STP: Self-supervised transfer learning based on transformer for noninvasive blood pressure estimation using photoplethysmography
Expert Systems with Applications(2024)
摘要
Non-invasive blood pressure (BP) monitoring plays a crucial role in cardiovascular disease prevention, but traditional cuff-based methods lack continuous monitoring capability. Photoplethysmography (PPG) offers a promising alternative by capturing blood volume changes optically. The challenge of effectively capturing fine-grained discriminative features of BP using limited paired signals persists, despite the benefits offered by deep models trained on extensive data. The objective of this study is to create a Transformer framework for continuous monitoring of noninvasive BP through self-supervised transfer learning and BP pattern adaptation. We developed a data preprocessing strategy that integrates eleven physiological signal transformations to perform unsupervised pseudo-labels for PPG signals. Then, we developed a self-supervised learning network based on the Transformer architecture to extract robust signal representations from transformed PPGs during the pretraining phase. Furthermore, we designed a transfer learning approach that incorporates BP pattern adaptation to derive discriminative features for accurate estimation of BP values. The proposed STP model was evaluated on multi-source datasets containing 1,213 subjects based on a subject-wise paradigm. The clinical standard was met with estimation errors of 0.85 ± 4.21 mmHg and 0.49 ± 2.76 mmHg for systolic BP and diastolic BP, respectively. These results indicate that the STP model performs competitively in terms of BP estimation when compared to current state-of-the-art approaches. Our study presents a novel STP approach for continuous monitoring of noninvasive BP using photoplethysmography signals. By integrating BP pattern adaptation, our approach achieves a competitive performance in estimating BP values, demonstrating its potential for clinical applications.
更多查看译文
关键词
Blood pressure monitoring,Photoplethysmography,Self-supervised learning,Transfer learning,Domain adaptive learning
AI 理解论文
溯源树
样例
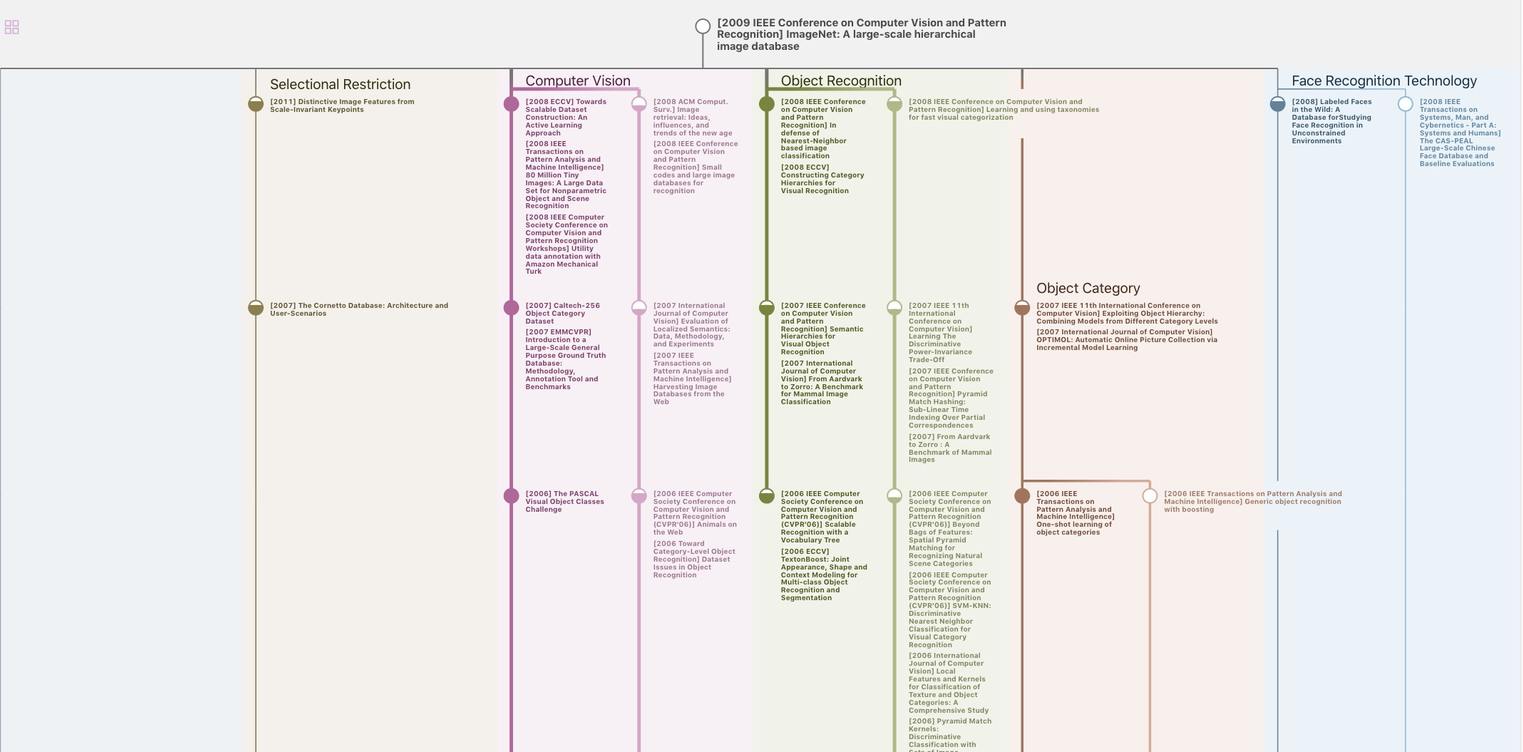
生成溯源树,研究论文发展脉络
Chat Paper
正在生成论文摘要