Fault diagnosis of lithium-ion batteries based on wavelet packet decomposition and Manhattan average distance
INTERNATIONAL JOURNAL OF GREEN ENERGY(2024)
摘要
As lithium-ion batteries are widely used in electric vehicles, safety accidents caused by battery failures emerge one after another. Nevertheless, failures caused by changes in the internal structure or characteristics of the battery, such as sudden and progressive failures, are still a serious problem for electric vehicles, challenging existing fault diagnosis methods. This paper first performs wavelet packet decomposition on the battery's raw voltage signal to obtain high-quality low-frequency and high-frequency characteristic signal components. Then performs singular value decomposition on the characteristic signal components to extract the corresponding singular value characteristic parameters, and introduces the Manhattan average distance algorithm to battery faults. Diagnosing and locating faulty battery units using the Laida criterion (3-sigma criterion) outlier detection method. Finally, actual vehicle data were used to verify the reliability, stability, accuracy of the method, and compared with the traditional Manhattan distance, correlation coefficient, information entropy methods. The method in this paper has good fault detection effects on vehicles with sudden and progressive faults vehicles.
更多查看译文
关键词
Lithium-ion batteries,sudden failures,progressive failures,actual vehicle data,fault diagnosis
AI 理解论文
溯源树
样例
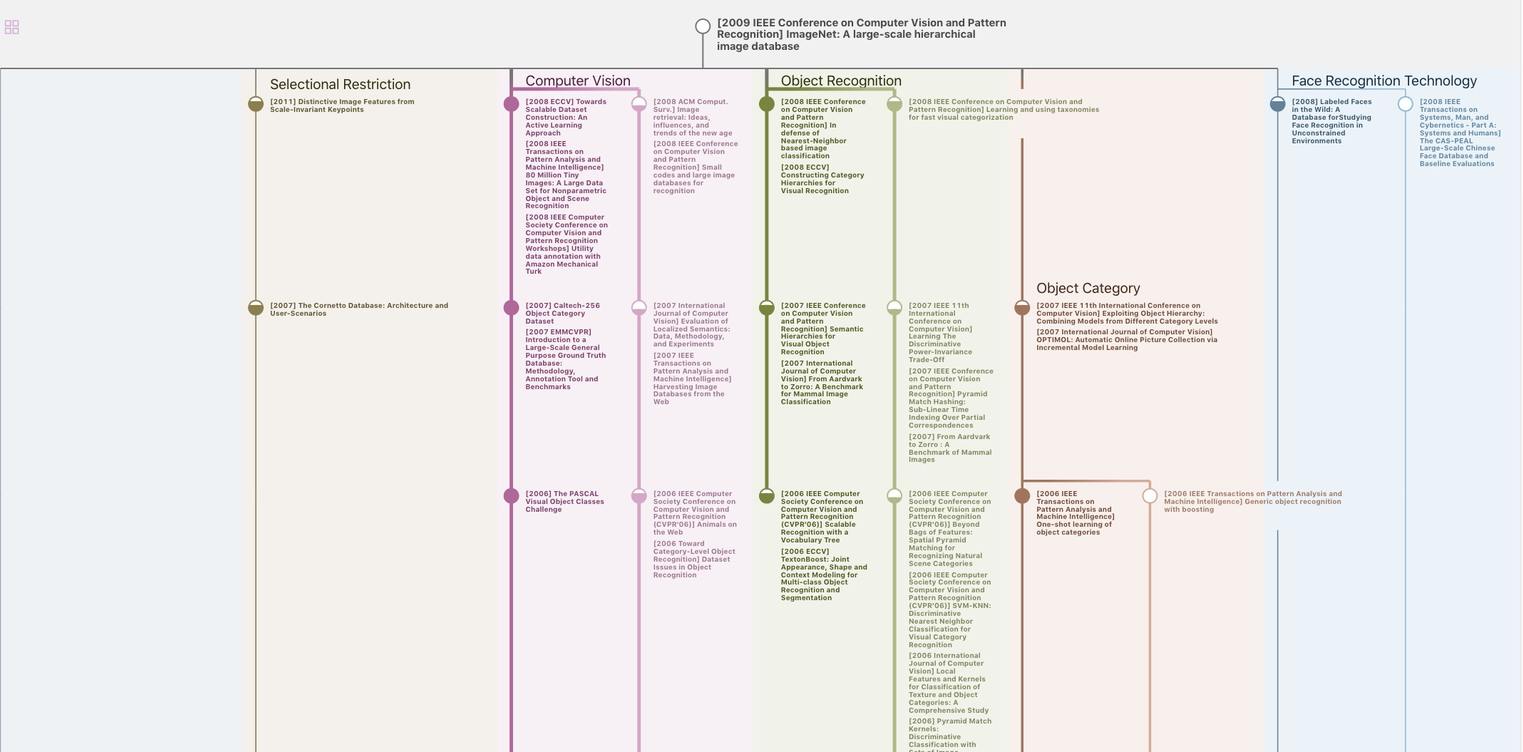
生成溯源树,研究论文发展脉络
Chat Paper
正在生成论文摘要