Spatial-Spectral Similarity Based on Adaptive Region for Landslide Inventory Mapping With Remote-Sensed Images.
IEEE Trans. Geosci. Remote. Sens.(2024)
摘要
Landslide is one of the most serious geological disasters around the world, and acquiring landslide inventory mapping (LIM) with remote sensed images (RSIs) plays an important role in disaster relief. However, various external imaging conditions of bitemporal RSIs usually cause pseudo-changes and challenges for achieving satisfied LIMs. In this article, a pioneering change magnitude measured distance named Spectral-Spatial Similarity based on Adaptive Region (S
3
AR) is proposed for achieving LIMs with bitemporal RSIs. First, an adaptive region is proposed to utilize the spatial-contextual information around each pixel, because the shapes and size of a landslide site are usually irregular and unpredictable. Then, a shape description algorithm is proposed for constructing a shape description vector, which aims at measuring the spatial difference of adaptive regions. Finally, to improve the separability between the landslide area and the background, brightness is suggested to couple with the shape description vector of an adaptive region to generate spatial-spectral similarity to measure the change magnitude between pairwise adaptive regions from the bitemporal RSIs. When the entire bitemporal RSIs are scanned and calculated via these steps, a change magnitude image between bitemporal RSIs can be generated, and then binary LIMs are obtained by a binary threshold. Experiments based on comparing eight state-of-the-art approaches demonstrated the feasibility and superiorities of the proposed S3AR for achieving LIMs with bitemporal RSIs. For example, the improvements on the four datasets are 5.81%, 14.06%, 6.03%, and 20.51% in terms of total error.
更多查看译文
关键词
adaptive region,landslide inventory mapping,spectral-spatial similarity,remote sensing images
AI 理解论文
溯源树
样例
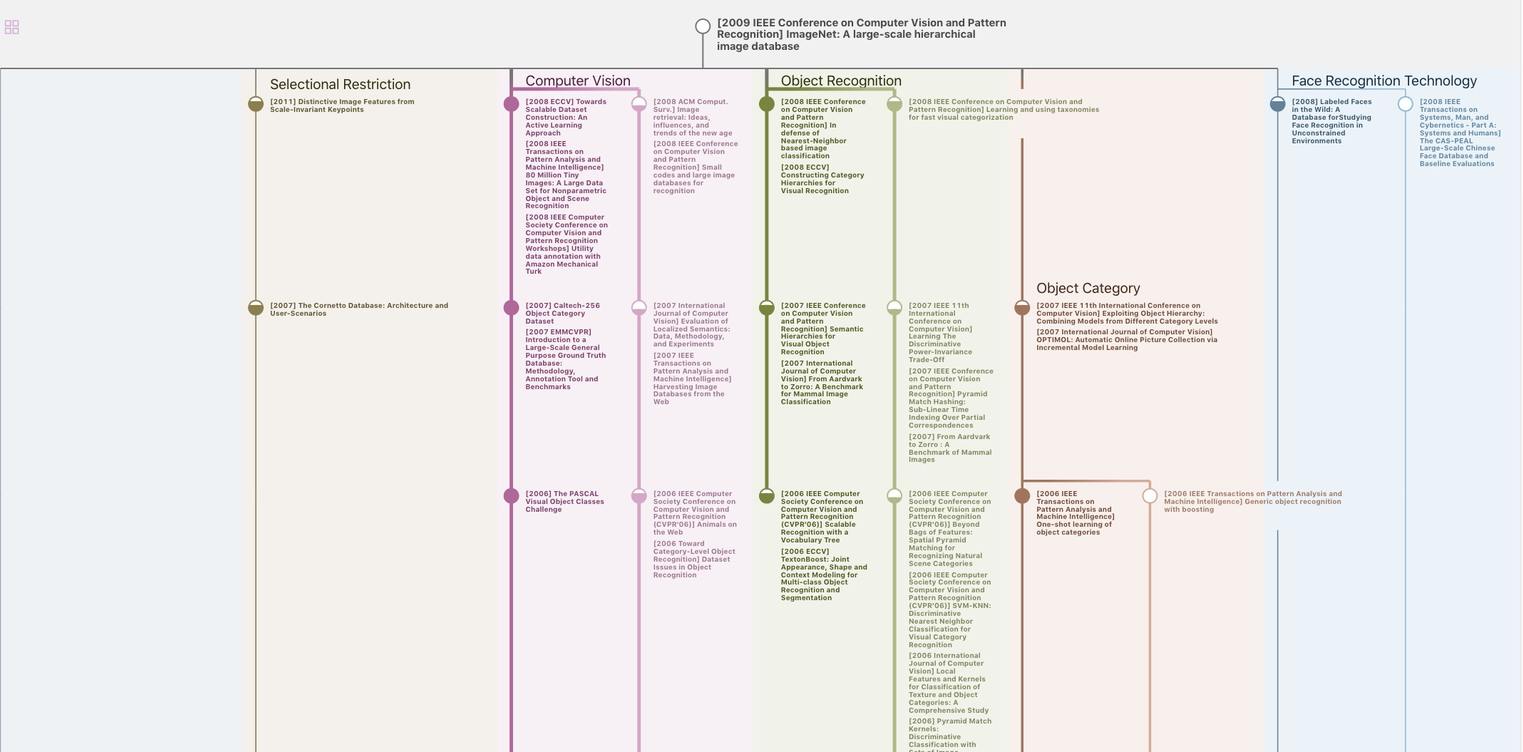
生成溯源树,研究论文发展脉络
Chat Paper
正在生成论文摘要