A machine-learning method for biobank-scale genetic prediction of blood group antigens
PLOS COMPUTATIONAL BIOLOGY(2024)
摘要
A key element for successful blood transfusion is compatibility of the patient and donor red blood cell (RBC) antigens. Precise antigen matching reduces the risk for immunization and other adverse transfusion outcomes. RBC antigens are encoded by specific genes, which allows developing computational methods for determining antigens from genomic data. We describe here a classification method for determining RBC antigens from genotyping array data. Random forest models for 39 RBC antigens in 14 blood group systems and for human platelet antigen (HPA)-1 were trained and tested using genotype and RBC antigen and HPA-1 typing data available for 1,192 blood donors in the Finnish Blood Service Biobank. The algorithm and models were further evaluated using a validation cohort of 111,667 Danish blood donors. In the Finnish test data set, the median (interquartile range [IQR]) balanced accuracy for 39 models was 99.9 (98.9-100)%. We were able to replicate 34 out of 39 Finnish models in the Danish cohort and the median (IQR) balanced accuracy for classifications was 97.1 (90.1-99.4)%. When applying models trained with the Danish cohort, the median (IQR) balanced accuracy for the 40 Danish models in the Danish test data set was 99.3 (95.1-99.8)%. The RBC antigen and HPA-1 prediction models demonstrated high overall accuracies suitable for probabilistic determination of blood groups and HPA-1 at biobank-scale. Furthermore, population-specific training cohort increased the accuracies of the models. This stand-alone and freely available method is applicable for research and screening for antigen-negative blood donors. Blood transfusion is one of the most common clinical procedures in the hospitals and the key element for safe transfusion is compatibility between the recipient and donor red blood cell antigens. Precise antigen matching reduces the risk for sensitization and other adverse transfusion outcomes. Here we describe a stand-alone and freely available random forest classification method and models for determining red blood cell and human platelet antigens from array technology-based genotyping data. We investigate the performance of models trained with Finnish blood donor biobank data and further validate the method with a large Danish cohort. The results demonstrate high overall accuracy, and the method is suitable for biobank-scale research and screening of antigen-negative donors. The implementation is possible in the local computing environment without sensitive data uploads and requires only a moderate level of bioinformatic skills.
更多查看译文
AI 理解论文
溯源树
样例
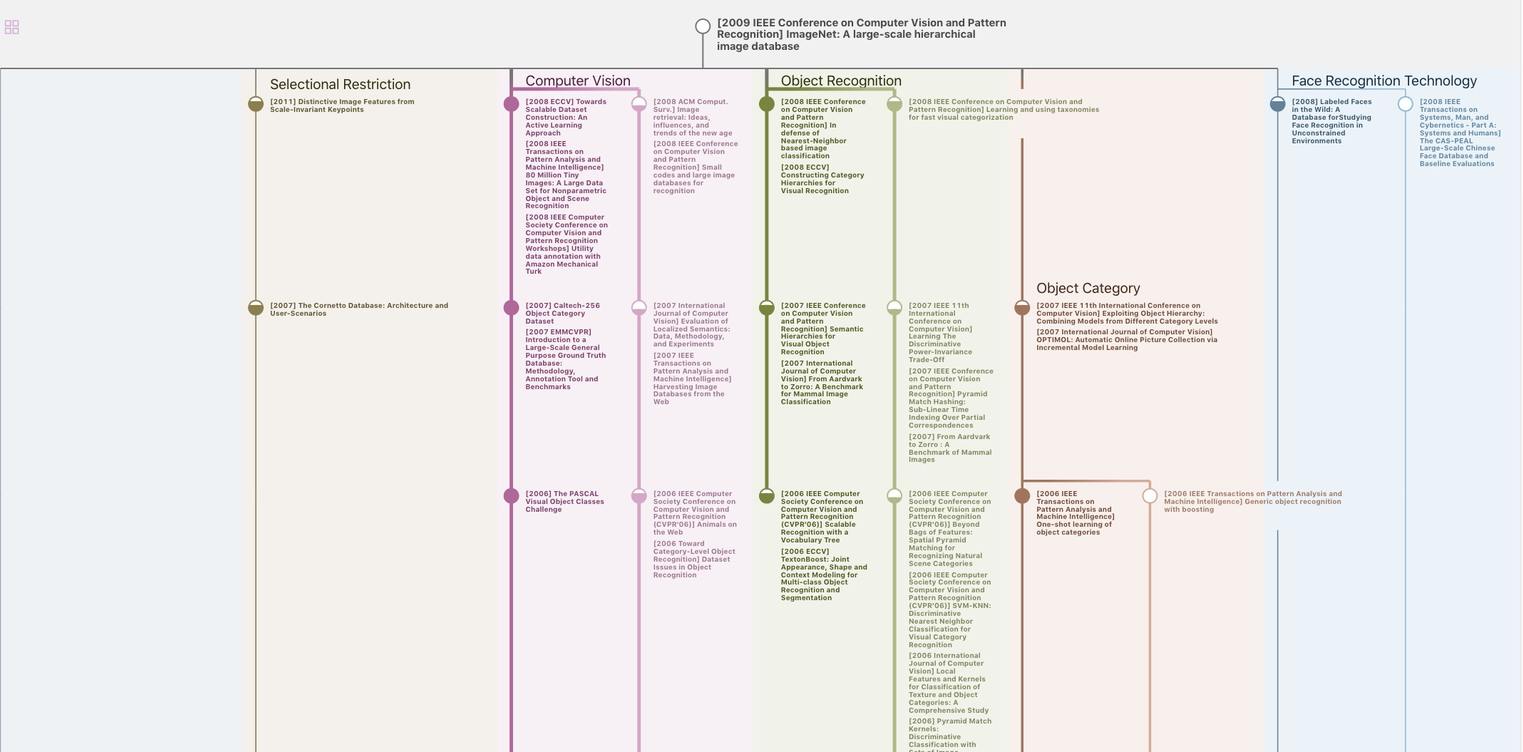
生成溯源树,研究论文发展脉络
Chat Paper
正在生成论文摘要