Neural Networks Based Behavioral Modeling of Dual-Band RF Power Amplifiers using Augmented BiLSTM Structures.
International Conference on Artificial Intelligence in Information and Communication(2024)
摘要
This paper introduces a novel Bidirectional Long-Short-Term-Memory (BiLSTM) based Artificial Neural Network (ANN) model for the behavioral modeling of the nonlinear distortions observed in dual-band radiofrequency power amplification systems. Addressing the demand for energy-efficient and green wireless infrastructures in the era of multi-band transmissions, the proposed Augmented BiLSTM uses selected features of the input signals in each band to concurrently predict the output waveform in each band. Experimental benchmarking against the established 2D-Memory Polynomial (2D-MP) model, conducted with a ZHL-5W-2G _ S+ power amplifier prototype driven by concurrent dual-band 5G signals, reveals that the proposed model is able to achieve comparable performance. In addition to its accuracy, the main advantage of the proposed neural network based model lies in its ability to simultaneously model the amplifier's behavior in both bands with a single trainable structure. This contrasts with conventional analytically defined models, which require a dedicated structure per signal transmission band.
更多查看译文
关键词
5G,artificial neural networks,behavioral modeling,distortions,memory effects,multi-band,power amplifiers
AI 理解论文
溯源树
样例
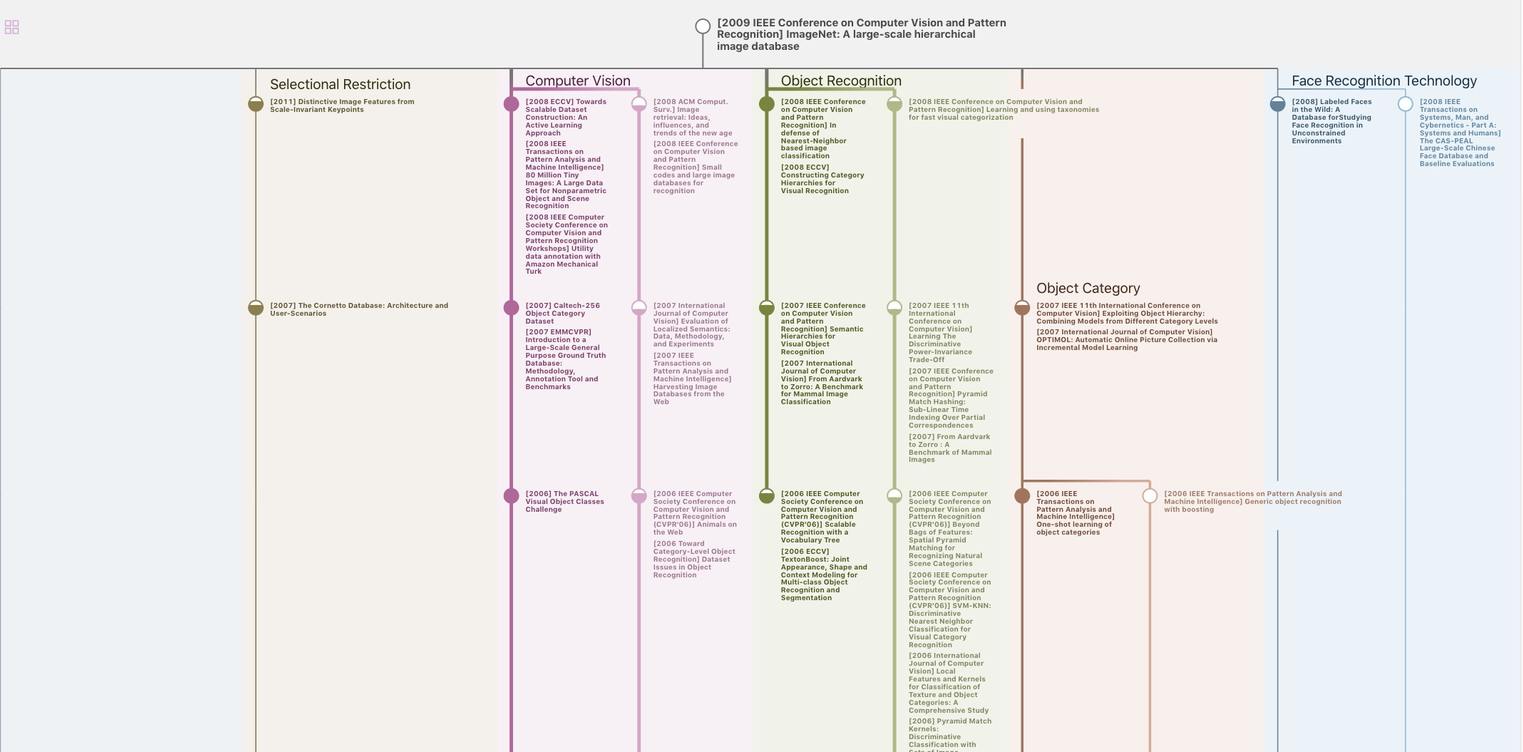
生成溯源树,研究论文发展脉络
Chat Paper
正在生成论文摘要