A novel spatio-temporal memory network for video anomaly detection
Multimedia Tools and Applications(2024)
摘要
Future frame prediction for anomaly detection methods based on memory networks have been extensively explored in the academic domain. Nevertheless, traditional memory-guided network techniques, which store dispersed spatial low-dimensional features, often fall short in delivering satisfactory results when applied to datasets characterized by variable scenes. This deficiency is evident in the frequent challenges faced during network convergence in the training process, resulting in unstable training outcomes. In response to this challenge, we introduce a novel Spatio-Temporal Memory Module, denoted as ST_MemAE. Our approach is designed to retain temporal correlation information within low-dimensional features, enhancing the representation of temporally closely linked features within the output of the encoder. Furthermore, we incorporate a homogeneous uncertainty function to optimize the balance of weights associated with multiple loss functions that are part of the memory module update process. As a result, our method offers improved stability in model training, faster convergence, and higher quality predictions of future frames. To validate the effectiveness of our approach, we conducted extensive experiments utilizing three distinct video anomaly detection datasets: UCSD Pedestrian 2, CUHK Avenue, and ShanghaiTech. The outcomes of these comprehensive experiments on publicly available datasets underscore the robustness of our method in accommodating diverse normal events while maintaining sensitivity to abnormal events.
更多查看译文
关键词
Video anomaly detection,Auto-encoder,Feature extraction,Memory
AI 理解论文
溯源树
样例
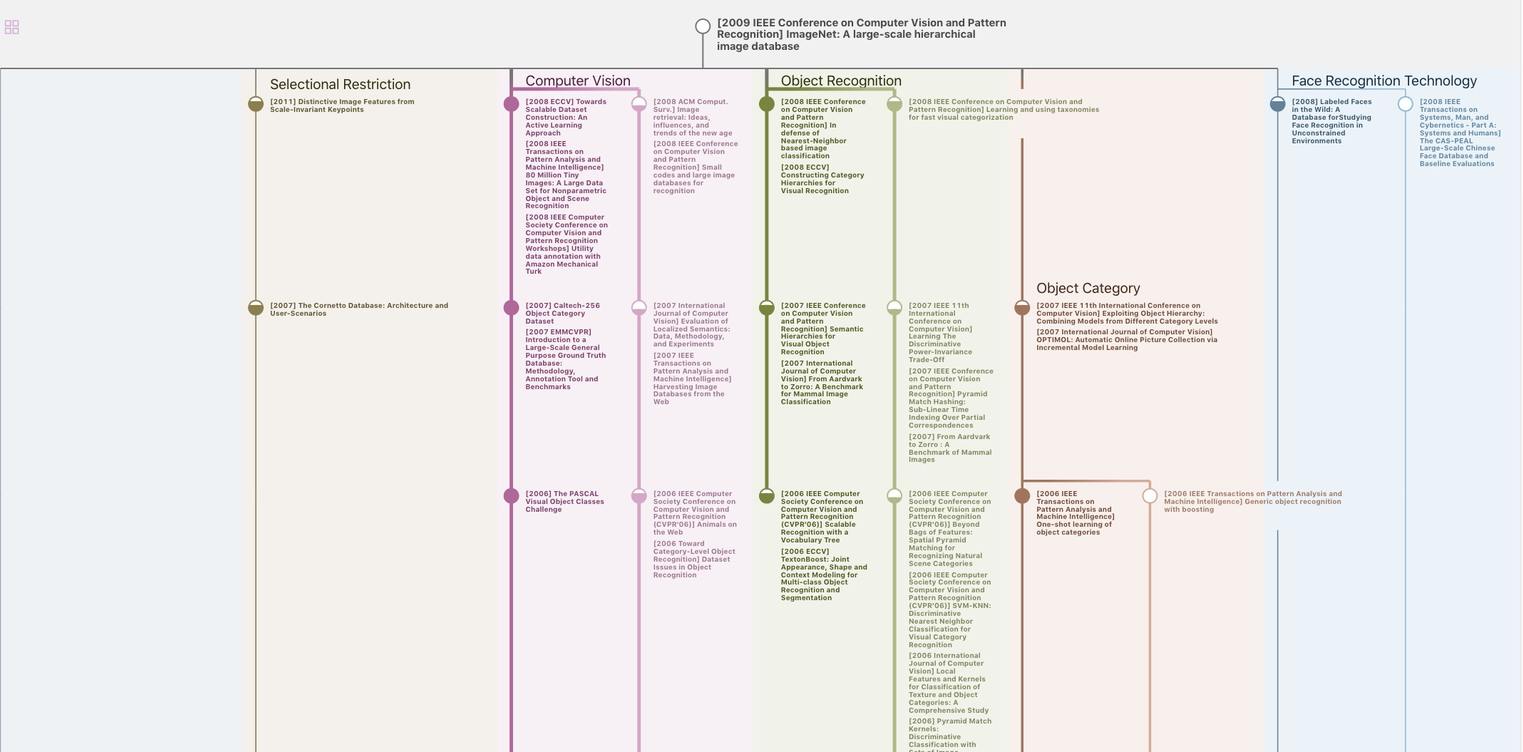
生成溯源树,研究论文发展脉络
Chat Paper
正在生成论文摘要