A Gradient Driven Graph Neural Network for Optimizing Precoding.
GLOBECOM (Workshops)(2023)
摘要
Precoder optimization for multi-user multi-antenna systems with the objective of sum rate maximization is a widely recognized challenging problem. Existing work has demonstrated the effectiveness of graph neural networks (GNNs) in learning the precoder, and various GNNs have been designed. In this paper, we propose a gradient driven GNN to learn the precoding policy. It incorporates two kinds of knowledge of optimizing precoder, namely the gradient of the sum rate with respect to the precoder and the permutation equivariant property of the precoding policy. By leveraging the knowledge, the proposed GNN employs an attention mechanism to learn the weights for information aggregation. Simulation results show that the proposed GNN can achieve the performance close to the traditional numerical algorithm and exhibit better generalization performance in terms of both users and antennas than baseline GNNs.
更多查看译文
关键词
GNN,gradient descent,precoding
AI 理解论文
溯源树
样例
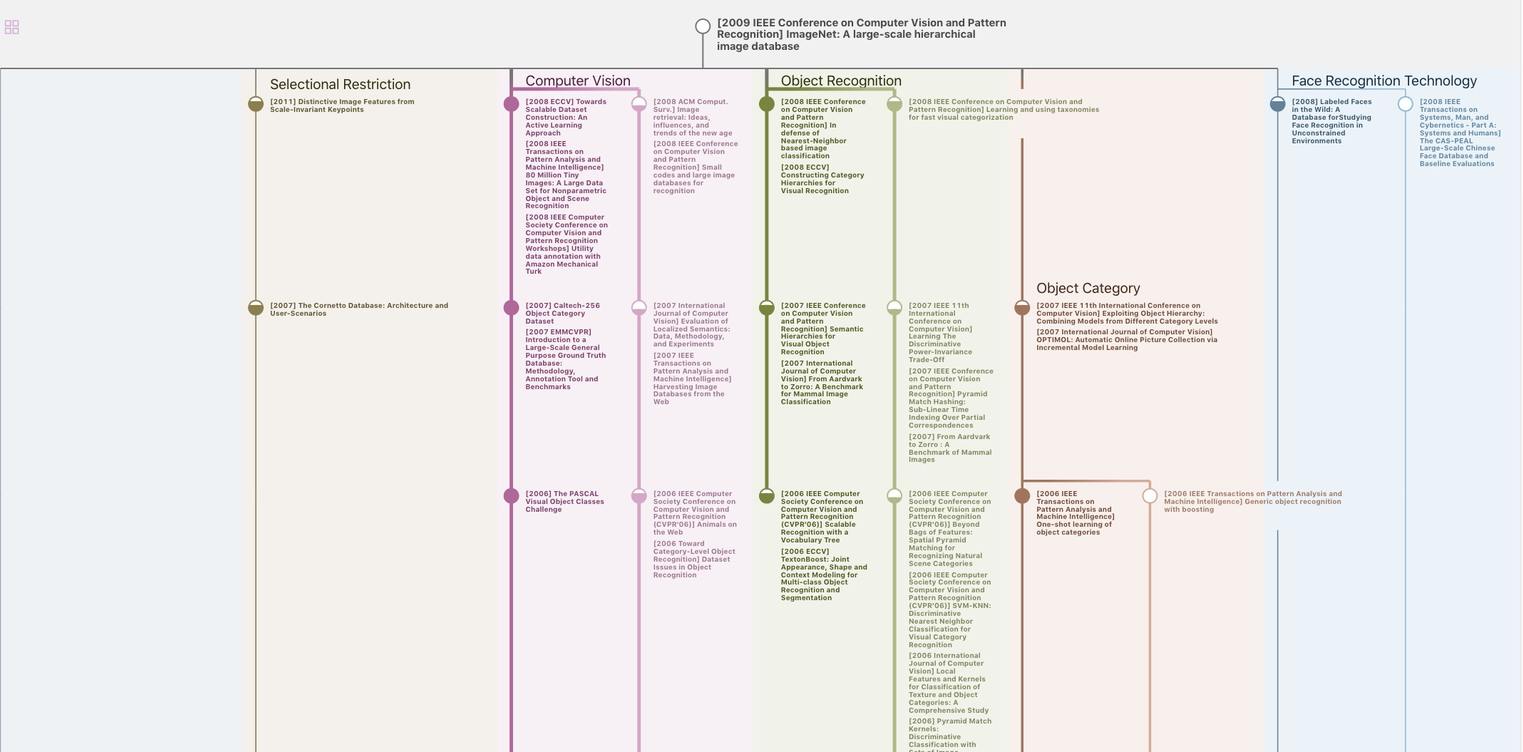
生成溯源树,研究论文发展脉络
Chat Paper
正在生成论文摘要