Class similarity weighted knowledge distillation for few shot incremental learning
Neurocomputing(2024)
摘要
Few-shot class incremental learning illustrates the challenges of learning new concepts, where the learner can access only a small sample per concept. The standard incremental learning techniques cannot be applied directly because of the small number of samples for training. Moreover, catastrophic forgetting is the propensity of an Artificial Neural Network to fully and abruptly forget previously learned knowledge upon learning new knowledge. This problem happens due to a lack of supervision in older classes or an imbalance between the old and new classes. In this work, we propose a new distillation structure to tackle the forgetting and overfitting issues. Particularly, we suggest a dual distillation module that adaptably draws knowledge from two different but complementary teachers. The first teacher is the base model, which has been trained on large class data, and the second teacher is the updated model from the previous K-1 session, which contains the modified knowledge of previously observed new classes. Thus, the first teacher can reduce overfitting issues by transferring the knowledge obtained from the base classes to the new classes. While the second teacher can reduce knowledge forgetting by distilling knowledge from the previous model. Additionally, we use semantic information as word embedding to facilitate the distillation process. To align visual and semantic vectors, we used the attention mechanism of the embedding of visual data. With extensive experiments on different data sets such as Mini-ImageNet, CIFAR100, and CUB200, our model shows state-of-the-art performance compared to the existing few shot incremental learning methods.
更多查看译文
关键词
Knowledge distillation,Semantic information,Few shot,Incremental learning
AI 理解论文
溯源树
样例
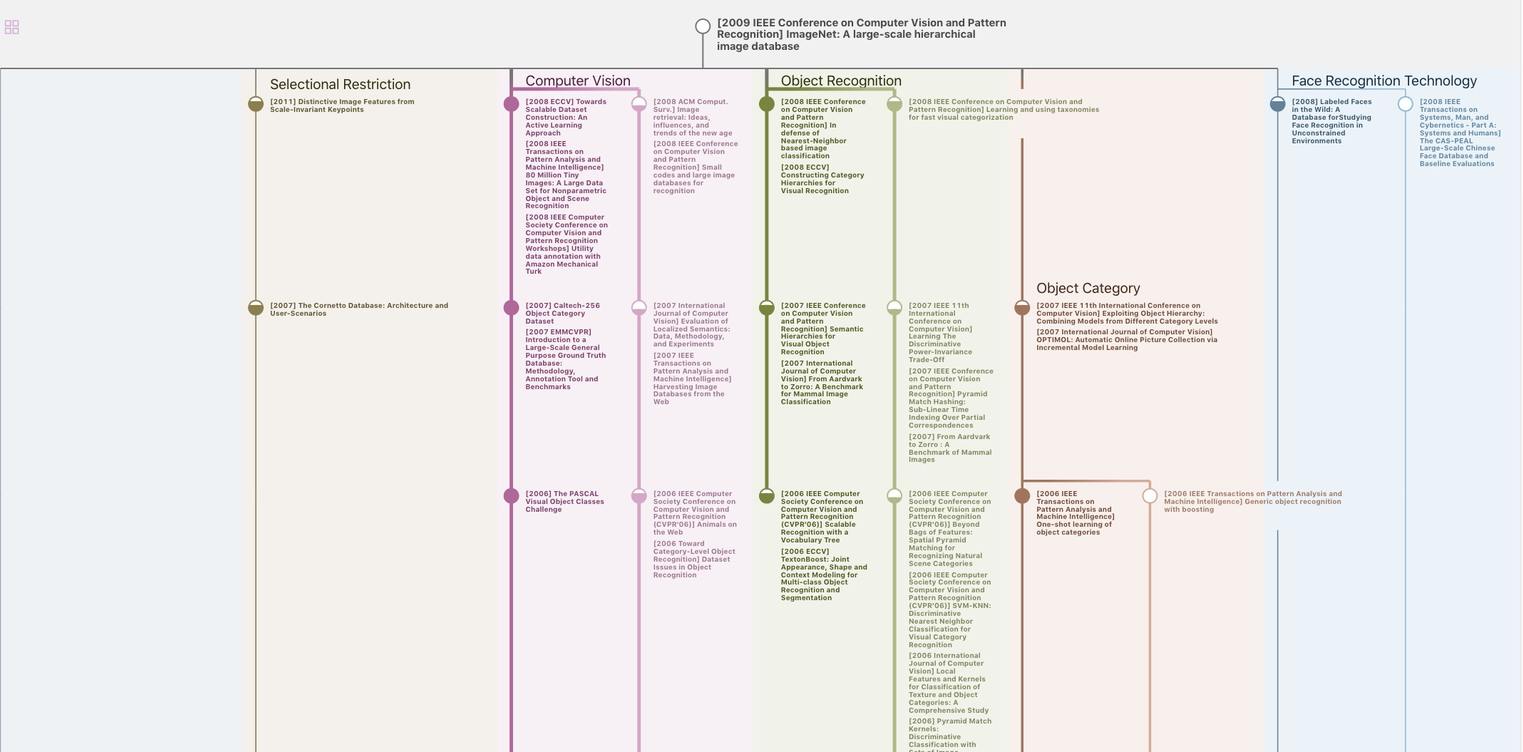
生成溯源树,研究论文发展脉络
Chat Paper
正在生成论文摘要