例
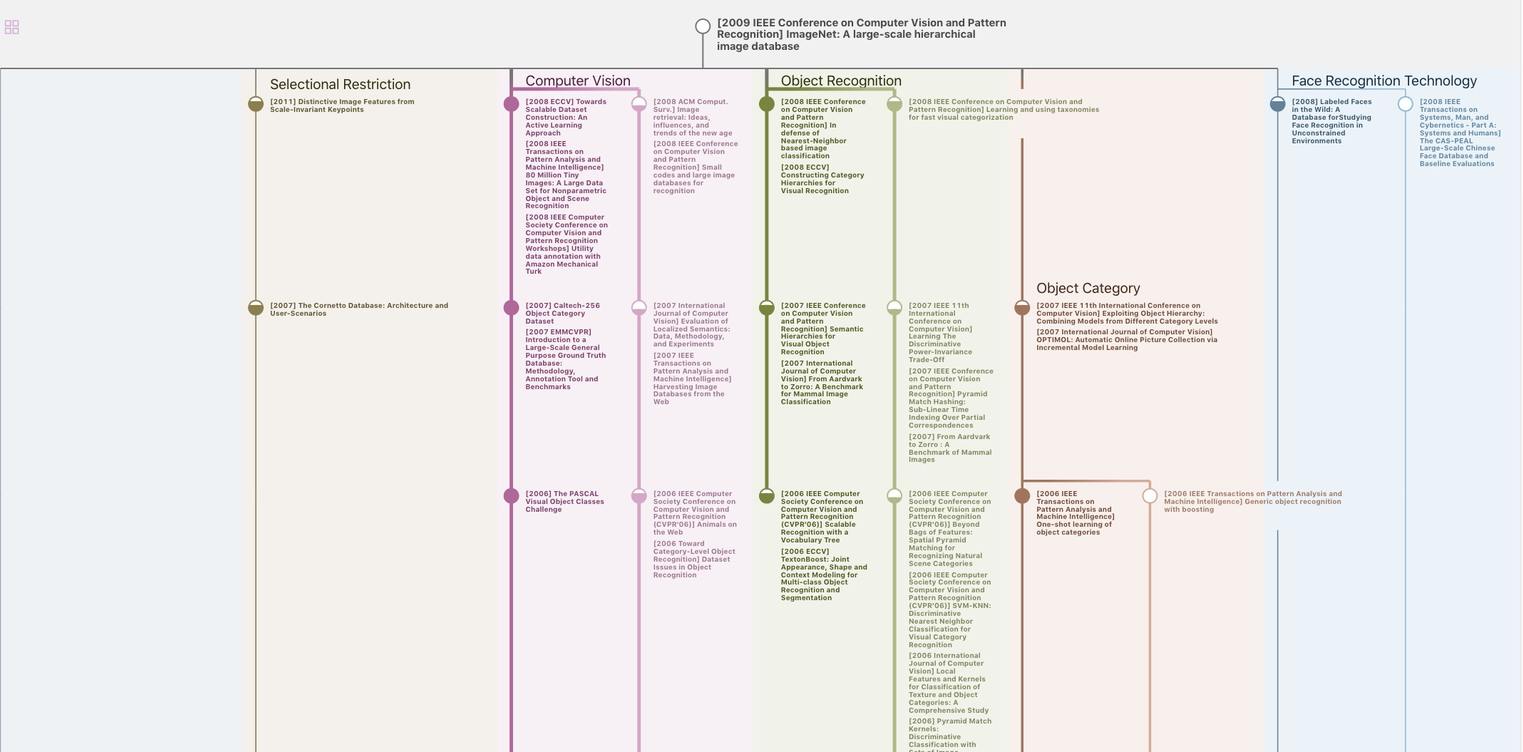
生成溯源树,研究论文发展脉络
Chat Paper
正在生成论文摘要