In-Memory Machine Learning Using Hybrid Decision Trees and Memristor Crossbars.
International Symposium on Smart Electronic Systems(2023)
摘要
We propose a method to design in-memory hybrid decision tree (HDT) circuits using memristor crossbars. Decision Trees (DTs) are a well known machine learning algorithm that carries multiple benefits when compared to deep neural networks. They are easily interpretable, fast and they require less data to train. These benefits make them a popular choice in wide-ranging applications that include edge devices and particle physics. We propose a HDT coupled with a multilayer perceptron (MLP) for creating a flexible nonlinear decision boundary which leads to better accuracy. Using this approach, we obtain a test accuracy of 0.90 for the MNIST dataset, which outperforms the state- of-the-art (SOTA). We map this decision tree onto crossbars which are purely memristor based. They utilize zero transistor and one memristor per cell and employ sneak-paths for flow- based in-memory computations. Due to the absence of transistors, our designs are radiation degradation resistant, serving their application in radiation-rich environments, and require less switching energy, making them energy efficient.
更多查看译文
关键词
In-memory computation,memristors,flow-based computing,decision trees,multivariate decision trees,multilayer perceptron
AI 理解论文
溯源树
样例
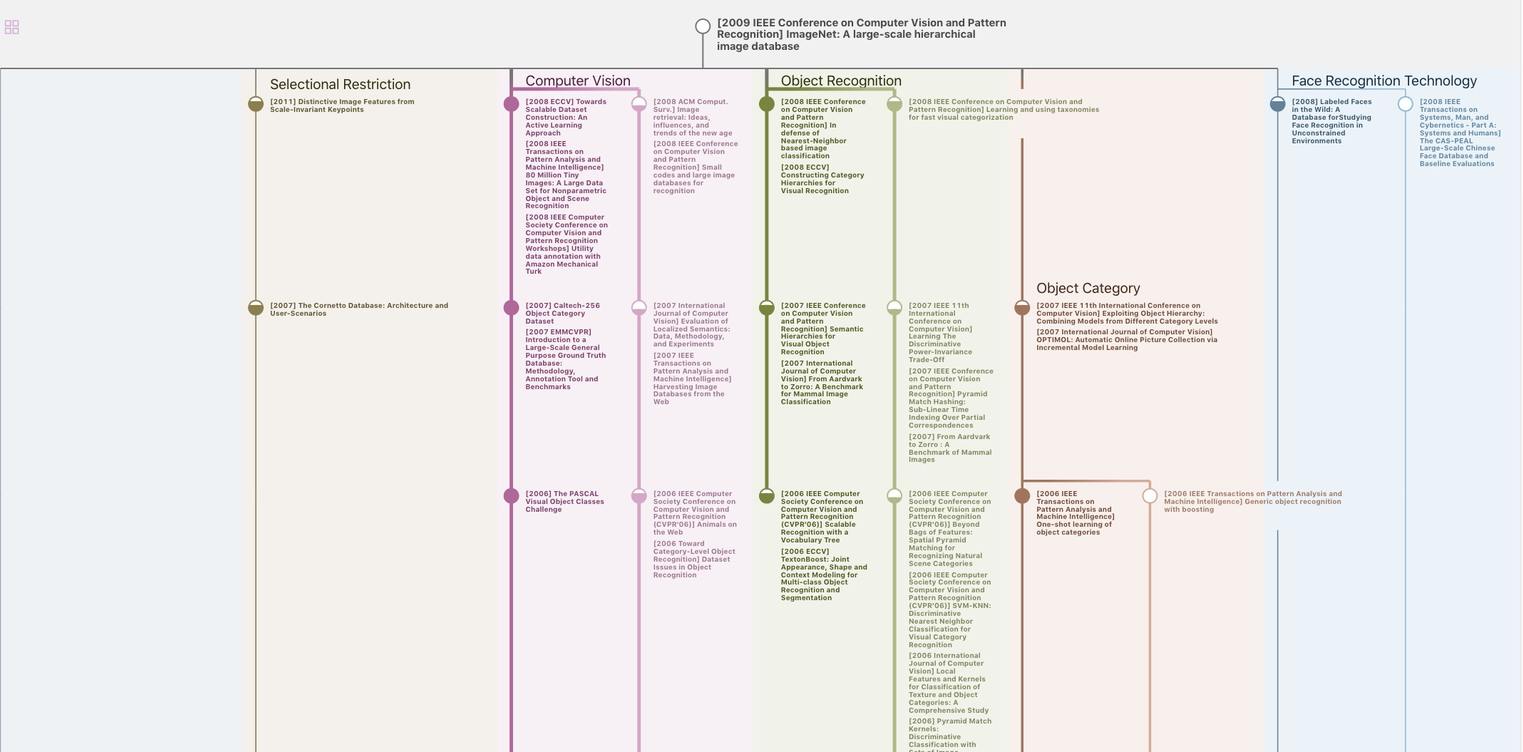
生成溯源树,研究论文发展脉络
Chat Paper
正在生成论文摘要