On the continuity and smoothness of the value function in reinforcement learning and optimal control
CoRR(2024)
摘要
The value function plays a crucial role as a measure for the cumulative
future reward an agent receives in both reinforcement learning and optimal
control. It is therefore of interest to study how similar the values of
neighboring states are, i.e., to investigate the continuity of the value
function. We do so by providing and verifying upper bounds on the value
function's modulus of continuity. Additionally, we show that the value function
is always Hölder continuous under relatively weak assumptions on the
underlying system and that non-differentiable value functions can be made
differentiable by slightly "disturbing" the system.
更多查看译文
AI 理解论文
溯源树
样例
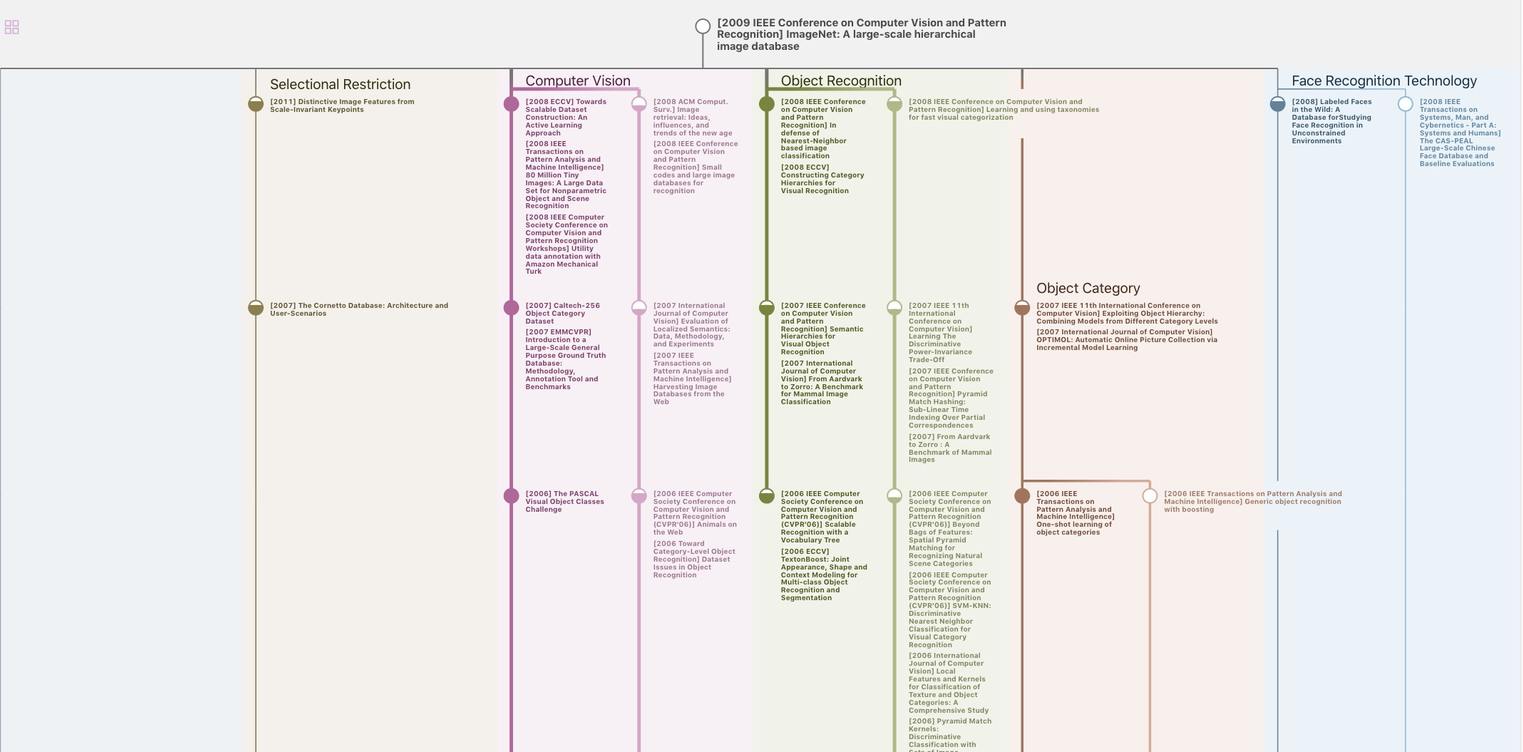
生成溯源树,研究论文发展脉络
Chat Paper
正在生成论文摘要