Random Clustering-based Outlier Detector
Information Sciences(2024)
摘要
Outlier detection is one of the most important issues in contemporary data analysis. At present, many methods are employed for anomaly and outlier detection, but there is still no universal tool that delivers a high degree of efficiency. In this study, we present a novel approach for outlier detection based on the skillful use of the law of large numbers. The main idea of the proposed solution consists of the random clustering of the elements of the analyzed set. Then, those elements that are sufficiently distant from the random cluster centers are marked as outliers. The proposed approach, besides being highly effective, is also very intuitive. The results of the conducted numerical experiments confirm the high degree of effectiveness of the proposed method, with the measures of accuracy and precision reaching a value of 1. The indisputable advantages of this novel approach for outlier detection are the simplicity of interpretation and the possibility of its modification by people who may lack an extensive experience in data analysis. The effectiveness of the proposed method was compared with other recognized techniques in detecting outliers within both artificially generated and empirical data sets.
更多查看译文
关键词
outlier detection,clustering,random clustering,number of clusters,metric,law of large numbers
AI 理解论文
溯源树
样例
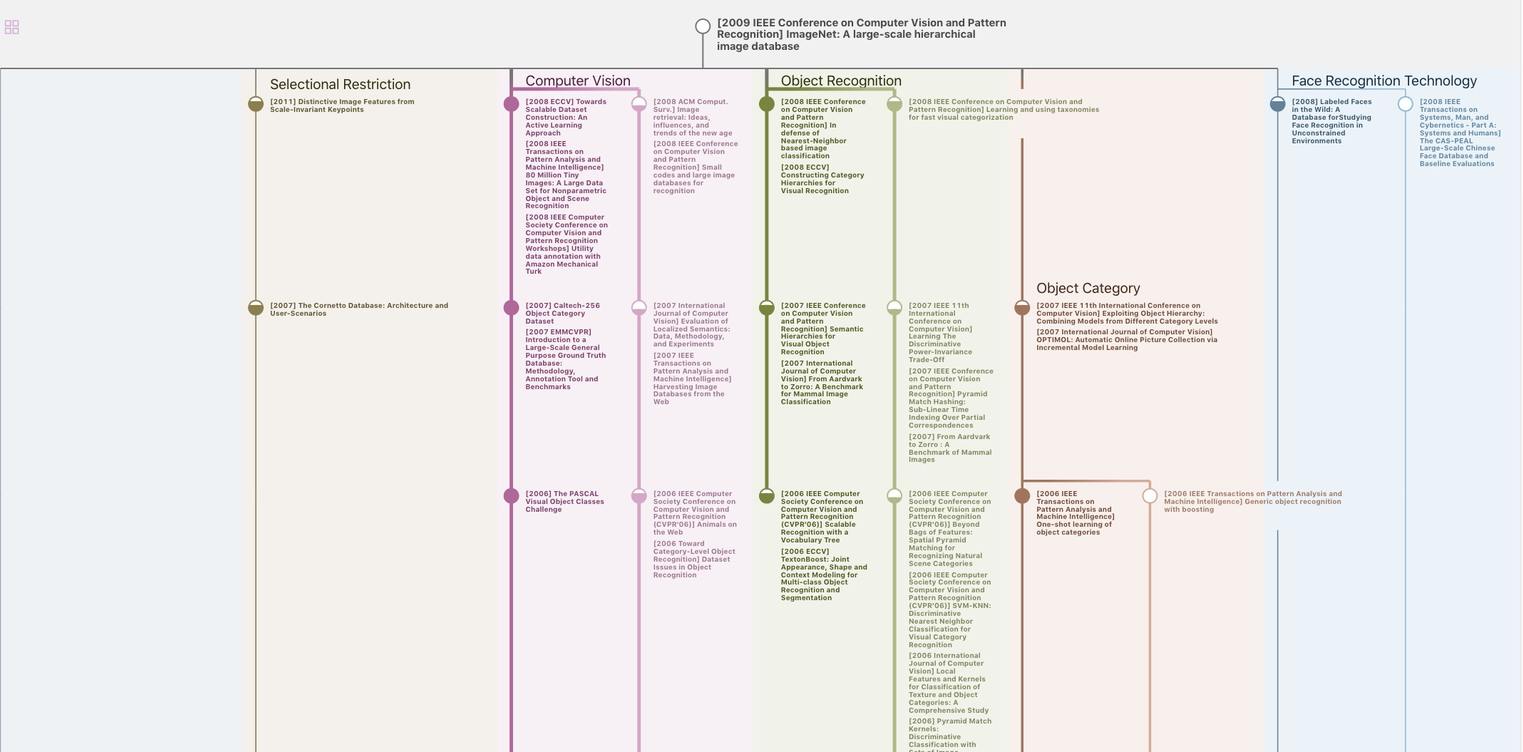
生成溯源树,研究论文发展脉络
Chat Paper
正在生成论文摘要