TADNet: Temporal Attention Decomposition Networks for Probabilistic Energy Forecasting
IEEE Transactions on Power Systems(2024)
摘要
Many decision-making processes in the energy industry depend on probabilistic forecasting to quantify the future uncertainties. However, existing methods are difficult to provide reliable probabilistic forecasting for the energy industry. Hence, we focus on solving the multi-horizon probabilistic forecasting problem of energy time series. In this paper, the time series is considered as the superposition of a regular changing component and a residual component. On this basis, we develop a novel deep learning-based temporal attention decomposition network (TADNet). TADNet predicts the regular change of the time series, and then quantifies the fluctuation intervals of the residual component, which enables TADNet to achieve reliable probabilistic forecasting. To take both short- and long-term patterns into account, TADNet combines convolution with attention mechanism to extract the temporal feature from the time series. To enable efficient information extraction, the gating components are utilized to suppress unnecessary features. Through extensive empirical evaluation of several real-world energy datasets, TADNet achieves better prediction accuracy than the compared models.
更多查看译文
关键词
Deep learning,Neural networks,Attention mechanism,Time series forecasting,Probabilistic energy forecasting
AI 理解论文
溯源树
样例
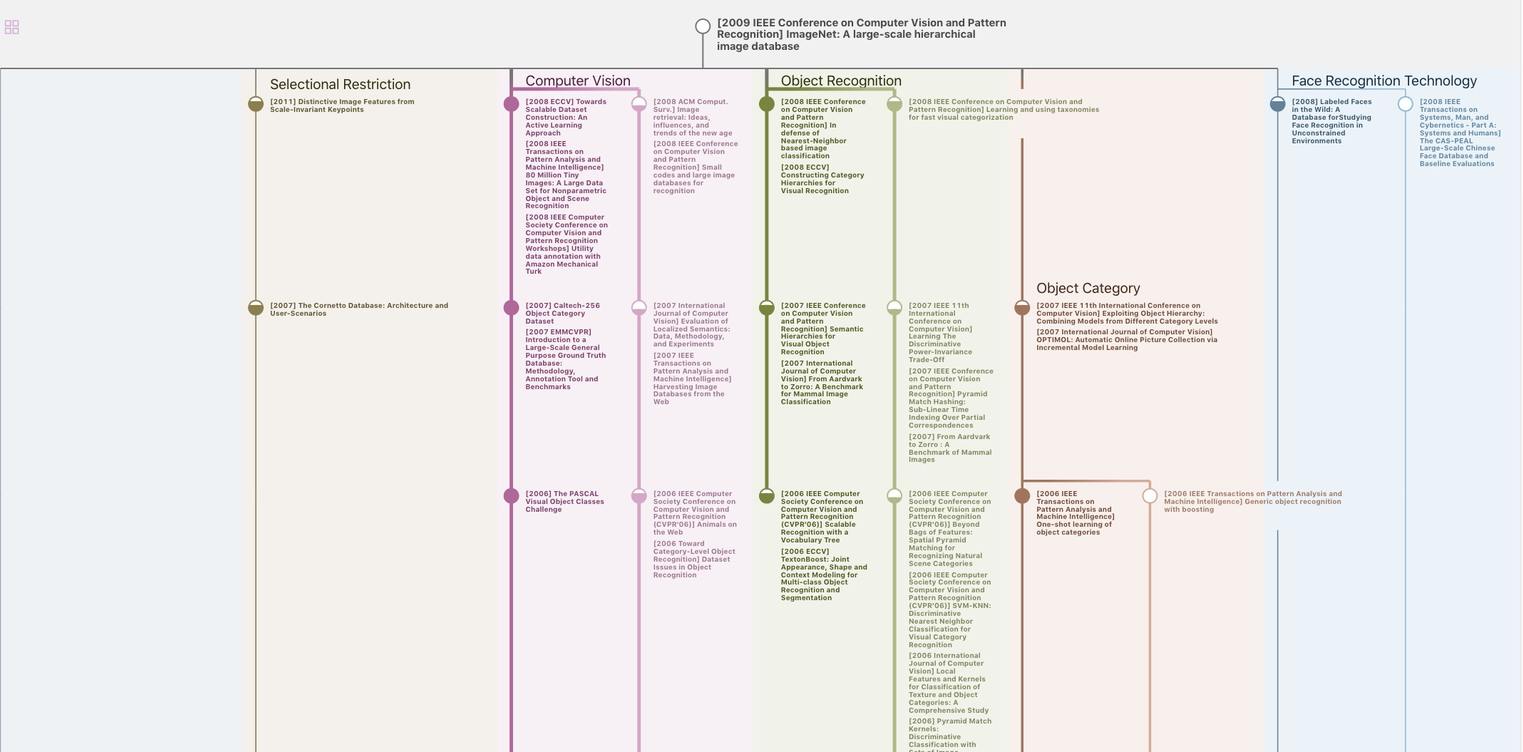
生成溯源树,研究论文发展脉络
Chat Paper
正在生成论文摘要