Efficient Learning Strategy for Predicting Glass Forming Ability in Imbalanced Datasets of Bulk Metallic Glasses
Physical Review Materials(2024)
摘要
The prediction of glass forming ability (GFA) and various properties in bulk
metallic glasses (BMGs) pose a challenge due to the unique disordered atomic
structure in this type of materials. Machine learning shows the potential
ability to find a way out. However, the training set from the experimental data
of BMGs faces the issue of data imbalance, including the distribution of data
related to elements, the range of performance data, and the distribution of
sparse and dense data area in each specific system. In this work, the origin of
the data imbalance and its impact on the GFA prediction ability of machine
learning models are analyzed. We propose the solutions by training the model
using the pruned dataset to mitigate the imbalance and by performing an active
experimental iterative learning to compensate for the information loss
resulting from data reduction. The strategy is proved in Zr-Al-Cu system, and
the automated workflow has been established. It effectively avoids the
prediction results from trapping into the intensive training data area or from
inducing by the data distribution of similar element systems. This approach
will expedite the development of new BMGs compositions especially for
unexplored systems.
更多查看译文
AI 理解论文
溯源树
样例
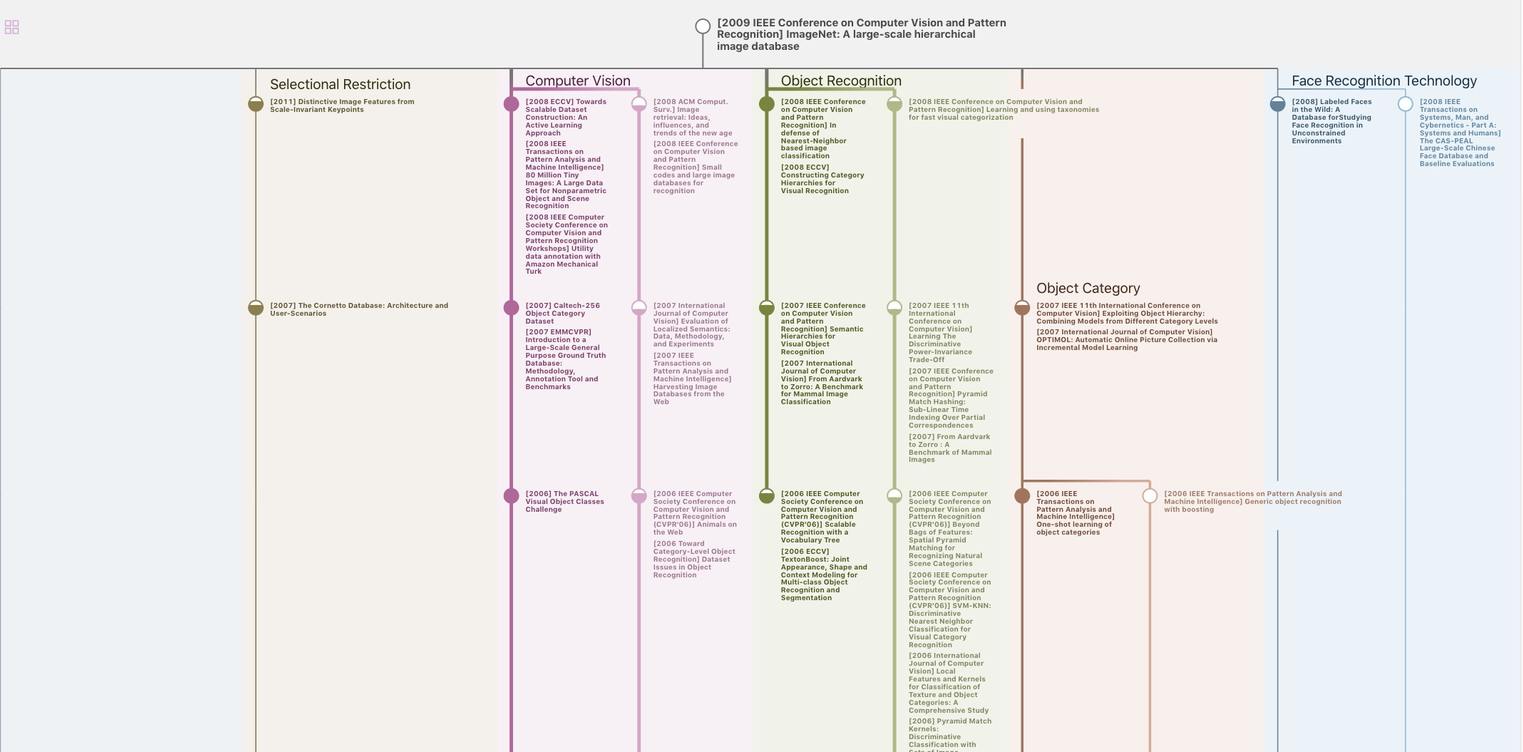
生成溯源树,研究论文发展脉络
Chat Paper
正在生成论文摘要