A semi-supervised learning method based on pseudo-label iterative purification for intelligent fault diagnosis of rolling bearing
MEASUREMENT SCIENCE AND TECHNOLOGY(2024)
摘要
Bearing fault diagnosis based on semi-supervised learning shows good results in solving the scarcity of data in industrial scenarios. Pseudo-label samples can be used to expand labeled datasets, thereby to solve the shortage of training samples. However, when pseudo-labeling is employed to improve the performance of the model, the low confidence level of pseudo-labels will have a negative impact on the model. To solve this problem, the pseudo-label iterative purification method is proposed. Firstly, the graph neural network is employed to aggregate samples in order to increase the degree of dissimilarity among target domain data belonging to distinct categories. Subsequently, the pseudo-labeled samples are purified, while multiple positive-negative discriminators are trained on particular data from the source domain to accurately identify the target domain samples containing the pseudo-labels. Finally, the backbone network is fine-tuned by the purified pseudo-labeled samples. Results of experiments on the self-built bearing dataset and the Paderborn University bearing dataset validate that the method proposed in this article exhibits outstanding performance.
更多查看译文
关键词
pseudo-label,fault diagnosis,transfer learning,iterative purification,graph neural network
AI 理解论文
溯源树
样例
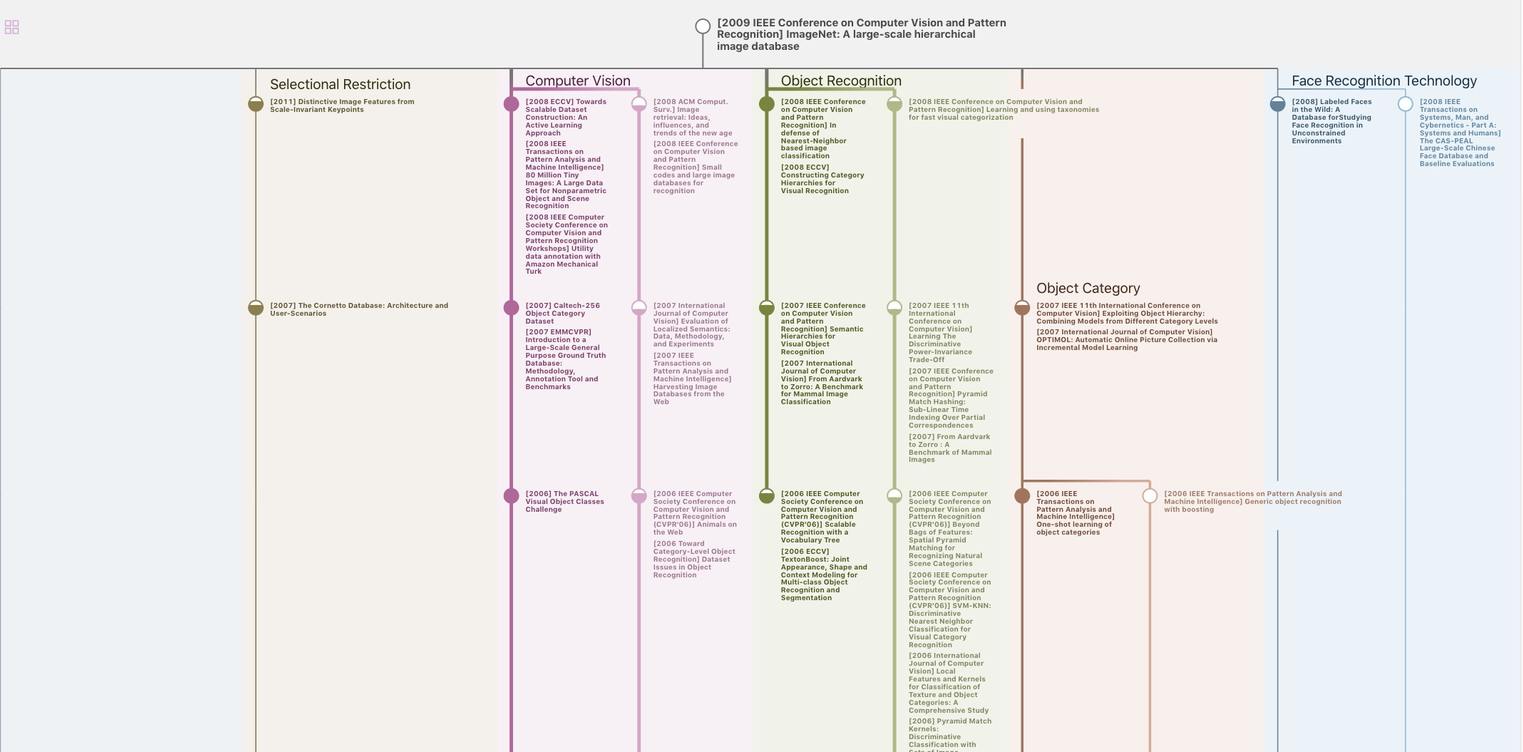
生成溯源树,研究论文发展脉络
Chat Paper
正在生成论文摘要