A Novel Hybrid Fingerprint-Based Indoor Localization Scheme with Hierarchical Training.
GLOBECOM (Workshops)(2023)
摘要
Due to its numerous applications, indoor localization is gaining relevance in industrial and general-purpose fields. Although the usage of machine learning-based methods has been proposed previously for indoor localization, most of the solutions provide generally unsatisfactory results when localization is performed with only one access point (AP) and Line-of-Sight (LoS) and Non-Line-of-Sight (NLoS) propagation conditions are combined. In order to account for this problem, a novel hybrid fingerprints-based indoor localization scheme with hierarchical training is proposed in this paper. Various types of representative large-scale and small-scale channel characteristics are used to define a novel hybrid fingerprint database, which allows for improving the localization performance. The proposed scheme is capable of: (i) achieving high localization accuracy when only one AP is used to transmit signals; (ii) performing LoSINLoS environmental sensing through the least-squares support vector classifiers (LS-SV C) model; and (iii) by using different methods to perform localization in the LoS and NLoS environments, enable optimal localization accuracy for each of them. More specifically, for the localization in NLoS environments, a hierarchical-SVC-support vector regressors (H-SVC-SVR) model is developed, based on hierarchical training. Simulation results are provided to prove that the proposed scheme exhibits superior advantages in localization accuracy compared to standard support vector machine (SVM)-based fingerprint indoor localization approaches. Furthermore, the method is proven to be robust to environmental changes, such as moving pedestrians. The designed approach represents a breakthrough in the field of machine learning-based indoor positioning methodologies.
更多查看译文
关键词
Indoor localization,LoSINLoS environmental sensing,hierarchical training,H -SV C-SVR,robustness
AI 理解论文
溯源树
样例
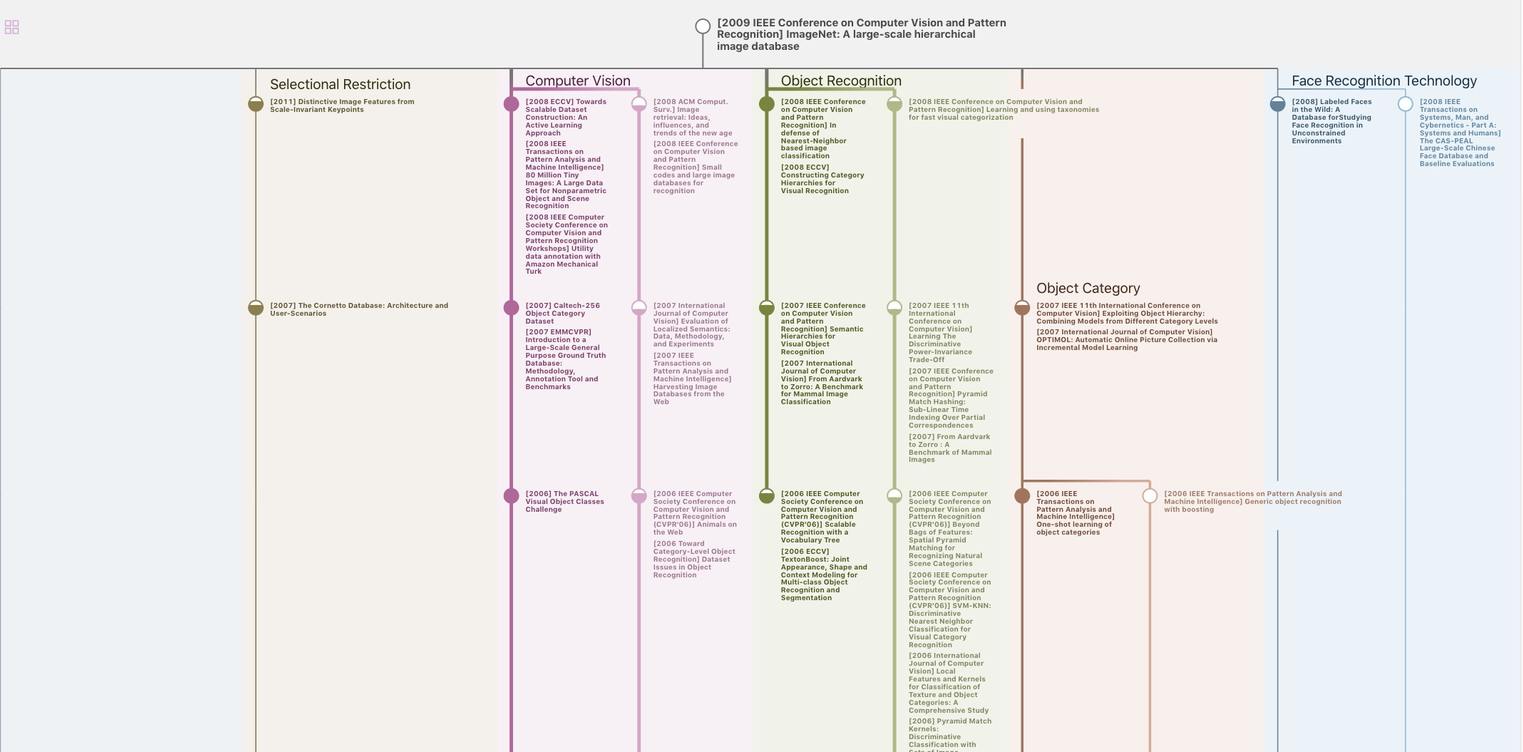
生成溯源树,研究论文发展脉络
Chat Paper
正在生成论文摘要