Unleashing the Power of Machine Learning and Remote Sensing for Robust Seasonal Drought Monitoring: A Stacking Ensemble Approach
Journal of Hydrology(2024)
摘要
Droughts cause significant economic losses in many regions around the world, highlighting a need to more accurately quantify implications of drought on production and water management. Remote sensing technologies and machine learning-based models offer promising solutions for timely and accurate regional drought monitoring, but the accuracy of such approaches is constrained by both the conceptual design and algorithms underpinning such approaches. We developed a machine learning stacking ensemble approach to overcome such limitations, drawing upon Precipitation Estimation from Remotely Sensed Information using Artificial Neural Network-Climate Data Record (PERSIANN-CDR), Moderate Resolution Imaging Spectroradiometer (MODIS) remote sensing products, and climate zoning data to estimate 3-month scale SPEI (Standardized Precipitation Evapotranspiration Index, SPEI-3) in 9 sub-regions of China. We compared 19 individual machine learning models and used the stacking approach to select the most robust meta-model for SPEI-3 prediction. We found that CatBoost Regressor (CBR), Extra Trees Regressor (ETR), Extreme Gradient Boosting (XGB), Light Gradient Boosting Machine (LGBM), and Random Forest (RF) were the top individual models for predicting drought, while using CBR as the stacked meta-model achieved the best performance. The R2 values for the stacking model with CBR as the meta-model were 0.9065 and 0.8218 in the eastern and western regions, respectively. We then employed the stacking model with CBR as the meta-model to generate seasonal drought maps based on SPEI-3 across different years and seasons. Our predicted SPEI-3 was compared with drought maps generated by the GPCC and ERA5 reanalysis datasets. We found a strong correlation between them with R2 exceeding 0.8 across multiple years and seasons, indicating our machine learning stacking ensemble approach had a good performance in monitoring seasonal drought conditions. The findings of our study establish a standardized protocol for predicting droughts in the agricultural sector, enabling its widespread application irrespective of variations in terrain and climate.
更多查看译文
关键词
Seasonal drought,Standardized Precipitation Evapotranspiration Index,Machine learning,Ensemble learning,Remote sensing
AI 理解论文
溯源树
样例
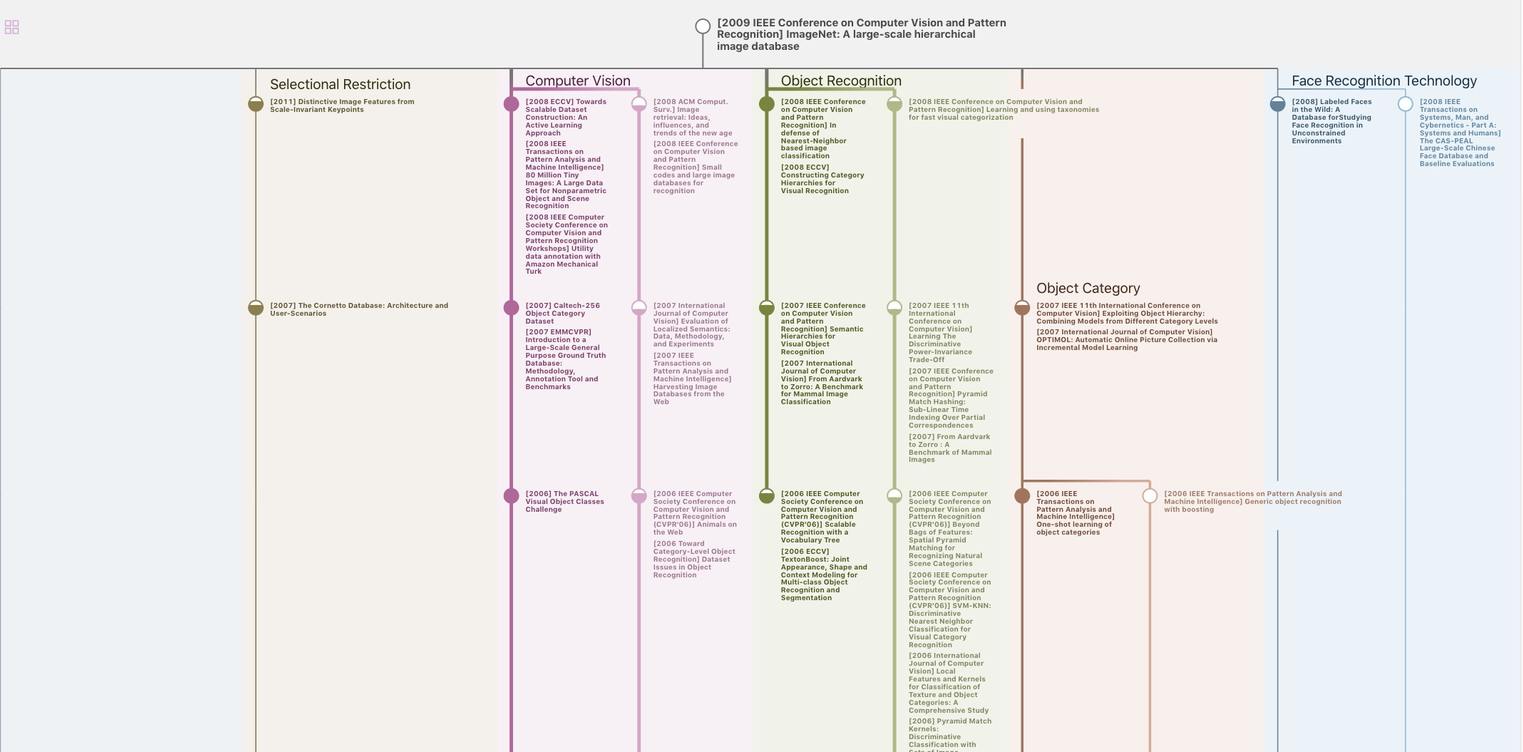
生成溯源树,研究论文发展脉络
Chat Paper
正在生成论文摘要