RankMatch: Exploring the Better Consistency Regularization for Semi-supervised Semantic Segmentation
CVPR 2024(2024)
摘要
The key lie in semi-supervised semantic segmentation is how to fully exploit substantial unlabeled data to im-prove the model's generalization performance by resorting to constructing effective supervision signals. Most methods tend to directly apply contrastive learning to seek additional supervision to complement independent regular pixel-wise consistency regularization. However, these methods tend not to be preferred ascribed to their complicated designs, heavy memory footprints and susceptibility to confirmation bias. In this paper, we analyze the bottlenecks exist in con-trastive learning-based methods and offer a fresh perspective on inter-pixel correlations to construct more safe and effective supervision signals, which is in line with the nature of semantic segmentation. To this end, we develop a coherent RankMatch network, including the construction of representative agents to model inter-pixel correlation beyond regular individual pixel-wise consistency, and fur-ther unlock the potential of agents by modeling inter-agent relationships in pursuit of rank-aware correlation consis-tency. Extensive experimental results on multiple bench-marks, including mitochondria segmentation, demonstrate that RankMatch performs favorably against state-of-the-art methods. Particularly in the low-data regimes, RankMatch achieves significant improvements.
更多查看译文
关键词
Semantic Segmentation,Consistency Regularization,Semi-supervised Semantic Segmentation,Unlabeled Data,Self-supervised Learning,Network Cohesion,Generalization Performance Of The Model,Supervision Signal,Representative Agent,Deep Neural Network,Optimal Model,Feature Maps,Stochastic Gradient Descent,Critical Information,Kullback-Leibler,Pixel Level,Semi-supervised Learning,Teacher Network,Competitive Methods,Classical Setting,Student Network,Original Pixel,SOTA Methods,Semantic Segmentation Task,ResNet-101 Backbone,2nd Row,ResNet-50 Backbone,Pixel Features,Range Of Descriptors,Representation Learning
AI 理解论文
溯源树
样例
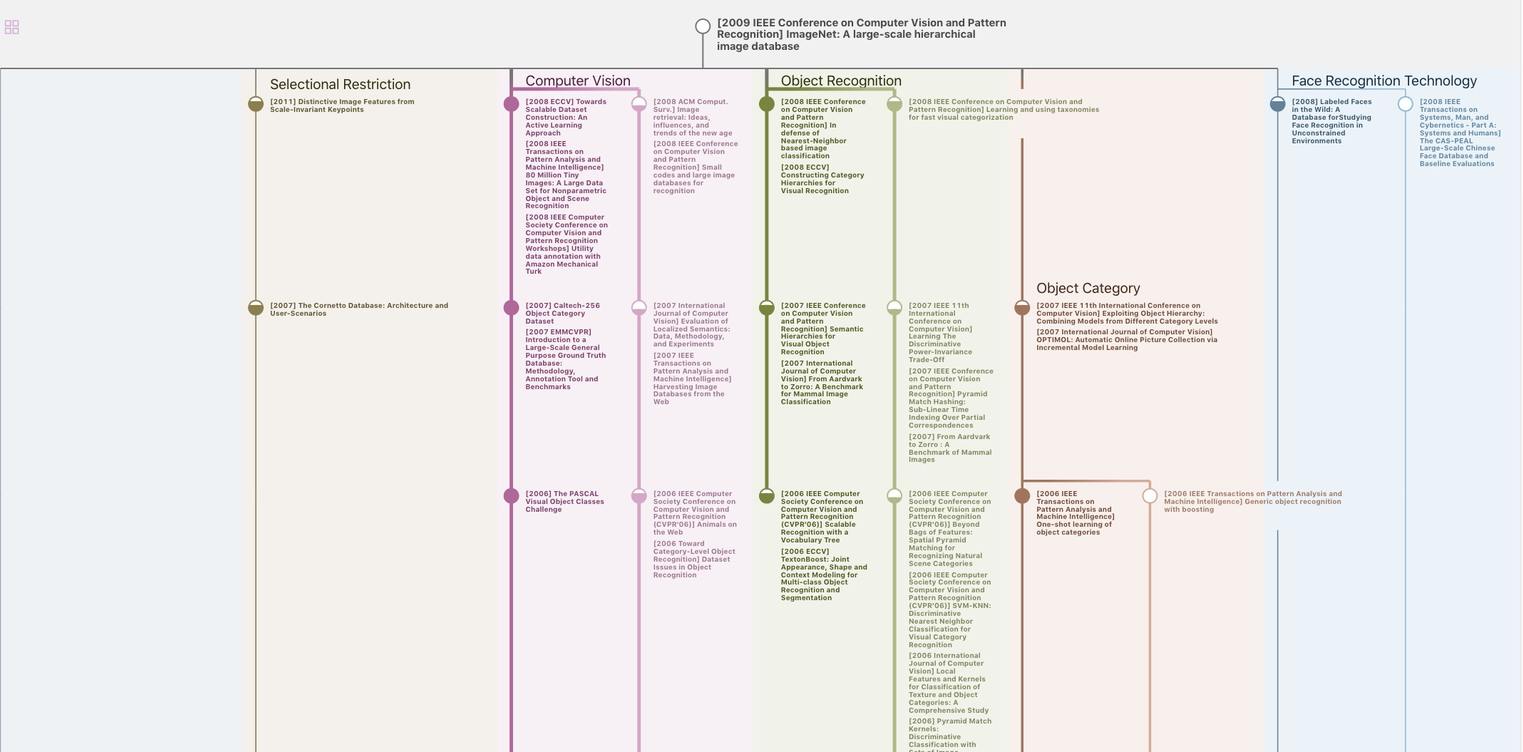
生成溯源树,研究论文发展脉络
Chat Paper
正在生成论文摘要