Efficient Machine Learning Model Focusing on Active Sites for the Discovery of Bifunctional Oxygen Electrocatalysts in Binary Alloys.
ACS applied materials & interfaces(2024)
摘要
The distinctive characteristics of alloy catalysts, encompassing composition, structure, and modifiable adsorption sites, present significant potential for the development of highly efficient electrocatalysts for oxygen evolution/reduction reactions [oxygen evolution reactions (OERs)/oxygen reduction reactions (ORRs)]. Machine learning (ML) methods can quickly establish the relationship between material features and catalytic activity, thus accelerating the development of alloy electrocatalysts. However, the current abundance of features presents a crucial challenge in selecting the most pertinent ones. In this study, we explored seven intrinsic features directly derived from the material's structure, with a specific focus on the chemical environment of active sites and their nearest neighbors. An accurate and efficient ML model to predict potential bifunctional oxygen electrocatalysts based on the intrinsic features of AB-type alloy active sites and intermediate free energies in the OERs/ORRs was established. These features possess clear physical and chemical meanings, closely linked to the electronic and geometric structures of active sites and neighboring atoms, thereby providing indispensable insights for the discovery of high-performance electrocatalysts. The ML model achieved R2 scores of 0.827, 0.913, and 0.711 for the predicted values of the three intermediate (OH, O, OOH) free energies, with corresponding mean absolute errors of 0.175, 0.242, and 0.200 eV, respectively. These results indicate that the ML model exhibits high accuracy in predicting the intermediate free energies. Furthermore, the ML model exhibited a prediction efficiency 150,000 times faster than traditional density functional theory calculations. This work will offer valuable insights and a framework for facilitating the rapid design of potential catalysts by ML methods.
更多查看译文
关键词
alloy catalysts,active sites,bifunctionaloxygen electrocatalyst,machine learning,intrinsicfeatures,density functional theory
AI 理解论文
溯源树
样例
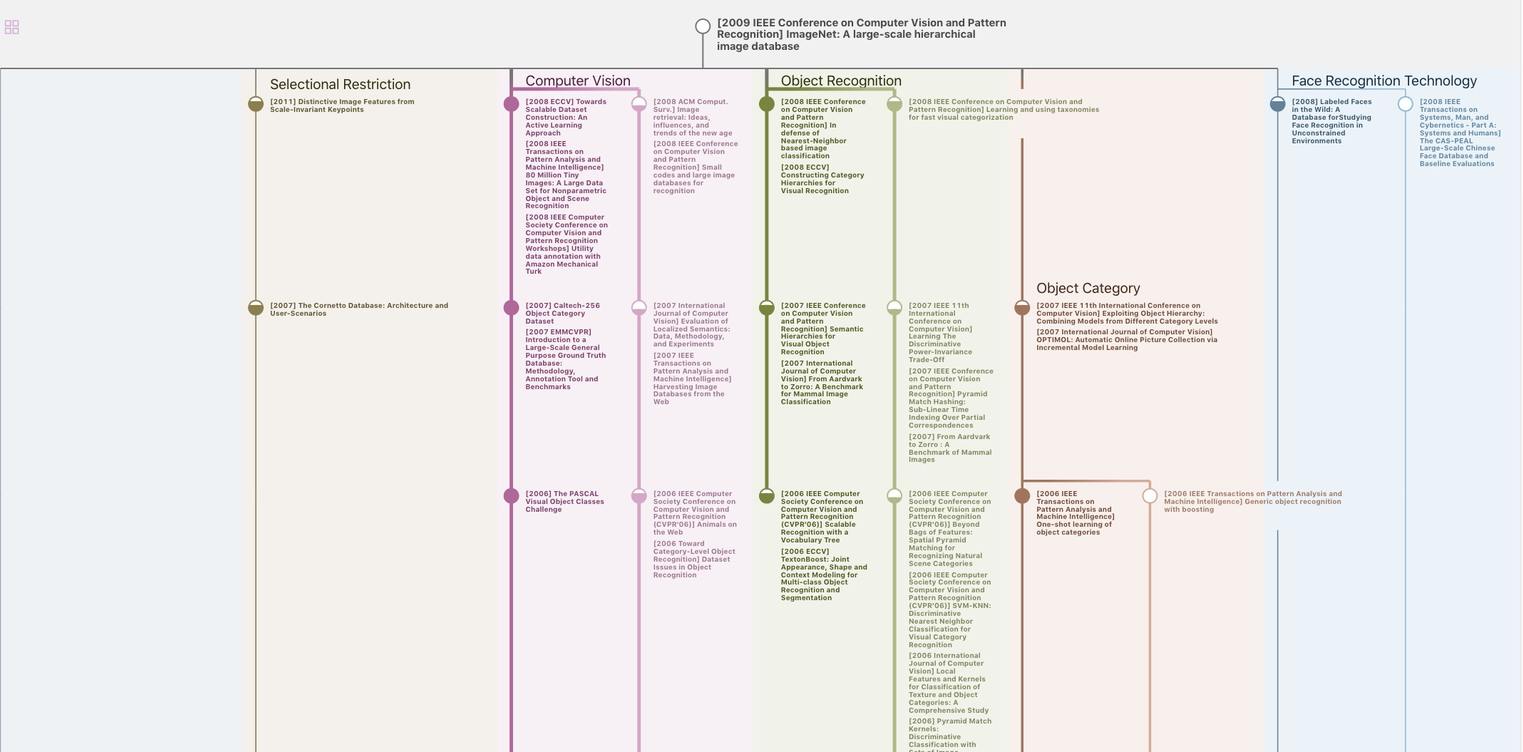
生成溯源树,研究论文发展脉络
Chat Paper
正在生成论文摘要