Redefining Tea Cultivation: A Federated Approach to Detecting Leaf Diseases with CNN
2024 Fourth International Conference on Advances in Electrical, Computing, Communication and Sustainable Technologies (ICAECT)(2024)
摘要
Tea leaf diseases have become a significant problem in the vast field of agricultural research, calling for sophisticated diagnostic methods. This research takes a cutting-edge approach, carefully classifying tea leaf illnesses using the power of Federated Learning combined with Convolutional Neural Networks (CNN). This research project included records from six customers, each representing four levels of illness severity. The research eliminated the requirement for centralized data aggregation by using the decentralized architecture of federated learning to permit collaborative but privacy-preserving data analysis. The Federated Averaging CNN Result Analysis reveals fascinating results. The Macro averages varied from a spectacular 88.66% (Z_2) to 93.35% (Z_4). Similar patterns emerged from weighted averages, with the zenith recorded at 93.46% (Z_4) and the nadir at 89.57% (Z_2). The weighted average numbers for customers like Z_4 and Z_5, which came in at 93.46% and 92.67%, were mirrored by the Micro averages, reflecting a holistic performance view. Such uniformity across several criteria highlights the model's flexibility and resilience. In conclusion, this landmark study clarifies the enormous potential of CNNs and Federated Learning to revolutionize the detection of tea leaf illness. The consistently high statistical results across various clients and severity levels herald a new era in precision agriculture, promising improved disease control and, consequently, healthier yields.
更多查看译文
关键词
Tea leaf diseases,Convolutional neural networks (CNNs),Federated learning,Disease classification,Severity levels
AI 理解论文
溯源树
样例
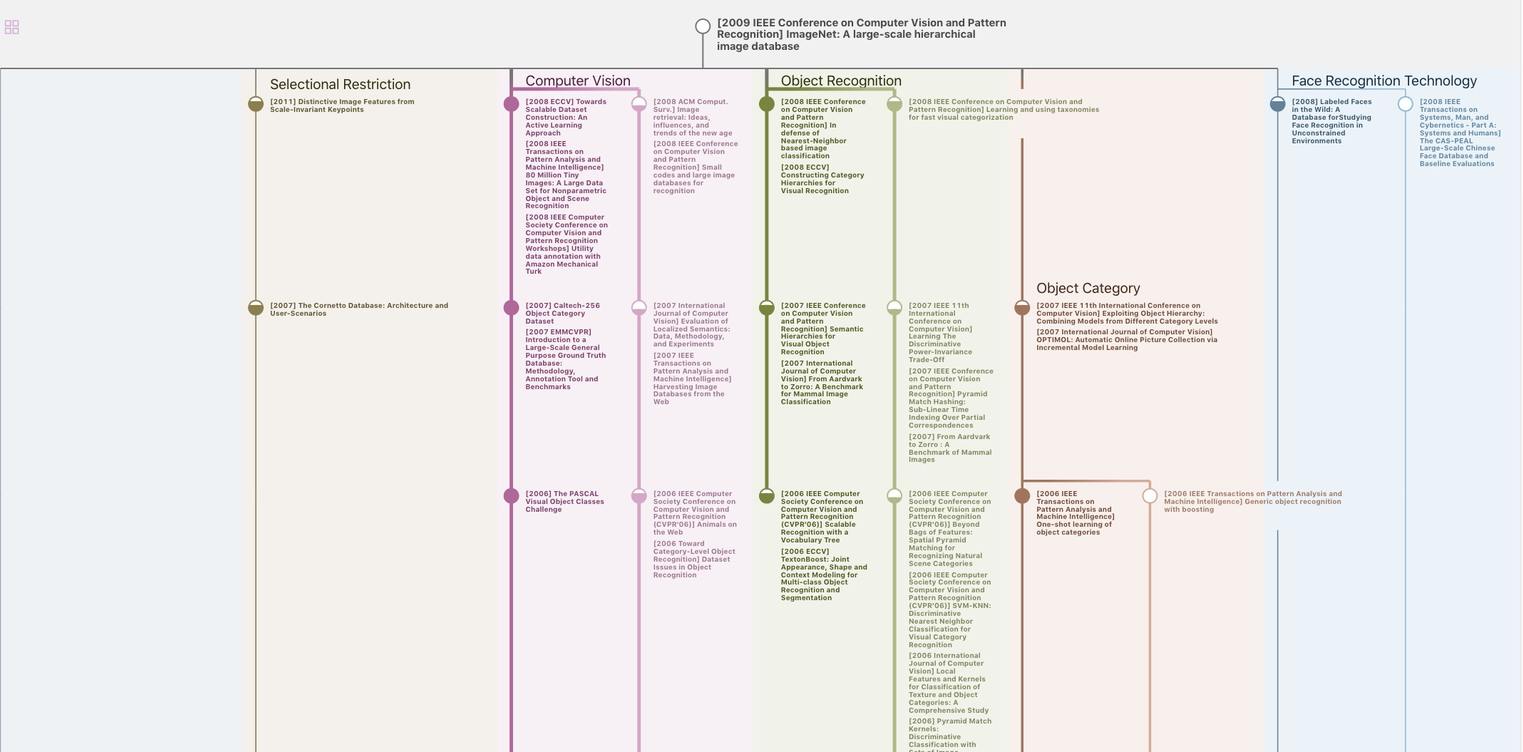
生成溯源树,研究论文发展脉络
Chat Paper
正在生成论文摘要