LAG-YOLO: Efficient road damage detector via lightweight attention ghost module
Journal of Intelligent Construction(2024)
摘要
Road damage detection plays an important role in ensuring road safety and improving traffic flow. The dramatic progress of artificial intelligence (AI) technology offers new opportunities for this field. In this paper, we introduce lightweight attention ghost-you only look once (LAG-YOLO), an efficient deep-learning network for road damage detection. LAG-YOLO optimizes the network structure of YOLO, making it more suitable for real-time processing and lightweight deployment while ensuring high accuracy. In addition, a novel module called attention ghost is designed to reduce the model parameters and improve the model performance by the simple attention module (SimAM). LAG-YOLO achieves an impressive parameter reduction to 4.19 million, delivering remarkable mean average precision (mAP) scores of 45.80% on the Hualu dataset and 52.35% on the RDD2020 dataset. In summary, the proposed network performs satisfactorily on extensive road damage datasets with fewer parameters, making it more suitable to be deployed in practice.
更多查看译文
关键词
road damage,deep learning,lightweight detector,attention mechanism
AI 理解论文
溯源树
样例
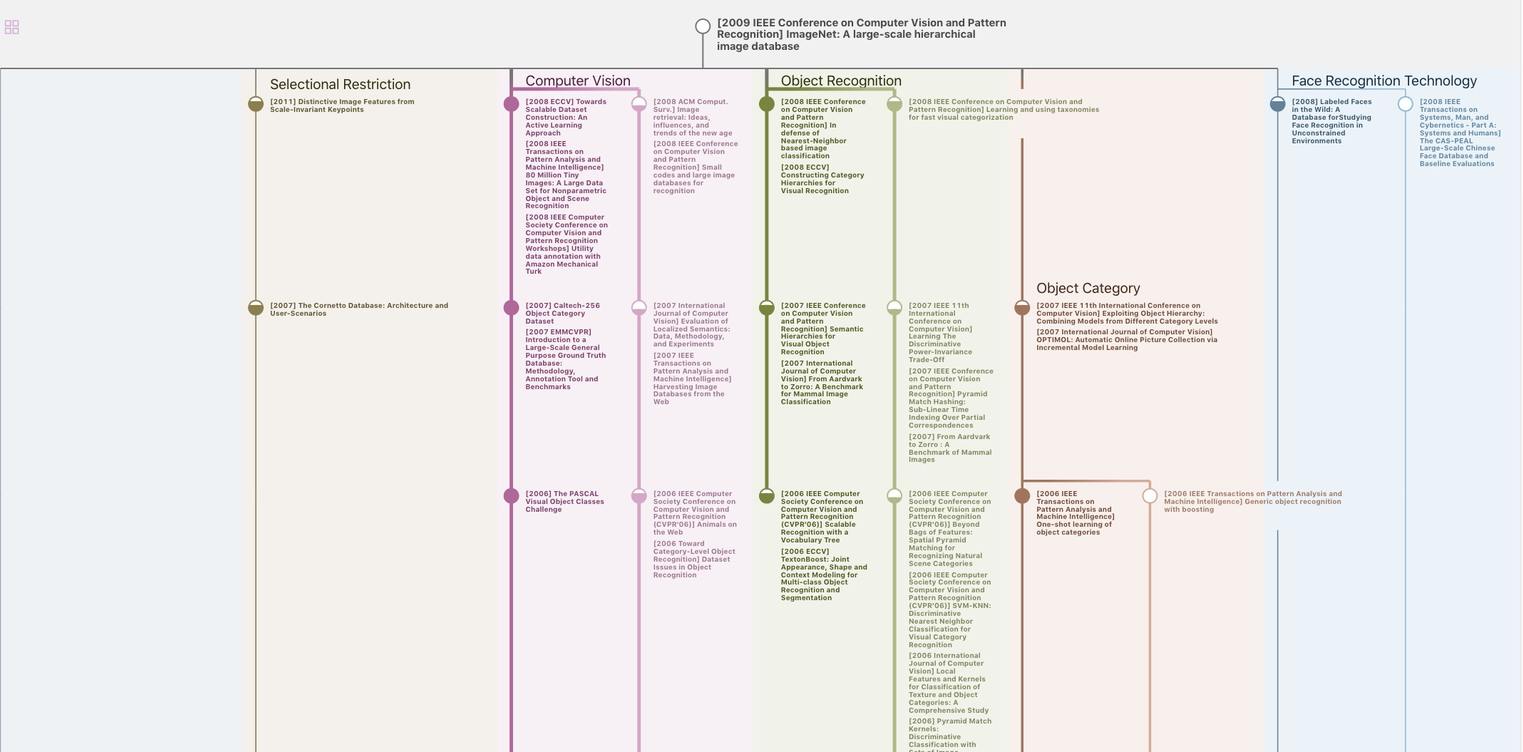
生成溯源树,研究论文发展脉络
Chat Paper
正在生成论文摘要